Evidence that self-similar microrheology of highly entangled polymeric solutions scales robustly with, and is tunable by, polymer concentration
arXiv: Soft Condensed Matter(2018)
Abstract
We report observations of a remarkable scaling behavior with respect to concentration in the passive microbead rheology of two highly entangled polymeric solutions, polyethylene oxide (PEO) and hyaluronic acid (HA). This behavior was reported previously [Hill et al., PLOS ONE (2014)] for human lung mucus, a complex biological hydrogel, motivating the current study for synthetic polymeric solutions PEO and HA. The strategy is to identify, and focus within, a wide range of lag times τ for which passive micron diameter beads exhibit self-similar (fractional, power law) mean-squared-displacement (MSD) statistics. For lung mucus, PEO at three different molecular weights (Mw), and HA at one Mw, we find ensemble-averaged MSDs of the form ⟨Δr^2(τ)⟩ = 4D_ατ^α, all within a common band, [1/60 sec, 3 sec], of lag times τ. We employ the MSD power law parameters (D_α,α) to classify each polymeric solution over a range of highly entangled concentrations. By the generalized Stokes-Einstein relation, power law MSD implies power law elastic G'(ω) and viscous G”(ω) moduli for frequencies 1/τ, [0.33 sec^-1, 60 sec^-1]. A natural question surrounds the polymeric properties that dictate D_α and α, e.g. polymer concentration c, Mw, and stiffness (persistence length). In [Hill et al., PLOS ONE (2014)], we showed the MSD exponent α varies linearly, while the pre-factor D_α varies exponentially, with concentration, i.e. the semi-log plot, (log(D_α),α)(c) of the classifier data is collinear. Here we show the same result for three distinct Mw PEO and HA at a single Mw. Future studies are required to explore the generality of these results for polymeric solutions, and to understand this scaling behavior with polymer concentration.
MoreTranslated text
AI Read Science
Must-Reading Tree
Example
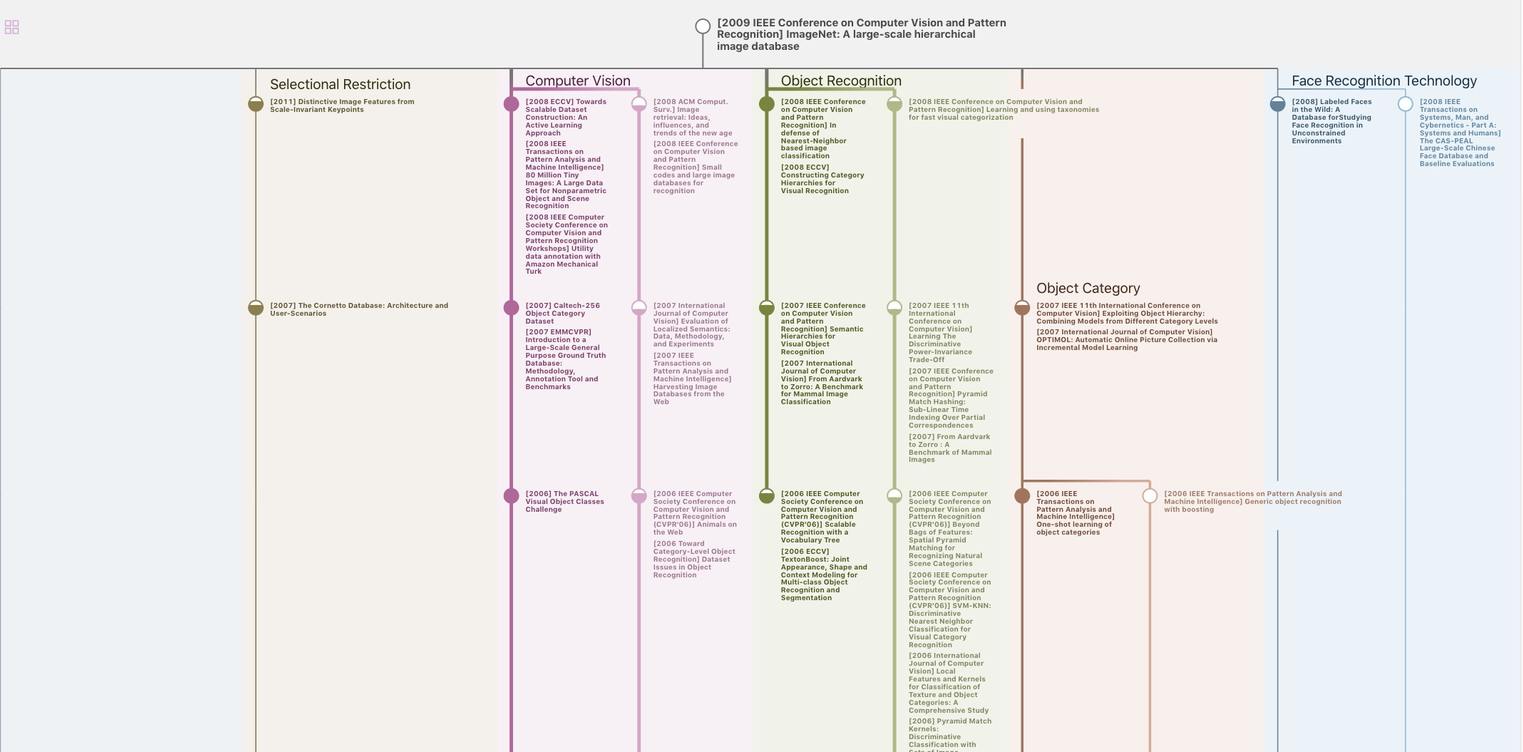
Generate MRT to find the research sequence of this paper
Chat Paper
Summary is being generated by the instructions you defined