Learning on Partial-Order Hypergraphs.
WWW '18: The Web Conference 2018 Lyon France April, 2018(2018)
摘要
Graph-based learning methods explicitly consider the relations between two entities (i.e., vertices) for learning the prediction function. They have been widely used in semi-supervised learning, manifold ranking, and clustering, among other tasks. Enhancing the expressiveness of simple graphs, hypergraphs formulate an edge as a link to multiple vertices, so as to model the higher-order relations among entities. For example, hyperedges in a hypergraph can be used to encode the similarity among vertices.
To the best of our knowledge, all existing hypergraph structures represent the hyperedge as an unordered set of vertices, without considering the possible ordering relationship among vertices. In real-world data, ordering relations commonly exist, such as in graded categorical features (e.g., users» ratings on movies) and numerical features (e.g., monthly income of customers). When constructing a hypergraph, ignoring such ordering relations among entities will lead to severe information loss, resulting in suboptimal performance of the subsequent learning algorithms.
In this work, we address the inherent limitation of existing hypergraphs by proposing a new data structure named Partial-Order Hypergraph, which specifically injects the partially ordering relations among vertices into a hyperedge. We develop regularization-based learning theories for partial-order hypergraphs, generalizing conventional hypergraph learning by incorporating logical rules that encode the partial-order relations. We apply our proposed method to two applications: university ranking from Web data and popularity prediction of online content. Extensive experiments demonstrate the superiority of our proposed partial-order hypergraphs, which consistently improve over conventional hypergraph methods.
更多查看译文
关键词
Hypergraph, Partial-Order Hypergraph, Graph-based Learning, University Ranking, Popularity Prediction
AI 理解论文
溯源树
样例
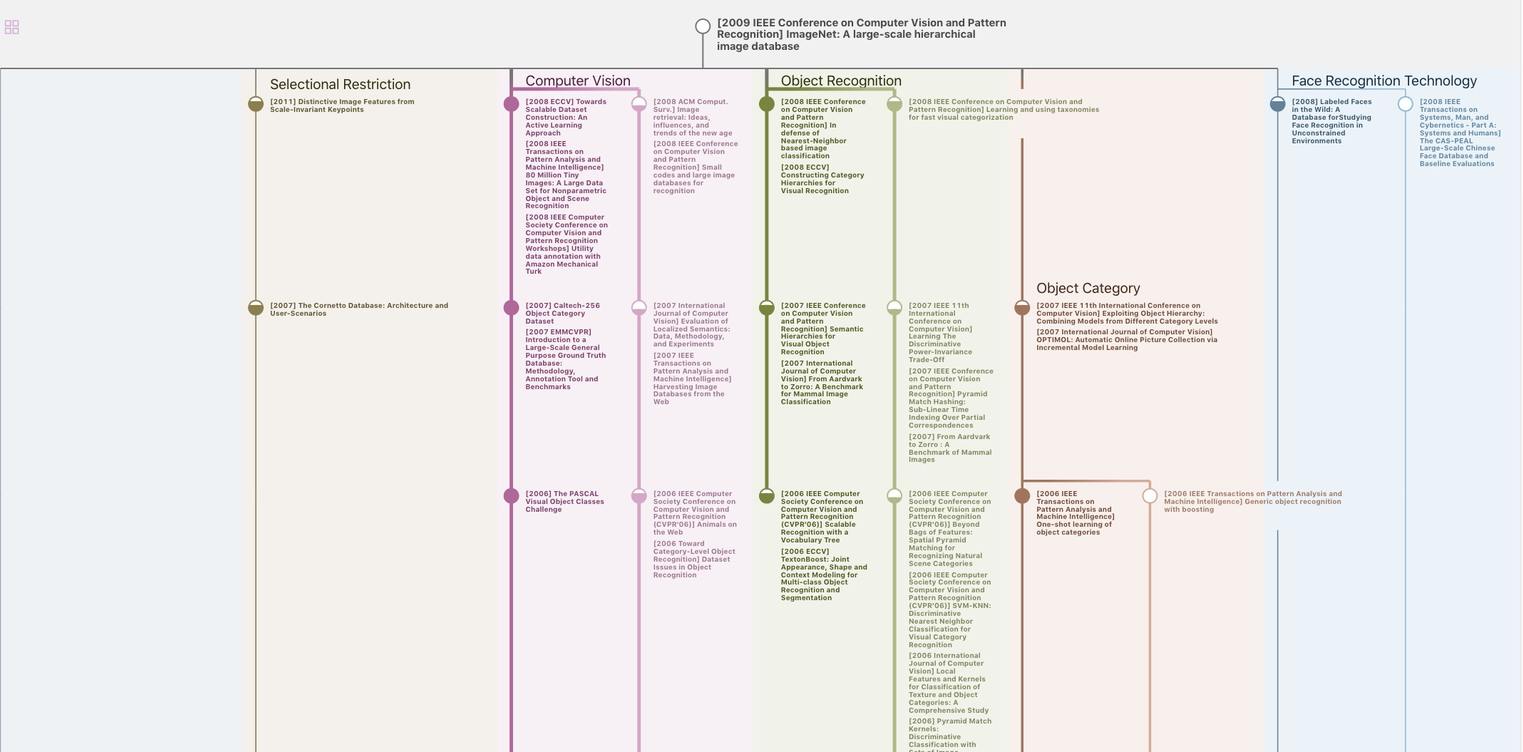
生成溯源树,研究论文发展脉络
Chat Paper
正在生成论文摘要