RecSys-DAN: Discriminative Adversarial Networks for Cross-Domain Recommender Systems
IEEE Transactions on Neural Networks and Learning Systems(2020)
摘要
Data sparsity and data imbalance are practical and challenging issues in cross-domain recommender systems (RSs). This paper addresses those problems by leveraging the concepts which derive from representation learning, adversarial learning, and transfer learning (particularly, domain adaptation). Although various transfer learning methods have shown promising performance in this context, our proposed novel method RecSys-DAN focuses on alleviating the cross-domain and within-domain data sparsity and data imbalance and learns transferable latent representations for users, items, and their interactions. Different from the existing approaches, the proposed method transfers the latent representations from a source domain to a target domain in an adversarial way. The mapping functions in the target domain are learned by playing a min-max game with an adversarial loss, aiming to generate domain indistinguishable representations for a discriminator. Four neural architectural instances of ResSys-DAN are proposed and explored. Empirical results on real-world Amazon data show that, even without using labeled data (i.e., ratings) in the target domain, RecSys-DAN achieves competitive performance as compared to the state-of-the-art supervised methods. More importantly, RecSys-DAN is highly flexible to both unimodal and multimodal scenarios, and thus it is more robust to the cold-start recommendation which is difficult for the previous methods.
更多查看译文
关键词
Adversarial learning,domain adaptation,imbalanced data,neural networks,recommender systems (RSs)
AI 理解论文
溯源树
样例
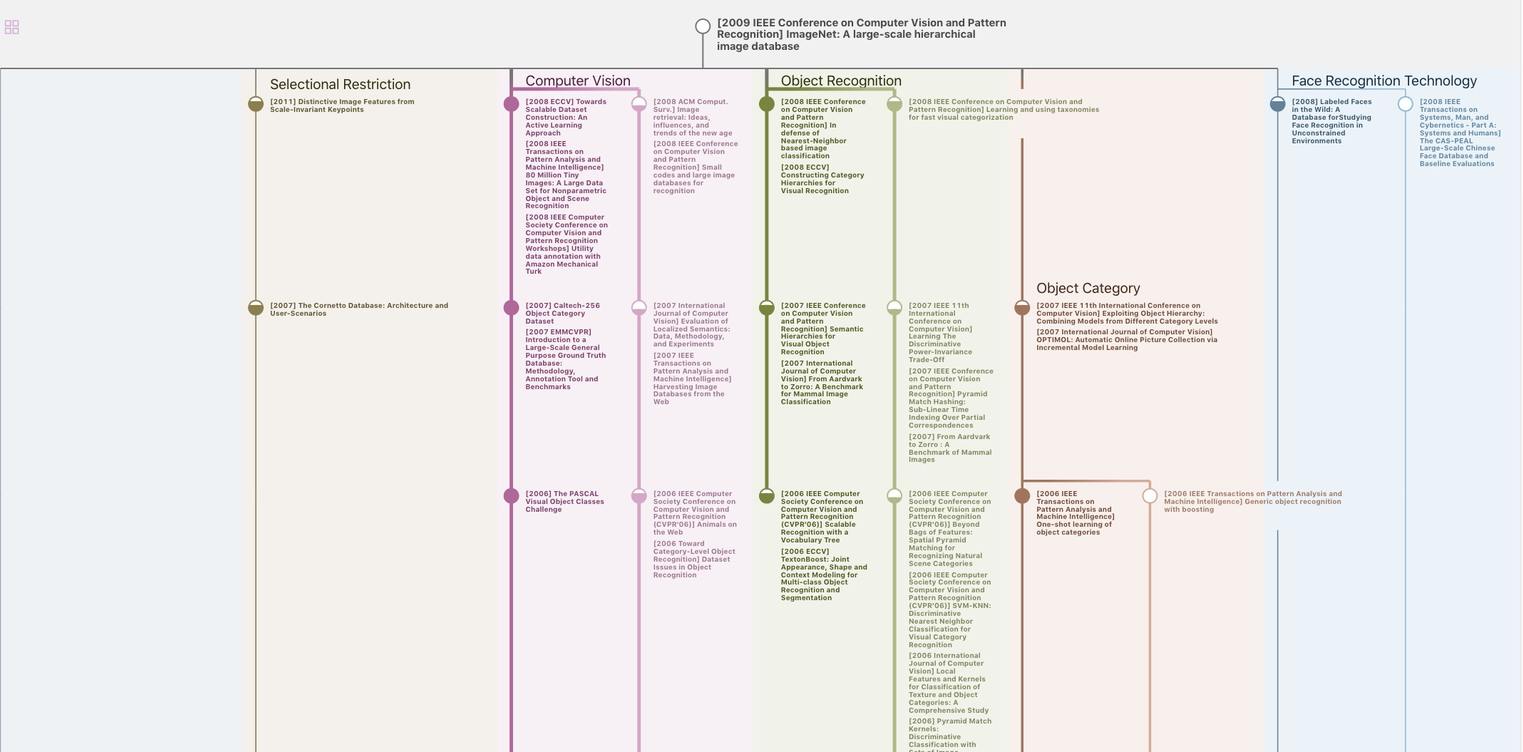
生成溯源树,研究论文发展脉络
Chat Paper
正在生成论文摘要