Knowledge Graph Embedding: A Locally and Temporally Adaptive Translation-Based Approach.
TWEB(2018)
摘要
A knowledge graph is a graph with entities of different types as nodes and various relations among them as edges. The construction of knowledge graphs in the past decades facilitates many applications, such as link prediction, web search analysis, question answering, and so on. Knowledge graph embedding aims to represent entities and relations in a large-scale knowledge graph as elements in a continuous vector space. Existing methods, for example, TransE, TransH, and TransR, learn the embedding representation by defining a global margin-based loss function over the data. However, the loss function is determined during experiments whose parameters are examined among a closed set of candidates. Moreover, embeddings over two knowledge graphs with different entities and relations share the same set of candidates, ignoring the locality of both graphs. This leads to the limited performance of embedding related applications. In this article, a locally adaptive translation method for knowledge graph embedding, called TransA, is proposed to find the loss function by adaptively determining its margin over different knowledge graphs. Then the convergence of TransA is verified from the aspect of its uniform stability. To make the embedding methods up-to-date when new vertices and edges are added into the knowledge graph, the incremental algorithm for TransA, called iTransA, is proposed by adaptively adjusting the optimal margin over time. Experiments on four benchmark data sets demonstrate the superiority of the proposed method, as compared to the state-of-the-art ones.
更多查看译文
关键词
Locally and temporally adaptive translation, convergence, knowledge graph embedding, optimal margin
AI 理解论文
溯源树
样例
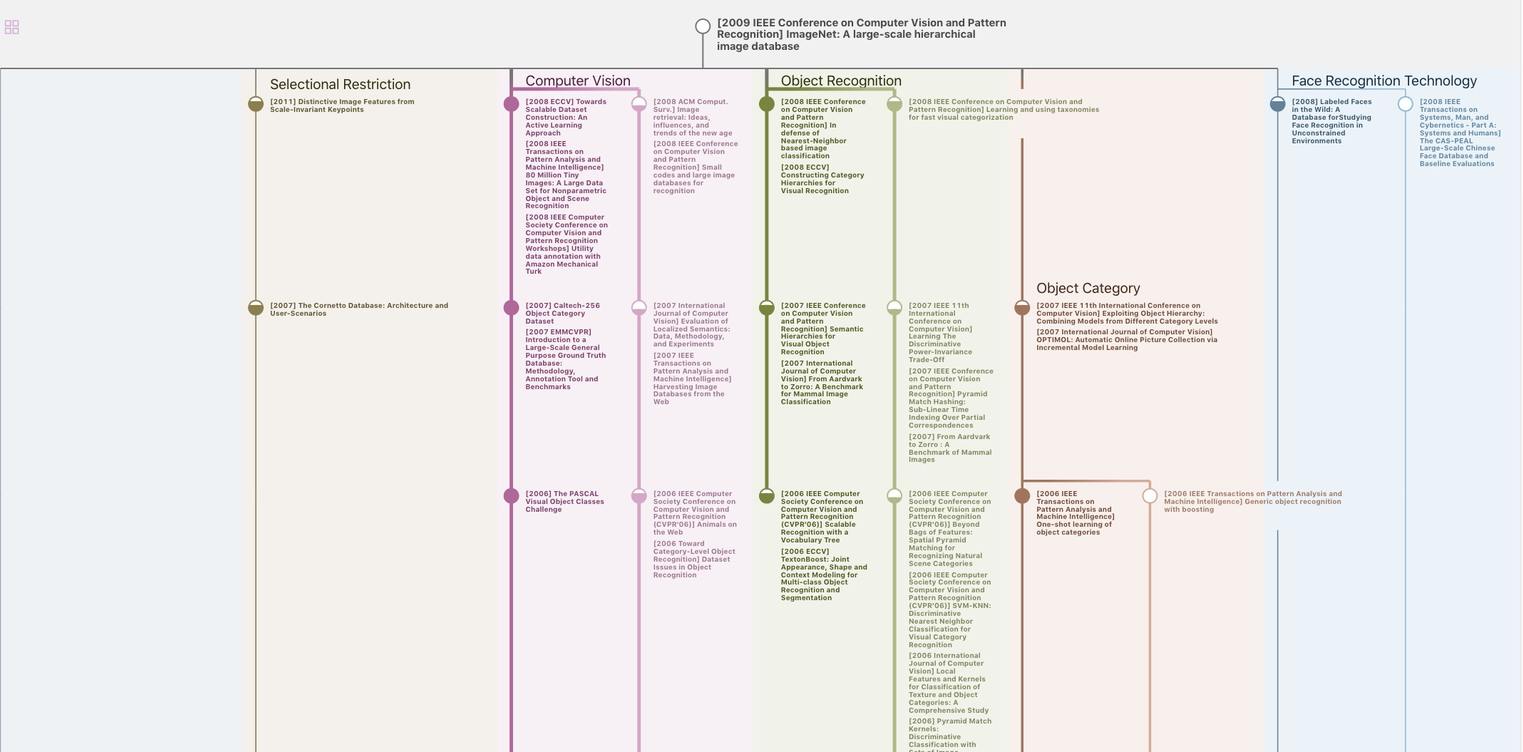
生成溯源树,研究论文发展脉络
Chat Paper
正在生成论文摘要