A Comprehensive Study on Predicting Functional Role of Metagenomes Using Machine Learning Methods.
IEEE/ACM transactions on computational biology and bioinformatics(2019)
摘要
"Metagenomics" is the study of genomic sequences obtained directly from environmental microbial communities with the aim to linking their structures with functional roles. The field has been aided in the unprecedented advancement through high-throughput omics data sequencing. The outcome of sequencing are biologically rich data sets. Metagenomic data consisting of microbial spe-cies which outnumber microbial samples, lead to the "curse of dimensionality". Hence the focus in metagenomics studies has moved towards developing efficient computational models using Machine Learning (ML), reducing the computational cost. In this paper, we comprehensively assessed various ML approaches to classifying high-dimensional human microbiota effectively into their functional phenotypes. We propose the application of embedded feature selection methods, namely, Extreme Gradient Boost-ing and Penalized Logistic Regression to determine important species. The resultant feature set enhanced the performance of one of the most popular state-of-the-art methods, Random Forest (RF) over metagenomic studies. Experimental results indicate that the proposed method achieved best results in terms of accuracy, area under Receiver Operating Characteristic curve (ROC-AUC) and major improvement in processing time. It outperformed other feature selection methods of filters or wrappers over RF and classifiers such as Support Vector Machine (SVM), Extreme Learning Machine (ELM), and -Nearest Neighbors (-NN).
更多查看译文
关键词
Sequential analysis,Radio frequency,Support vector machines,Diseases,Bioinformatics,Feature extraction,Boosting
AI 理解论文
溯源树
样例
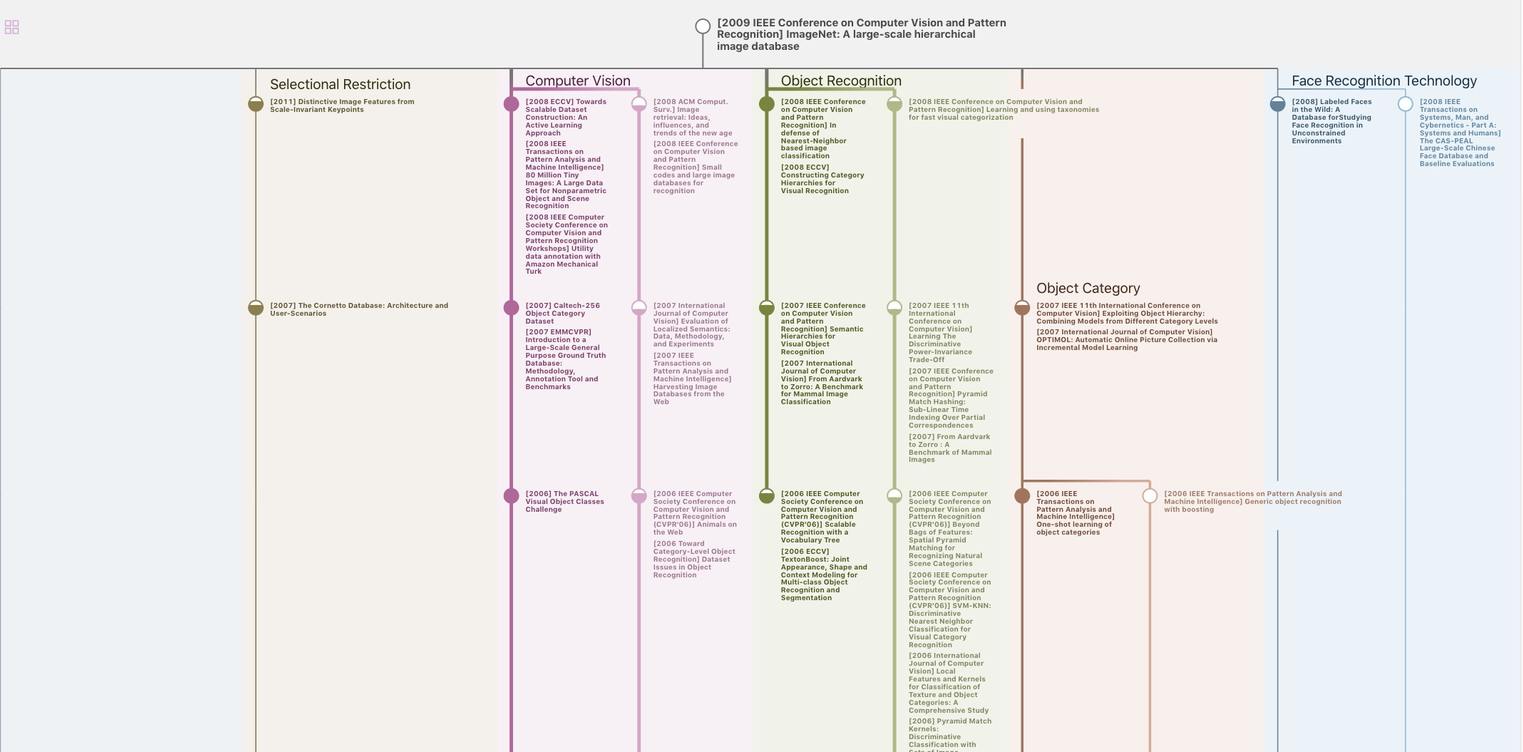
生成溯源树,研究论文发展脉络
Chat Paper
正在生成论文摘要