High-performance spiking neural net accelerators for embedded computer vision applications
2017 IEEE SOI-3D-Subthreshold Microelectronics Technology Unified Conference (S3S)(2017)
摘要
One key component in computer vision algorithms involves developing and identifying relevant features from raw data. In this work, we designed spiking recurrent neural net accelerators to implement a class of unsupervised machine learning algorithms known as sparse coding. The accelerators perform fast unsupervised learning of features, and extract sparse representations of inputs for low-power classification. Taking advantage of high sparsity, spiking neurons, and error tolerance, the compact accelerator chips are capable of processing images at several hundred megapixels per second, while dissipating less than 10 mW. The accelerators can be embedded in sensors as frontend processors for feature learning, encoding, and compression.
更多查看译文
关键词
low-power classification,compact accelerator chips,feature learning,high-performance spiking neural net accelerators,computer vision algorithms,recurrent neural net accelerators,unsupervised machine learning algorithms,sparse coding,embedded computer vision,sparse representations,image processing
AI 理解论文
溯源树
样例
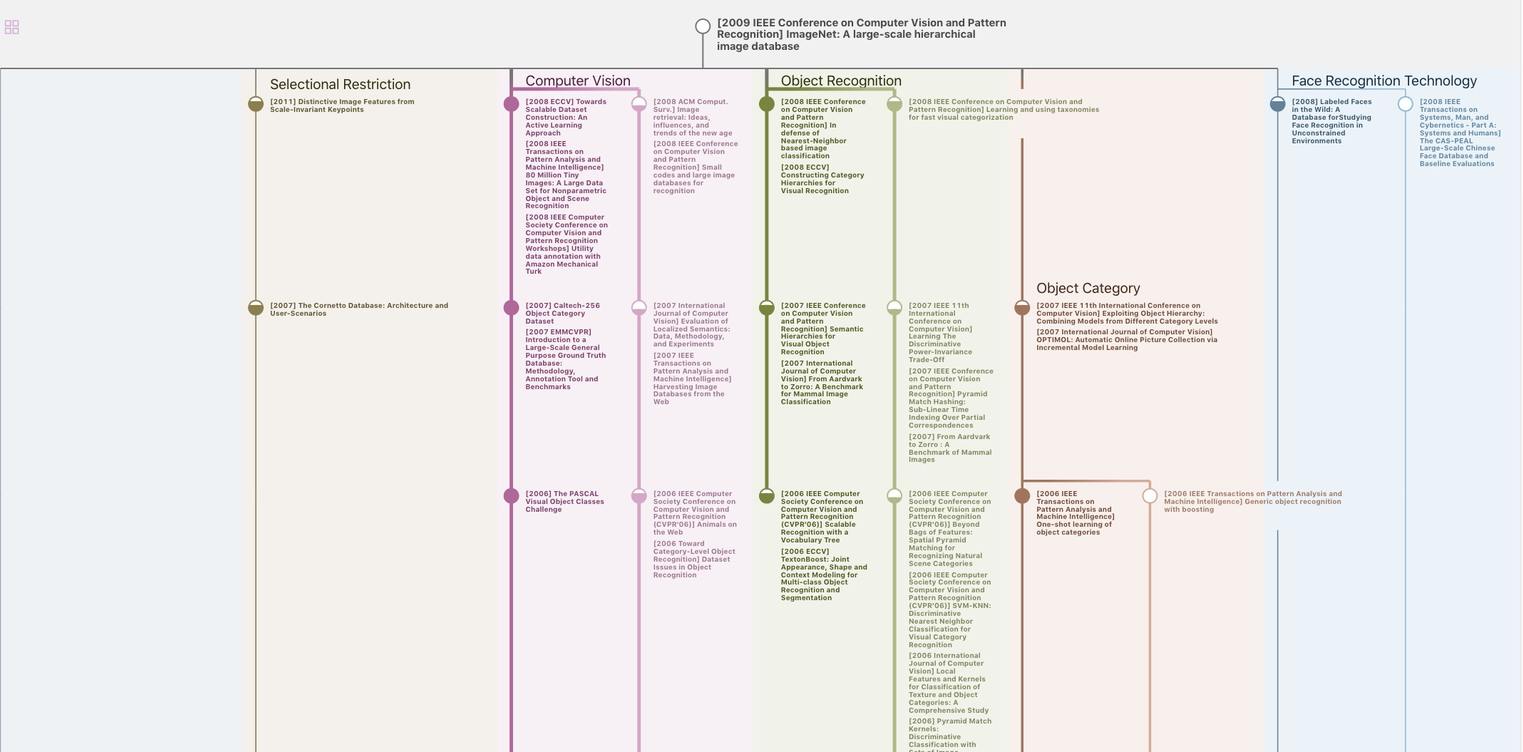
生成溯源树,研究论文发展脉络
Chat Paper
正在生成论文摘要