Incorporating Semantic Item Representations To Soften The Cold Start Problem
WEBMEDIA'18: PROCEEDINGS OF THE 24TH BRAZILIAN SYMPOSIUM ON MULTIMEDIA AND THE WEB(2018)
摘要
Recommender systems have been extensively used to provide meaningful and personalized content to users. A recurring issue, especially in collaborative filtering methods, is the cold-start problem, which can be related to new items or new users. This problem can be smoothed by aggregating item information into the recommender calculation, thus the semantics behind these items representations are important. In this paper, we propose four rich item representations, based on three kinds of semantics: sentiment analysis, sense embeddings and similarities. The items' features are disambiguated concepts extracted from textual users' reviews, which are known for possessing a great information load with both item descriptions and user preferences. We apply these four representations in two classic collaborative filtering algorithms, which were adapted to be attribute aware. We compare our approach against the original recommenders, and evaluate our results in two very different datasets to show the generality of our approach. Results show a very positive influence of the item representations to reduce prediction error.
更多查看译文
关键词
Recommender systems, Item representation, cold-start
AI 理解论文
溯源树
样例
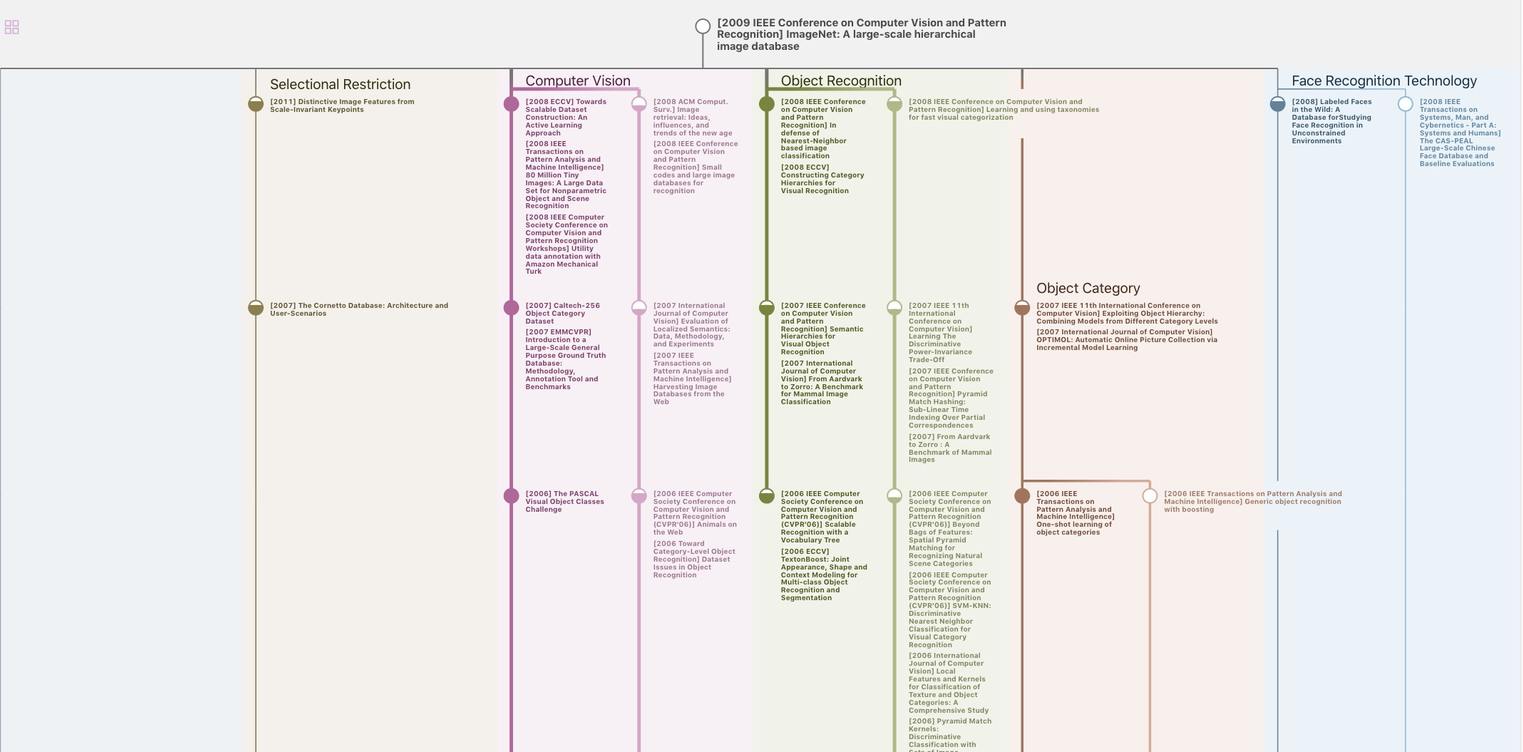
生成溯源树,研究论文发展脉络
Chat Paper
正在生成论文摘要