Efficient Weakly-Supervised Discrete Hashing For Large-Scale Social Image Retrieval
Pattern Recognition Letters(2020)
摘要
Hashing has been widely exploited for information retrieval recently, because of its high computation efficiency and low storage cost. However, many existing hashing methods cannot perform well on large-scale social image retrieval, due to the relaxed hash optimization and the lack of supervised semantic labels. In this paper, we propose an efficient Weakly-supervised Discrete Hashing (WDH) to solve the limitations. We formulate a unified weakly-supervised hash learning framework. It could effectively enrich the semantics of image hash codes with the freely obtained user-provided social tags and simultaneously remove their involved adverse noises. Furthermore, instead of relaxed hash optimization, we propose an efficient discrete hash optimization method based on Augmented Lagrangian Multiplier (ALM) to directly solve the hash codes without quantization information loss. Experiments on two standard social image datasets demonstrate the superior performance of the proposed method compared with several state-ofthe-art hashing techniques. (C) 2018 Elsevier B.V. All rights reserved.
更多查看译文
关键词
Hashing,Image retrieval
AI 理解论文
溯源树
样例
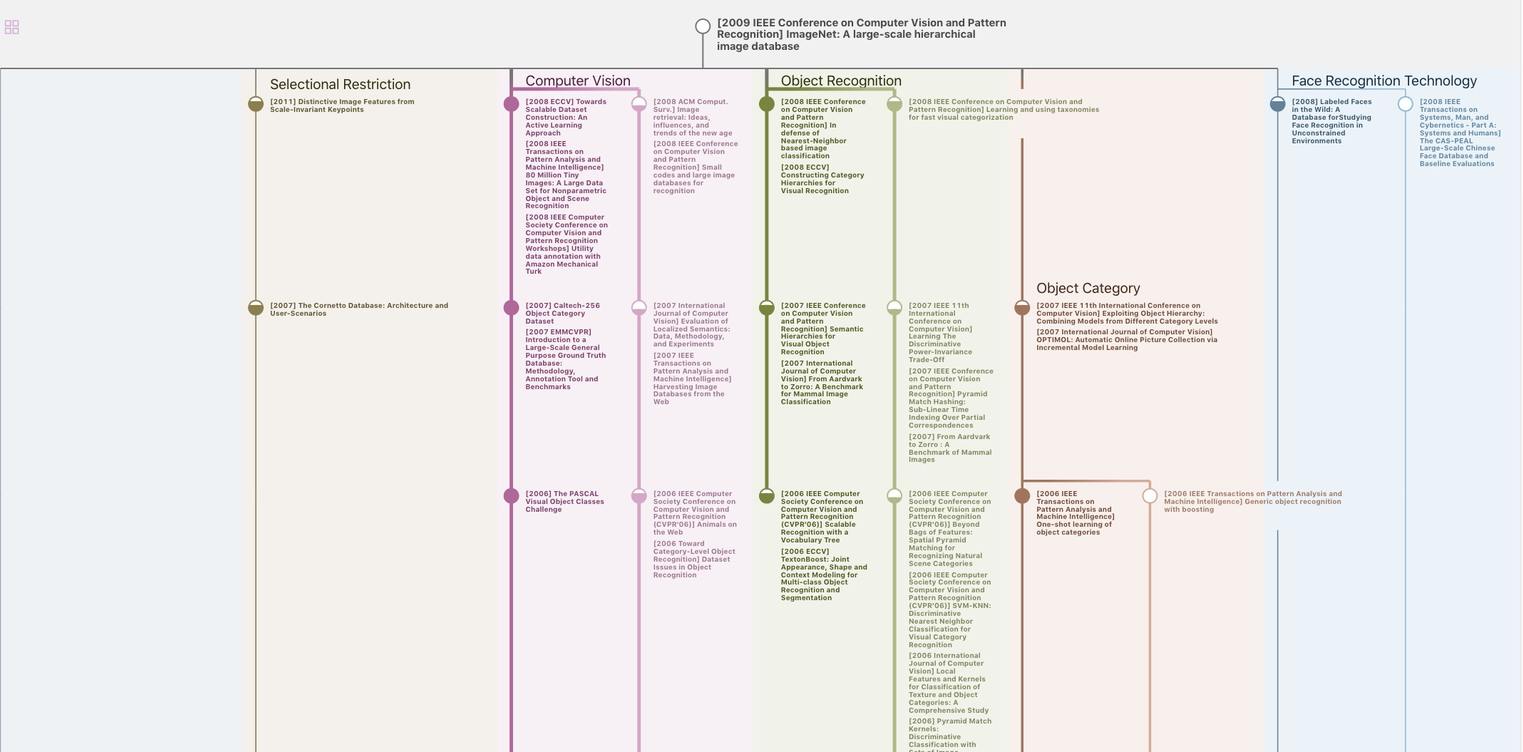
生成溯源树,研究论文发展脉络
Chat Paper
正在生成论文摘要