Fast active learning for hyperspectral image classification using extreme learning machine.
IET Image Processing(2019)
摘要
Owing to undulating and complexity of the earth's surface, obtaining the training samples for remote sensing data is time-consuming and expensive. Therefore, it is highly desirable to design a model that uses as few labelled samples as possible and reducing the computational time. Several active learning (AL) algorithms have been proposed in the literature for the classification of hyperspectral images (HSIs). However, its performance in terms of computational time has not been focused yet. Here, the authors have proposed AL approach based on extreme learning machine (ELM) that effectively decreases the computational time while maintaining the classification accuracy. Further, the effectiveness of the proposed approach has been depicted by comparing its performance with state-of-the-art AL algorithms in terms of classification accuracy and computational time as well. The ELM-based AL with different query strategies were conducted on two HSI data sets. The proposed approach achieves the classification accuracy up to 90% which is comparable to support vector machine-based AL approach but effectively reduces the computational time significantly by 1000 times. Thus, the proposed system shows the encouraging results with adequate classification accuracy while reducing the computation time drastically.
更多查看译文
关键词
geophysical image processing,image classification,learning (artificial intelligence),hyperspectral imaging,remote sensing
AI 理解论文
溯源树
样例
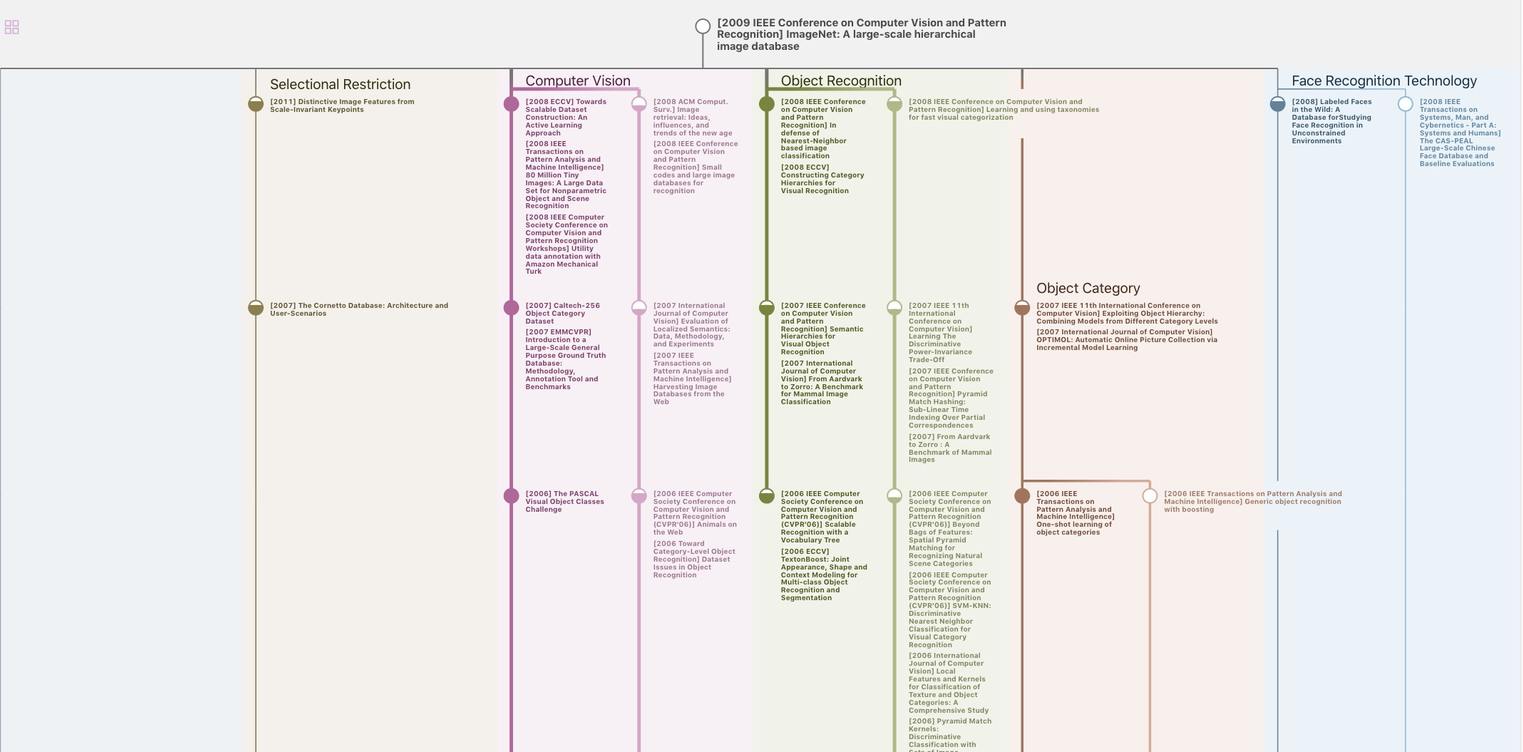
生成溯源树,研究论文发展脉络
Chat Paper
正在生成论文摘要