Structured Domain Randomization: Bridging the Reality Gap by Context-Aware Synthetic Data
2019 INTERNATIONAL CONFERENCE ON ROBOTICS AND AUTOMATION (ICRA)(2018)
摘要
We present structured domain randomization (SDR), a variant of domain randomization (DR) that takes into account the structure and context of the scene. In contrast to DR, which places objects and distractors randomly according to a uniform probability distribution, SDR places objects and distractors randomly according to probability distributions that arise from the specific problem at hand. In this manner, SDR-generated imagery enables the neural network to take the context around an object into consideration during detection. We demonstrate the power of SDR for the problem of 2D bounding box car detection, achieving competitive results on real data after training only on synthetic data. On the KITTI easy, moderate, and hard tasks, we show that SDR outperforms other approaches to generating synthetic data (VKITTI, Sim 200k, or DR), as well as real data collected in a different domain (BDD100K). Moreover, synthetic SDR data combined with real KITTI data outperforms real KITTI data alone.
更多查看译文
关键词
synthetic SDR data,structured domain randomization,context-aware synthetic data,uniform probability distribution,SDR places objects,probability distributions,SDR-generated imagery,2D bounding box car detection
AI 理解论文
溯源树
样例
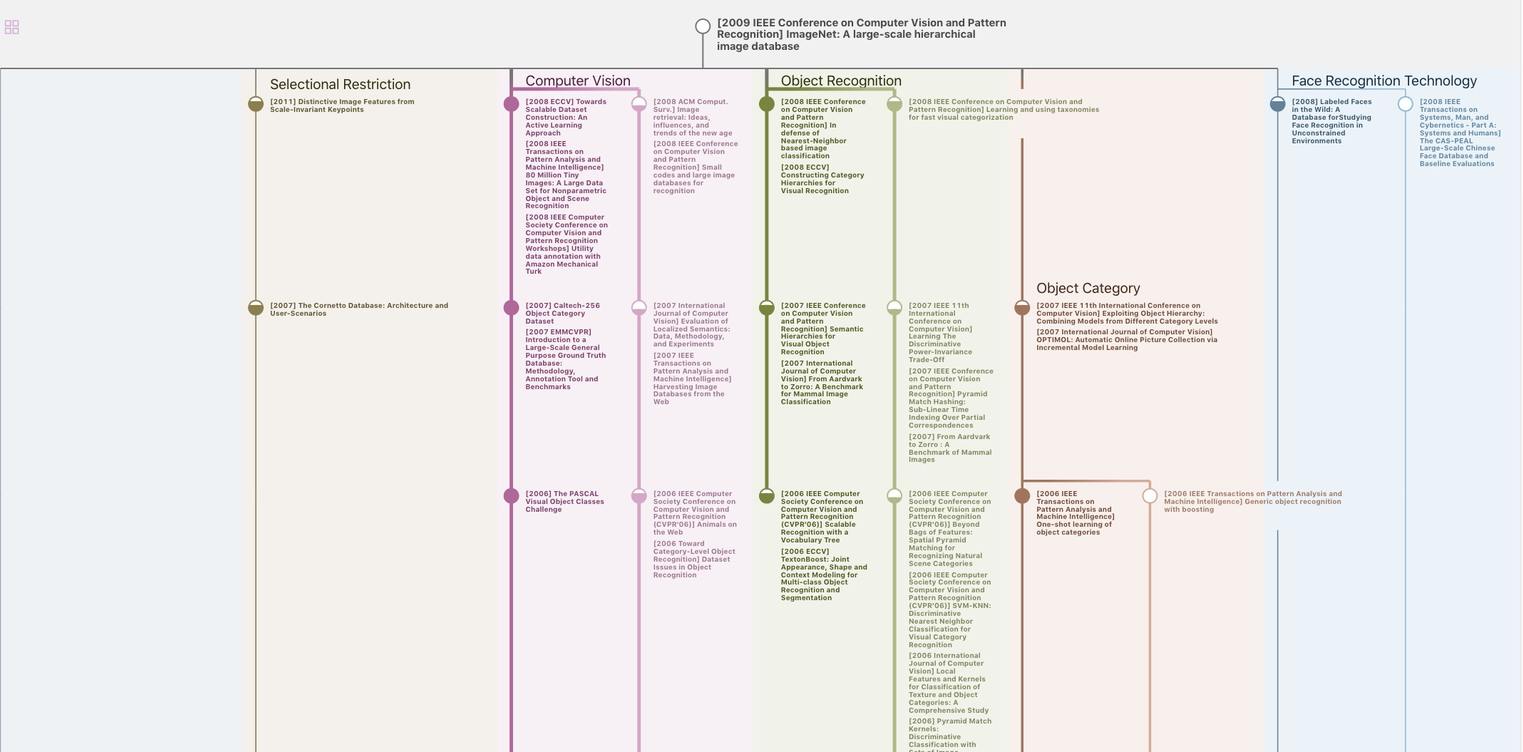
生成溯源树,研究论文发展脉络
Chat Paper
正在生成论文摘要