Network-Based Prediction of Polygenic Disease Genes Involved in Cell Motility
ACM-BCB'18: PROCEEDINGS OF THE 2018 ACM INTERNATIONAL CONFERENCE ON BIOINFORMATICS, COMPUTATIONAL BIOLOGY, AND HEALTH INFORMATICS(2018)
摘要
Schizophrenia and autism are examples of polygenic diseases caused by a multitude of genetic variants. Recently, both diseases have been associated with disrupted neuron motility and migration patterns, suggesting that aberrant cell motility is a phenotype for these neurological diseases. Abnormal neuronal development is central to both schizophrenia and autism, which critically implicates these cell motility perturbations in the disease mechanisms. However, despite the genetic characterization of these diseases by large-scale genome-wide association studies, extracting causality for symptoms and pathophysiology from these data remains challenging due to the large number of genes implicated and the additive effect the mutations have on the cellular processes. We present a network-based machine learning approach to identify genes implicated in both a disease of interest (e.g., schizophrenia or autism) and a disease phenotype (e.g., aberrant cell motility). We use a brain-specific functional interaction network to identify which genes are most centrally implicated in a polygenic disease based on functional similarity. Our algorithm identifies genes that are near known disease genes and cell motility genes in the network. Top schizophrenia candidates include many Protein Phosphatase 1 subunits and Lysyl Oxidase, which are promising genes for follow-up experimental validation. Candidate genes predicted by our method suggest testable hypotheses about these genes' role in cell motility regulation, offering a framework for generating predictions for experimental validation.
更多查看译文
关键词
Semi-supervised learning, functional interaction network, schizophrenia, autism, cell motility
AI 理解论文
溯源树
样例
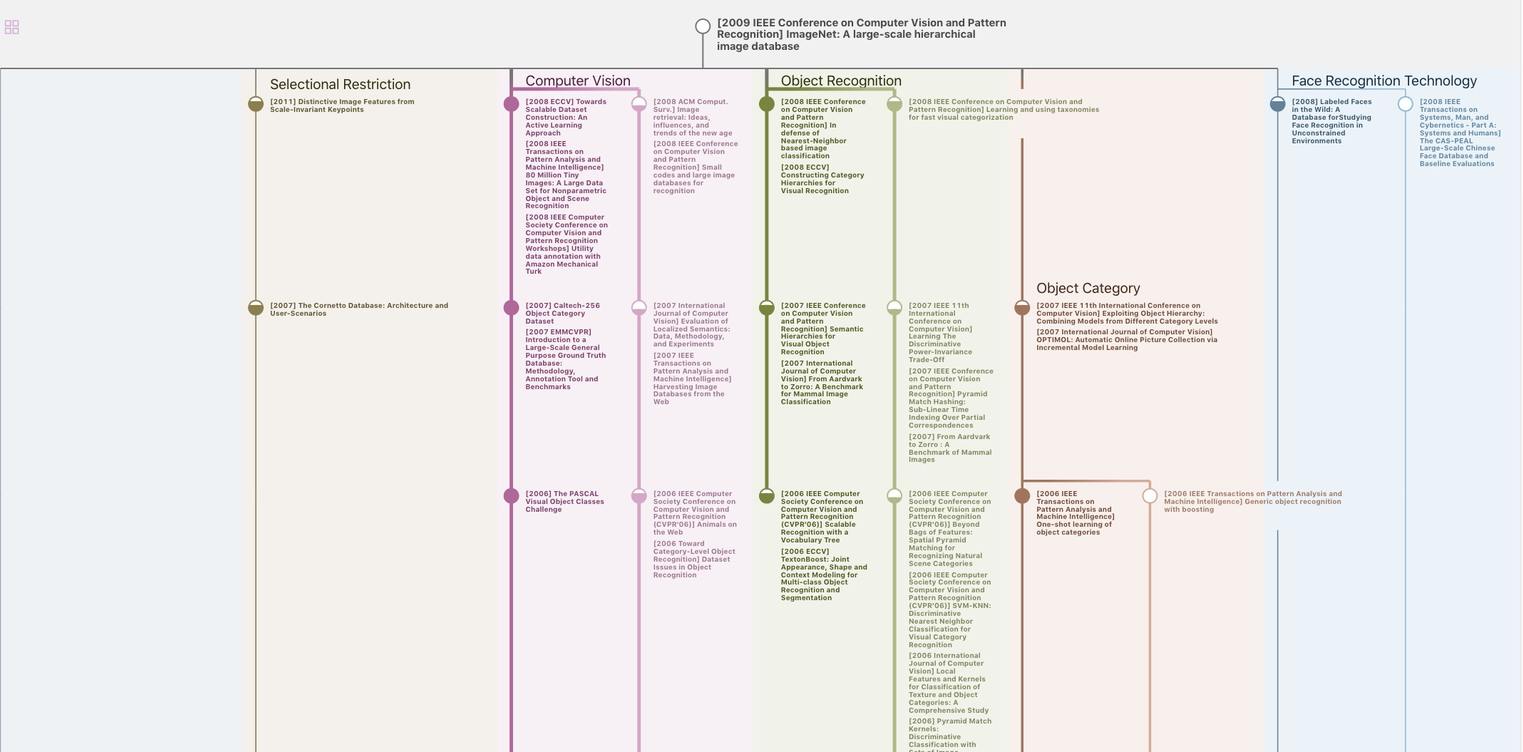
生成溯源树,研究论文发展脉络
Chat Paper
正在生成论文摘要