On Preempting Advanced Persistent Threats Using Probabilistic Graphical Models.
CoRR(2019)
摘要
This paper presents PULSAR, a framework for pre-empting Advanced Persistent Threats (APTs). PULSAR employs a probabilistic graphical model (specifically a Factor Graph) to infer the time evolution of an attack based on observed security events at runtime. PULSAR (i) learns the statistical significance of patterns of events from past attacks; (ii) composes these patterns into FGs to capture the progression of the attack; and (iii) decides on preemptive actions. PULSARu0027s accuracy and its performance are evaluated in three experiments at SystemX: (i) a study with a dataset containing 120 successful APTs over the past 10 years (PULSAR accurately identifies 91.7%); (ii) replaying of a set of ten unseen APTs (PULSAR stops 8 out of 10 replayed attacks before system integrity violation, and all ten before data exfiltration); and (iii) a production deployment of PULSAR (during a month-long deployment, PULSAR took an average of one second to make a decision).
更多查看译文
AI 理解论文
溯源树
样例
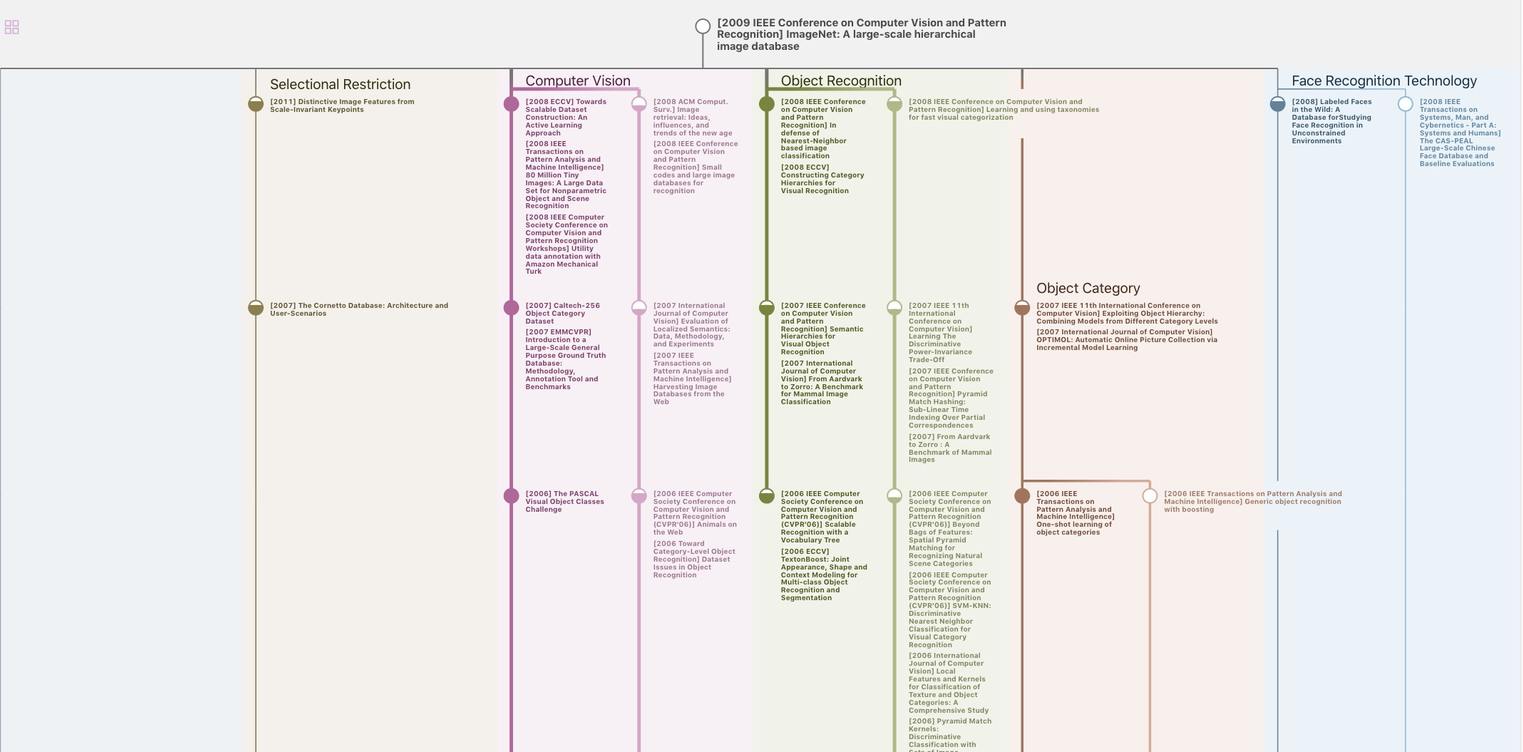
生成溯源树,研究论文发展脉络
Chat Paper
正在生成论文摘要