Sampling From Rough Energy Landscapes
COMMUNICATIONS IN MATHEMATICAL SCIENCES(2020)
摘要
We examine challenges to sampling from Boltzmann distributions associated with multiscale energy landscapes. The multiscale features, or "roughness", corresponds to highly oscillatory, but bounded, perturbations of a smooth landscape. Through a combination of numerical experiments and analysis we demonstrate that the performance of Metropolis adjusted Langevin algorithm can be severely attenuated as the roughness increases. In contrast, we prove that random walk Metropolis is insensitive to such roughness. We also formulate two alternative sampling strategies that incorporate large scale features of the energy landscape, while resisting the impact of fine scale roughness; these also outperform random walk Metropolis. Numerical experiments on these landscapes are presented that confirm our predictions. Open questions and numerical challenges are also highlighted.
更多查看译文
关键词
Markov chain Monte Carlo, random walk Metropolis, Metropolis adjusted Langevin, rough energy landscapes, multi-scale energy landscapes, mean squared displacement
AI 理解论文
溯源树
样例
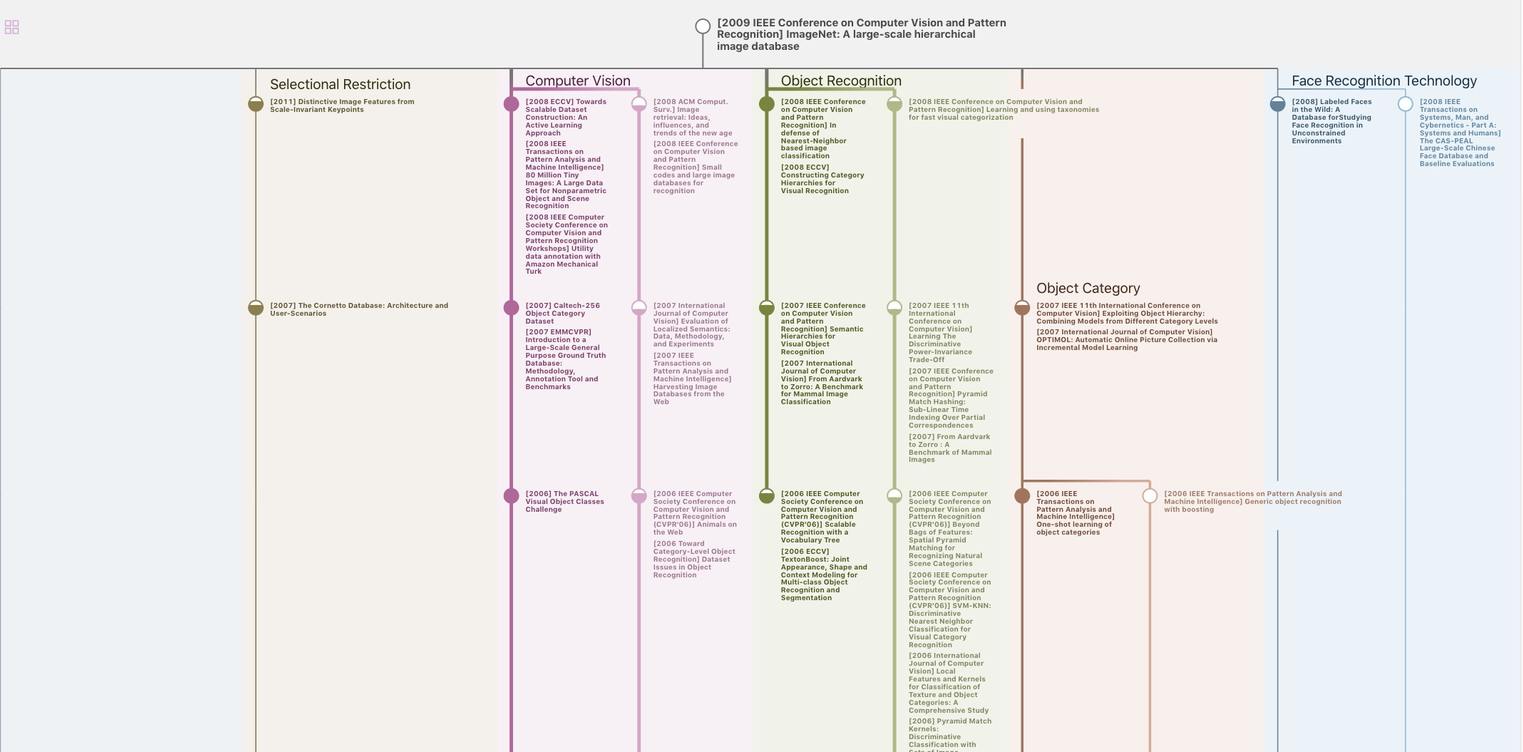
生成溯源树,研究论文发展脉络
Chat Paper
正在生成论文摘要