On the Computation of Kantorovich-Wasserstein Distances between 2D-Histograms by Uncapacitated Minimum Cost Flows
arXiv: Optimization and Control(2018)
摘要
In this work, we present a method to compute the Kantorovich-Wasserstein distance of order one between a pair of two-dimensional histograms. Recent works in Computer Vision and Machine Learning have shown the benefits of measuring Wasserstein distances of order one between histograms with n bins, by solving a classical transportation problem on very large complete bipartite graphs with n nodes and n^2 edges. The main contribution of our work is to approximate the original transportation problem by an uncapacitated min cost flow problem on a reduced flow network of size O(n) that exploits the geometric structure of the cost function. More precisely, when the distance among the bin centers is measured with the 1-norm or the ∞-norm, our approach provides an optimal solution. When the distance among bins is measured with the 2-norm: (i) we derive a quantitative estimate on the error between optimal and approximate solution; (ii) given the error, we construct a reduced flow network of size O(n). We numerically show the benefits of our approach by computing Wasserstein distances of order one on a set of grey scale images used as benchmark in the literature. We show how our approach scales with the size of the images with 1-norm, 2-norm and ∞-norm ground distances, and we compare it with other two methods which are largely used in the literature.
更多查看译文
AI 理解论文
溯源树
样例
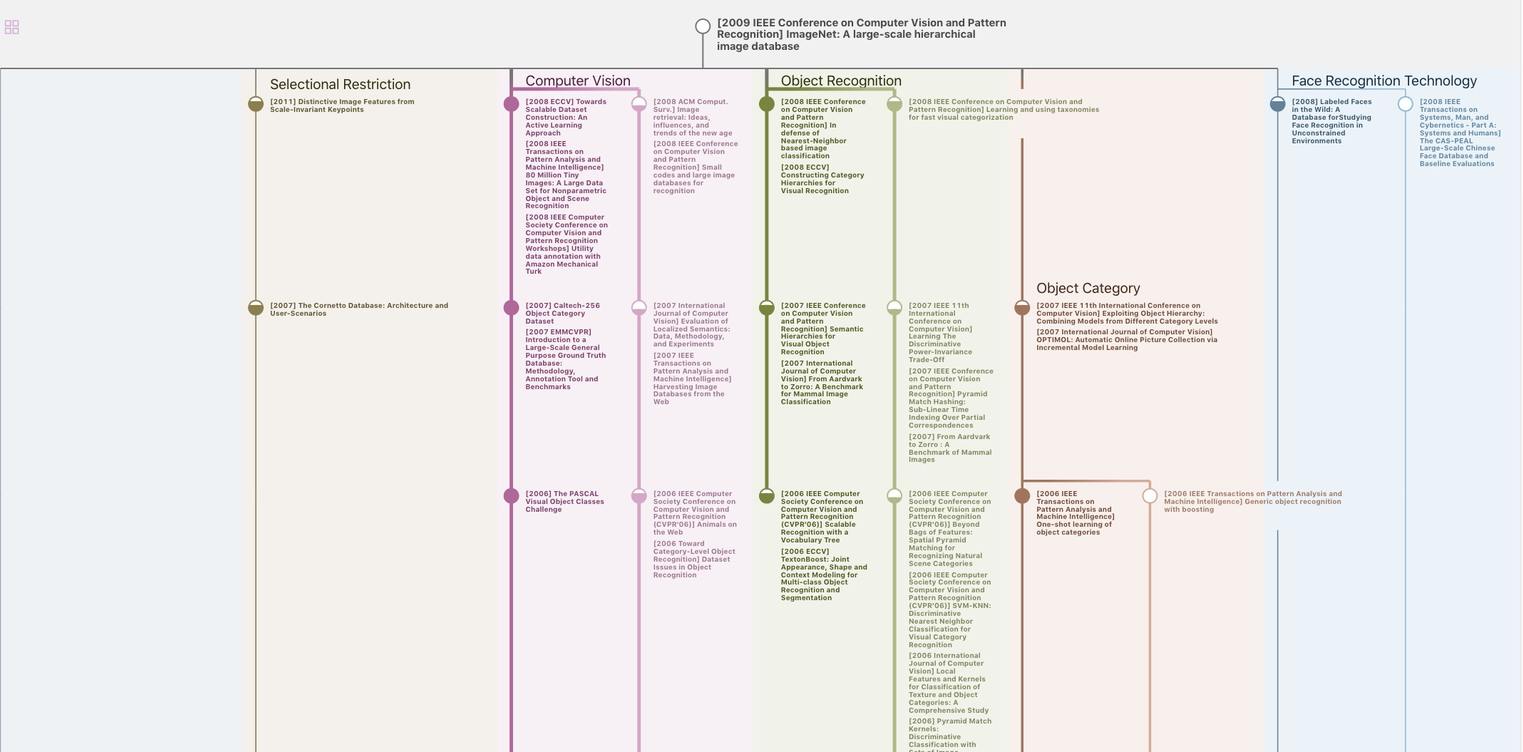
生成溯源树,研究论文发展脉络
Chat Paper
正在生成论文摘要