Inference Without Compatibility
arxiv(2019)
摘要
We consider hypothesis testing problems for a single covariate in the context of a linear model with Gaussian design when $p>n$. Under minimal sparsity conditions of their type and without any compatibility condition, we construct an asymptotically Gaussian estimator with variance equal to the oracle least-squares. The estimator is based on a weighted average of all models of a given sparsity level in the spirit of exponential weighting. We adapt this procedure to estimate the signal strength and provide a few applications. We support our results using numerical simulations based on algorithm which approximates the theoretical estimator and provide a comparison with the de-biased lasso.
更多查看译文
AI 理解论文
溯源树
样例
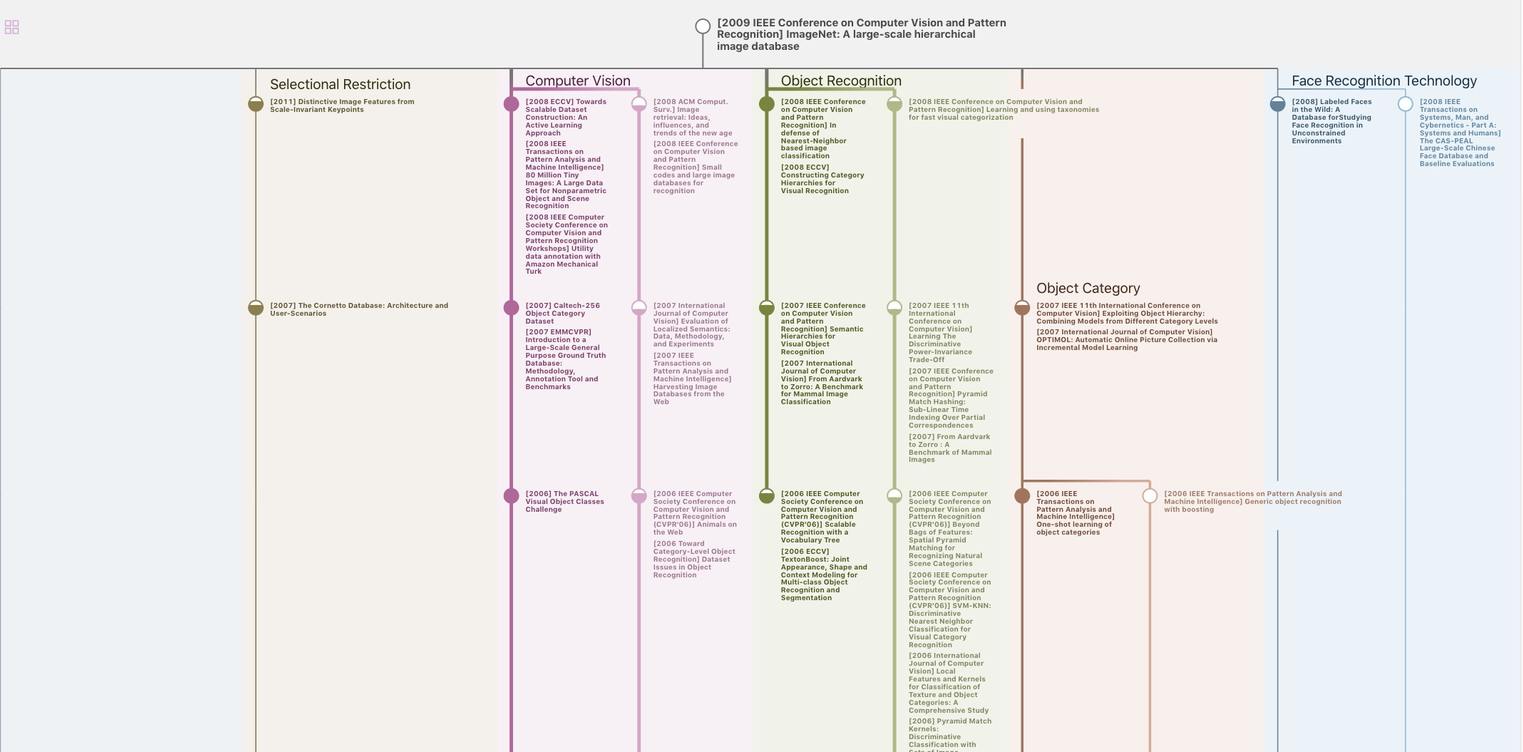
生成溯源树,研究论文发展脉络
Chat Paper
正在生成论文摘要