Flexible and accurate inference and learning for deep generative models.
ADVANCES IN NEURAL INFORMATION PROCESSING SYSTEMS 31 (NIPS 2018)(2018)
摘要
We introduce a new approach to learning in hierarchical latent-variable generative models called the "distributed distributional code Helmholtz machine", which emphasises flexibility and accuracy in the inferential process. Like the original Helmholtz machine and later variational autoencoder algorithms (but unlike adversarial methods) our approach learns an explicit inference or "recognition" model to approximate the posterior distribution over the latent variables. Unlike these earlier methods, it employs a posterior representation that is not limited to a narrow tractable parametrised form (nor is it represented by samples). To train the generative and recognition models we develop an extended wake-sleep algorithm inspired by the original Helmholtz machine. This makes it possible to learn hierarchical latent models with both discrete and continuous variables, where an accurate posterior representation is essential. We demonstrate that the new algorithm outperforms current state-of-the-art methods on synthetic, natural image patch and the MNIST data sets.
更多查看译文
关键词
latent variables,posterior distribution,continuous variables,helmholtz machine
AI 理解论文
溯源树
样例
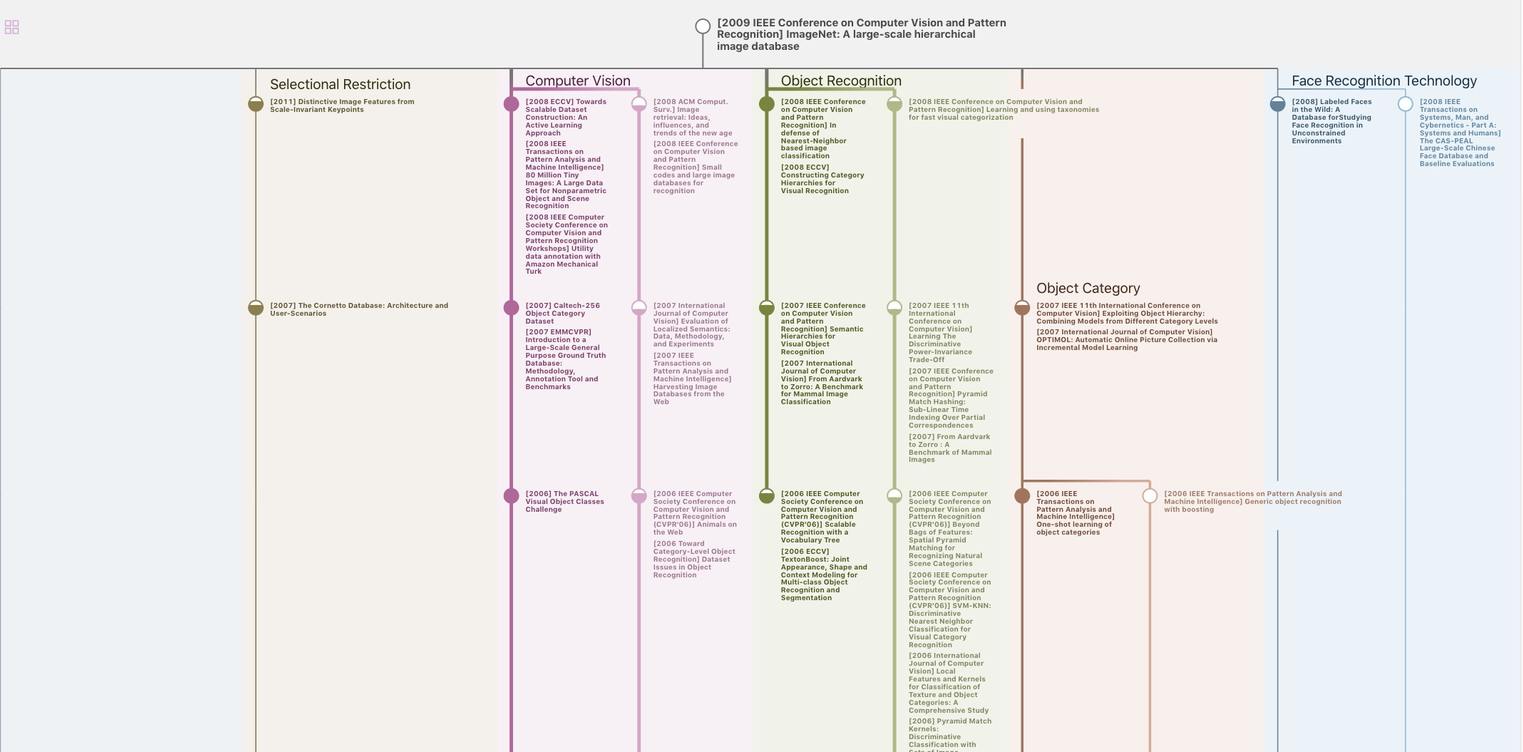
生成溯源树,研究论文发展脉络
Chat Paper
正在生成论文摘要