Powerful, transferable representations for molecules through intelligent task selection in deep multitask networks
PHYSICAL CHEMISTRY CHEMICAL PHYSICS(2020)
摘要
Chemical representations derived from deep learning are emerging as a powerful tool in areas such as drug discovery and materials innovation. Currently, this methodology has three major limitations - the cost of representation generation, risk of inherited bias, and the requirement for large amounts of data. We propose the use of multi-task learning in tandem with transfer learning to address these limitations directly. In order to avoid introducing unknown bias into multi-task learning through the task selection itself, we calculate task similarity through pairwise task affinity, and use this measure to programmatically select tasks. We test this methodology on several real-world data sets to demonstrate its potential for execution in complex and low-data environments. Finally, we utilise the task similarity to further probe the expressiveness of the learned representation through a comparison to a commonly used cheminformatics fingerprint, and show that the deep representation is able to capture more expressive task-based information.
更多查看译文
关键词
deep multitask networks,molecules,intelligent task selection,transferable representations
AI 理解论文
溯源树
样例
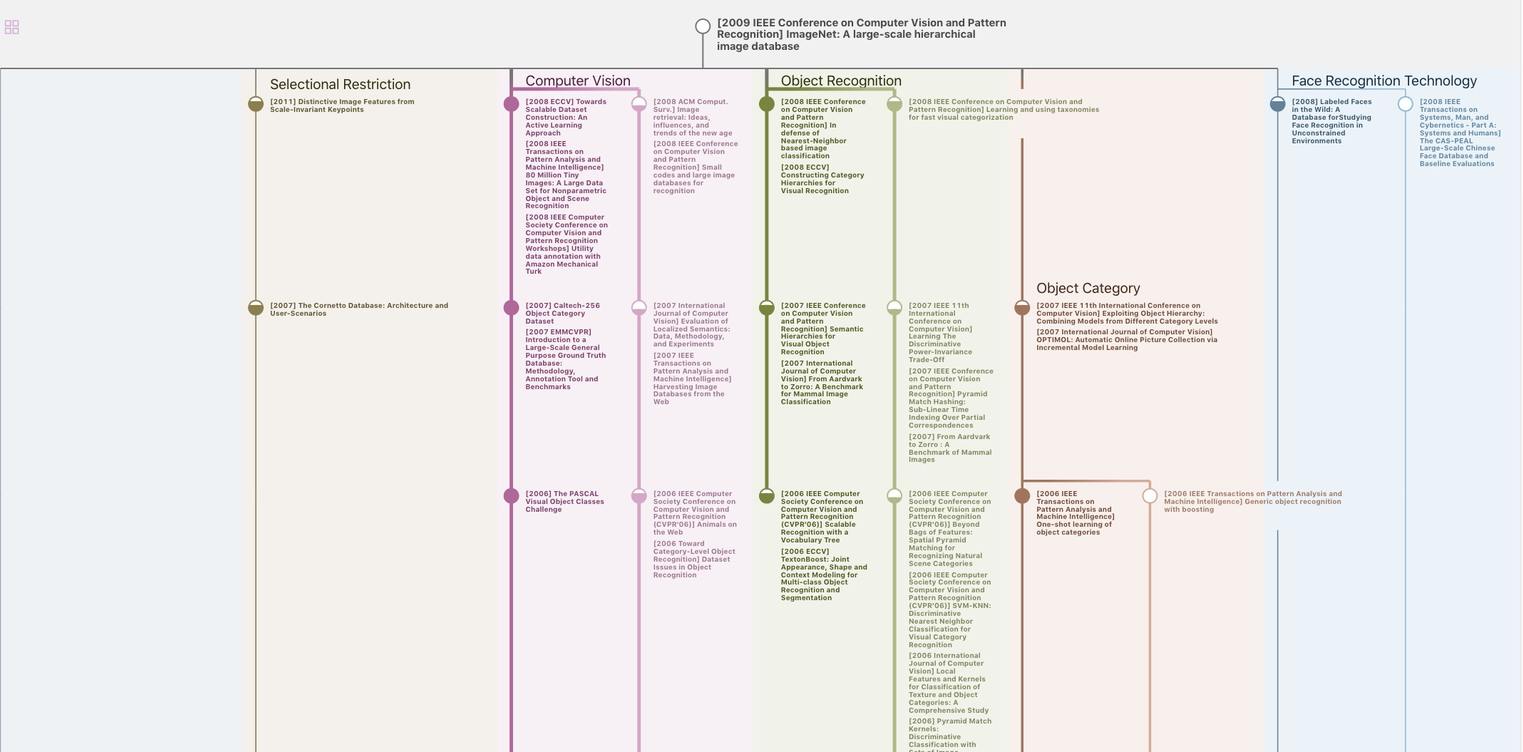
生成溯源树,研究论文发展脉络
Chat Paper
正在生成论文摘要