Adversarial Attacks on Deep-Learning Based Radio Signal Classification.
IEEE Wireless Communications Letters(2019)
摘要
Deep learning (DL), despite its enormous success in many computer vision and language processing applications, is exceedingly vulnerable to adversarial attacks. We consider the use of DL for radio signal (modulation) classification tasks, and present practical methods for the crafting of white-box and universal black-box adversarial attacks in that application. We show that these attacks can considerably reduce the classification performance, with extremely small perturbations of the input. In particular, these attacks are significantly more powerful than classical jamming attacks, which raises significant security and robustness concerns in the use of DL-based algorithms for the wireless physical layer.
更多查看译文
关键词
Perturbation methods,Modulation,Wireless communication,Signal to noise ratio,Computational modeling,Computer vision,Robustness
AI 理解论文
溯源树
样例
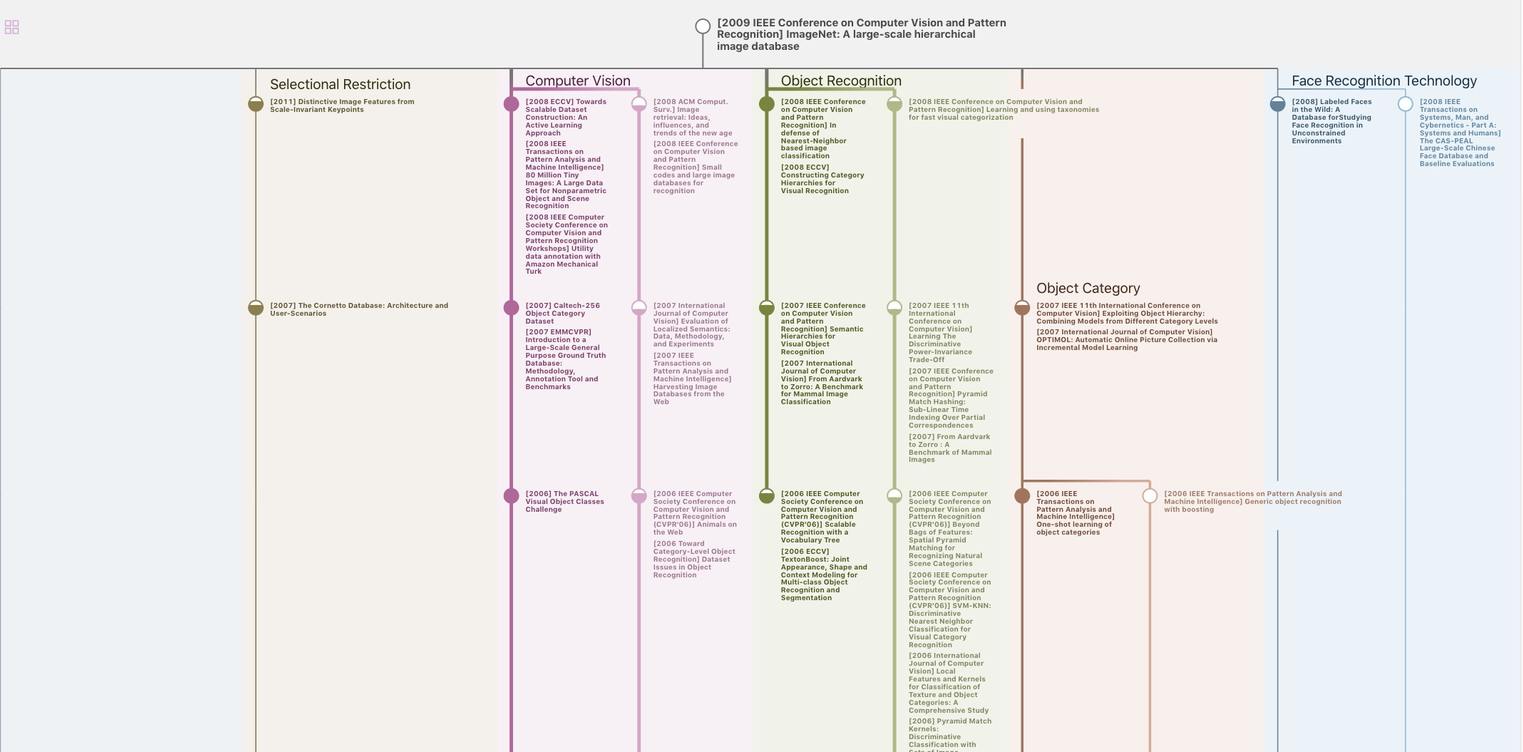
生成溯源树,研究论文发展脉络
Chat Paper
正在生成论文摘要