The Node-Similarity Distribution of Complex Networks and Its Applications in Link Prediction
IEEE TRANSACTIONS ON KNOWLEDGE AND DATA ENGINEERING(2022)
摘要
Over the years, quantifying the similarity of nodes has been a hot topic in network science, yet little has been known about the distribution of node-similarity. In this paper, we consider a typical measure of node-similarity called the common neighbor based similarity (CNS). By means of the generating function, we propose a general framework for calculating the CNS distributions of node sets in various networks. Particularly, we show that for the Erdos-Renyi random network, the CNS distribution of node sets of any size obeys the Poisson law. Furthermore, we connect the node-similarity distribution to the link prediction problem, and derive analytical solutions for two key evaluation metrics: i) precision and ii) area under the receiver operating characteristic curve (AUC). We also use the similarity distributions to optimize link prediction by i) deriving the expected prediction accuracy of similarity scores and ii) providing the optimal prediction priority of unconnected node pairs. Simulation results confirm our theoretical findings and also validate the proposed tools in evaluating and optimizing link prediction.
更多查看译文
关键词
Complex networks,Tools,Training,Data models,Predictive models,Measurement,Node-similarity,common neighbor,link prediction,complex networks
AI 理解论文
溯源树
样例
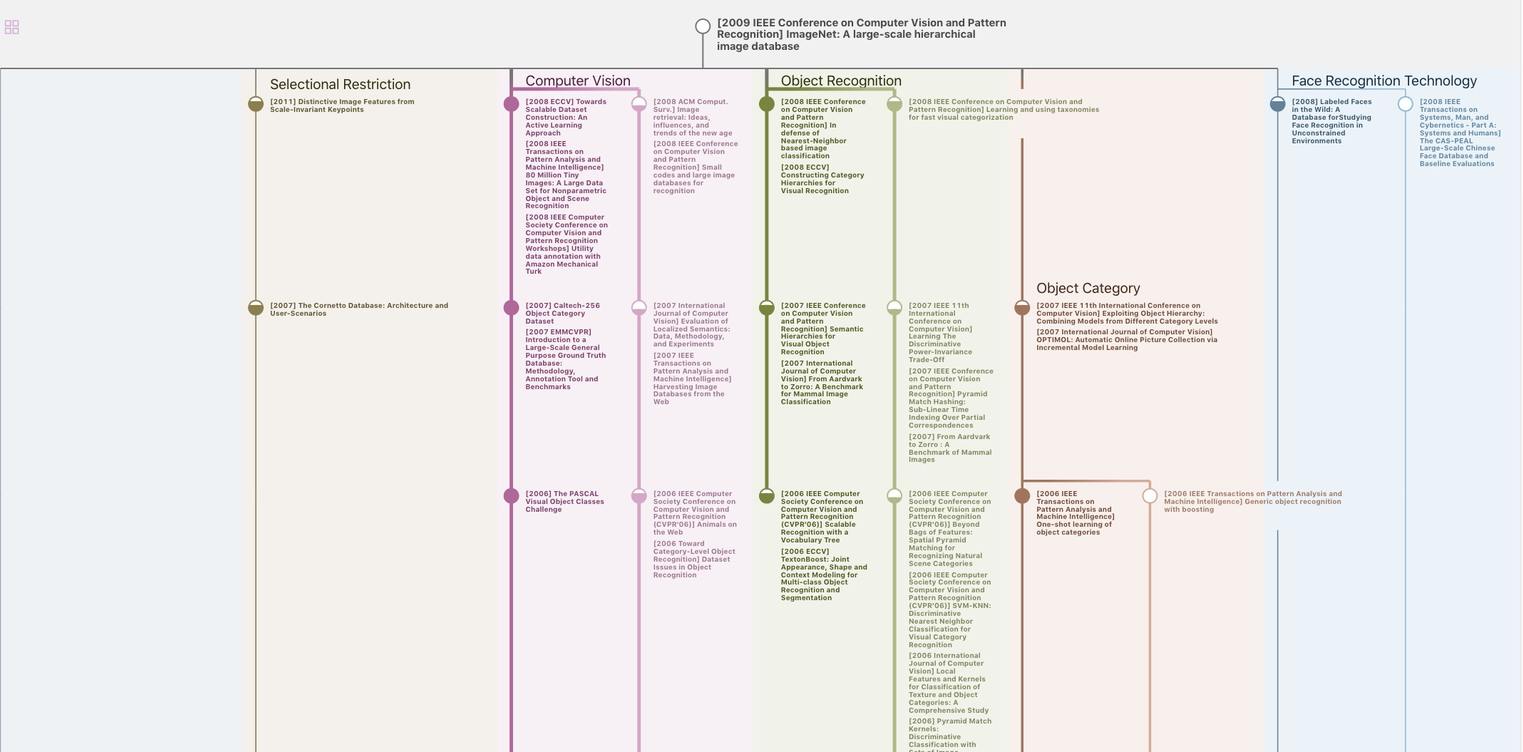
生成溯源树,研究论文发展脉络
Chat Paper
正在生成论文摘要