A Bayesian Network-Based Approach For Incremental Learning Of Uncertain Knowledge
INTERNATIONAL JOURNAL OF UNCERTAINTY FUZZINESS AND KNOWLEDGE-BASED SYSTEMS(2018)
摘要
Bayesian network (BN) is the well-accepted framework for representing and inferring uncertain knowledge. To learn the BN-based uncertain knowledge incrementally in response to the new data is useful for analysis, prediction, decision making, etc. In this paper, we propose an approach for incremental learning of BNs by focusing on the incremental revision of BN's graphical structures. First, we give the concept of influence degree to describe the influence of new data on the existing BN by measuring the variation of BN's probability parameters w.r.t. the likelihood of the new data. Then, for the nodes ordered decreasingly by their influence degrees, we give the scoring-based algorithm for revising BN's subgraphs iteratively by hill-climbing search for reversing, adding or deleting edges. In the incremental revision, we emphasize the preservation of probabilistic conditional independencies implied in the BN based on the concept and properties of Markov equivalence. Experimental results show the correctness, precision and efficiency of our approach.
更多查看译文
关键词
Uncertain knowledge, Bayesian network, incremental learning, influence degree, scoring function
AI 理解论文
溯源树
样例
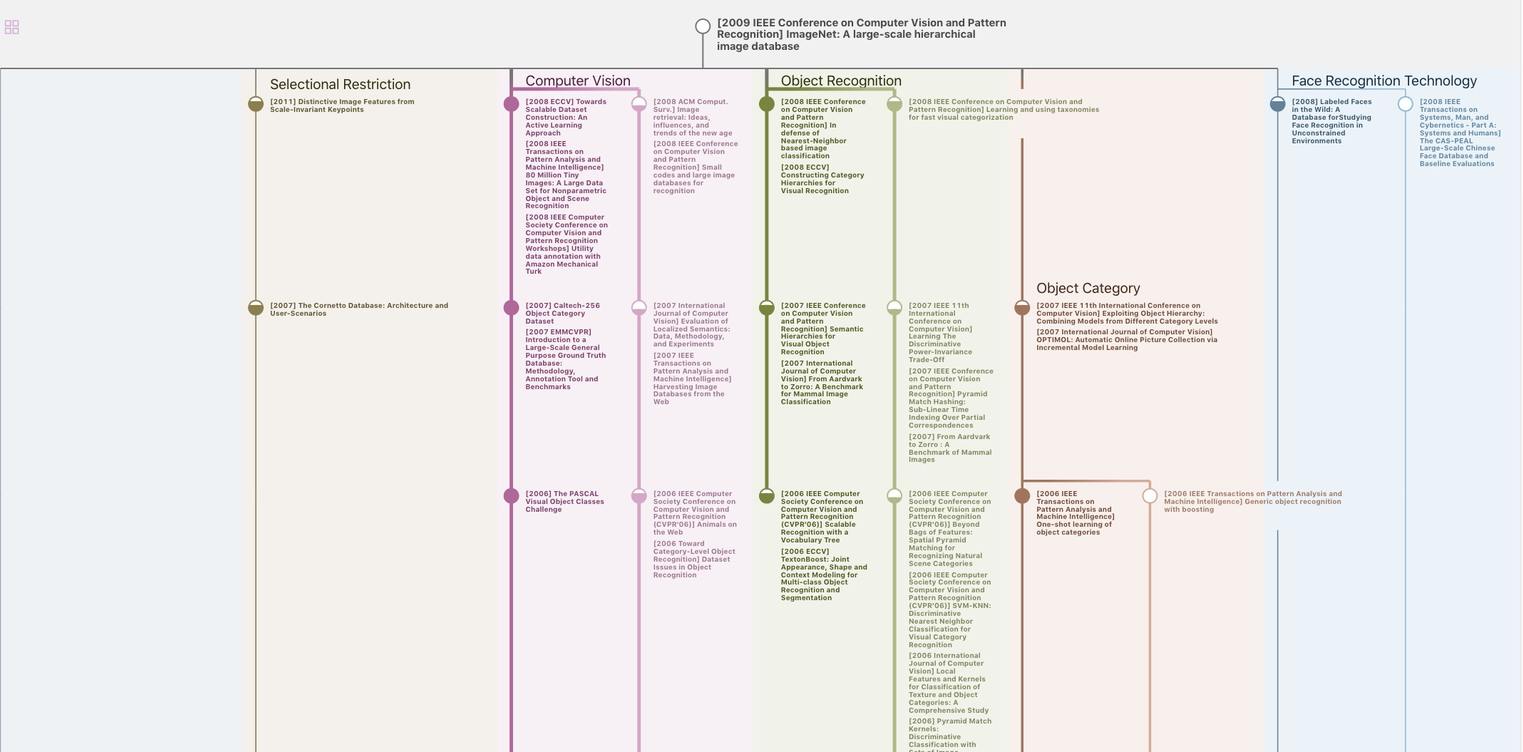
生成溯源树,研究论文发展脉络
Chat Paper
正在生成论文摘要