Consistency and Variation in Kernel Neural Ranking Model.
SIGIR(2018)
摘要
This paper studies the consistency of the kernel-based neural ranking model K-NRM, a recent state-of-the-art neural IR model, which is important for reproducible research and deployment in the industry. We find that K-NRM has low variance on relevance-based metrics across experimental trials. In spite of this low variance in overall performance, different trials produce different document rankings for individual queries. The main source of variance in our experiments was found to be different latent matching patterns captured by K-NRM. In the IR-customized word embeddings learned by K-NRM, the query-document word pairs follow two different matching patterns that are equally effective, but align word pairs differently in the embedding space. The different latent matching patterns enable a simple yet effective approach to construct ensemble rankers, which improve K-NRM's effectiveness and generalization abilities.
更多查看译文
关键词
Neural-IR,Retrieval Model Stability,Ensemble-Rankers
AI 理解论文
溯源树
样例
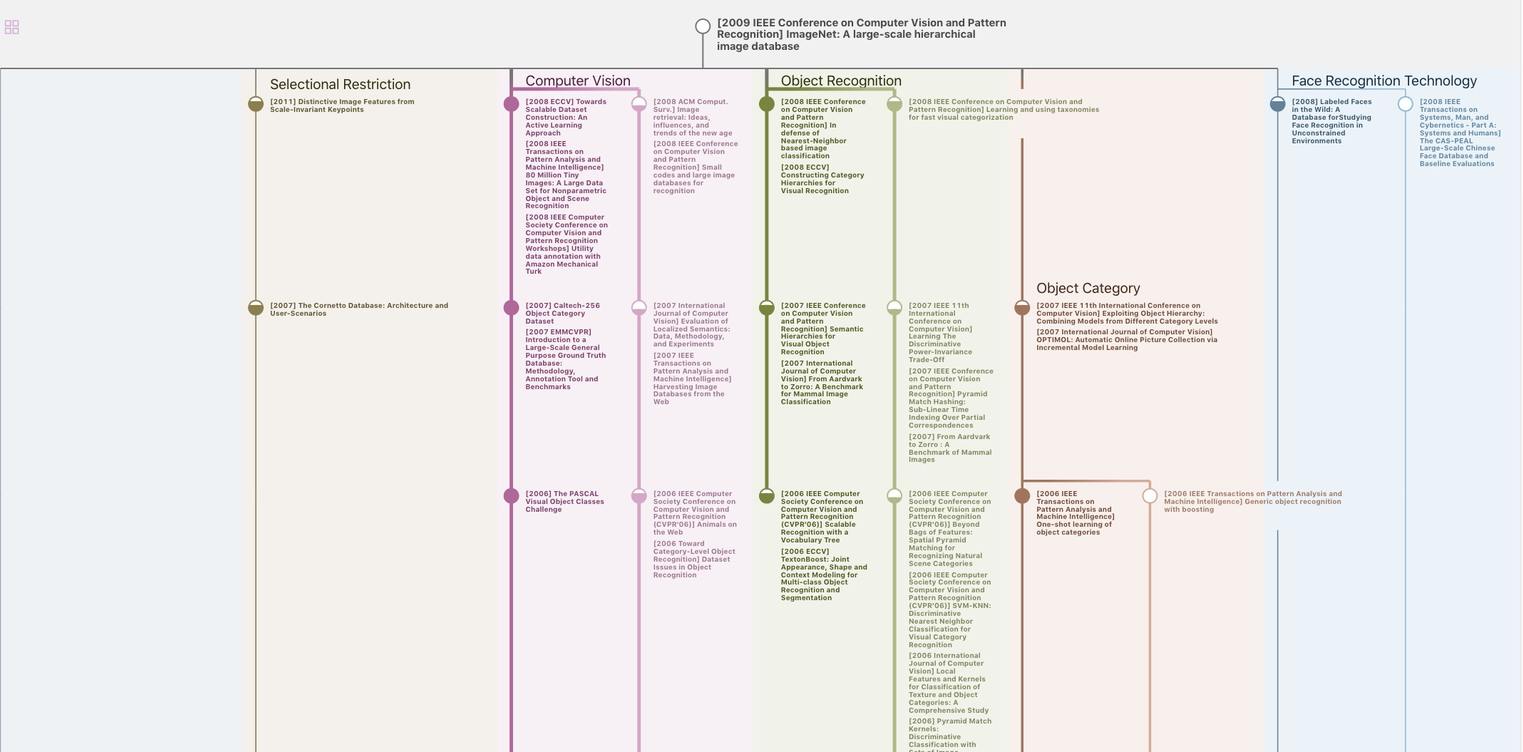
生成溯源树,研究论文发展脉络
Chat Paper
正在生成论文摘要