Generalization capability of neural networks based on fuzzy operators
APPLIED AND COMPUTATIONAL MATHEMATICS(2011)
Abstract
This paper discusses the generalization capability of neural networks based on various fuzzy operators introduced earlier by the authors as Fuzzy Flip-Flop based Neural Networks (FNNs), in comparison with standard (e.g. tansig function based, MATLAB Neural Network Toolbox type) networks in the frame of simple function approximation problems. Various fuzzy neurons, one of them based on a pair of new fuzzy intersection and union, and several other selected well known fuzzy operators (Lukasiewicz and Dombi operators) combined with standard negation have been proposed as suitable for the construction of novel FNNs. We briefly present the sigmoid function generators derived from fuzzy J-K and D flip-flops. An advantage of such FNNs is their easy hardware implementability. The experimental results show that these FNNs provide rather good generalization performance, with far better mathematical stability than the standard tansig based neural networks and are more suitable to avoid overfitting in the case of test data containing noisy items in the form of outliers.
MoreTranslated text
Key words
Multilayer Perceptrons Based on Fuzzy Flip-Flops,Bacterial Memetic Algorithm With Modified Operator Execution Order,Fuzzy Neural Networks Generalization Capability
AI Read Science
Must-Reading Tree
Example
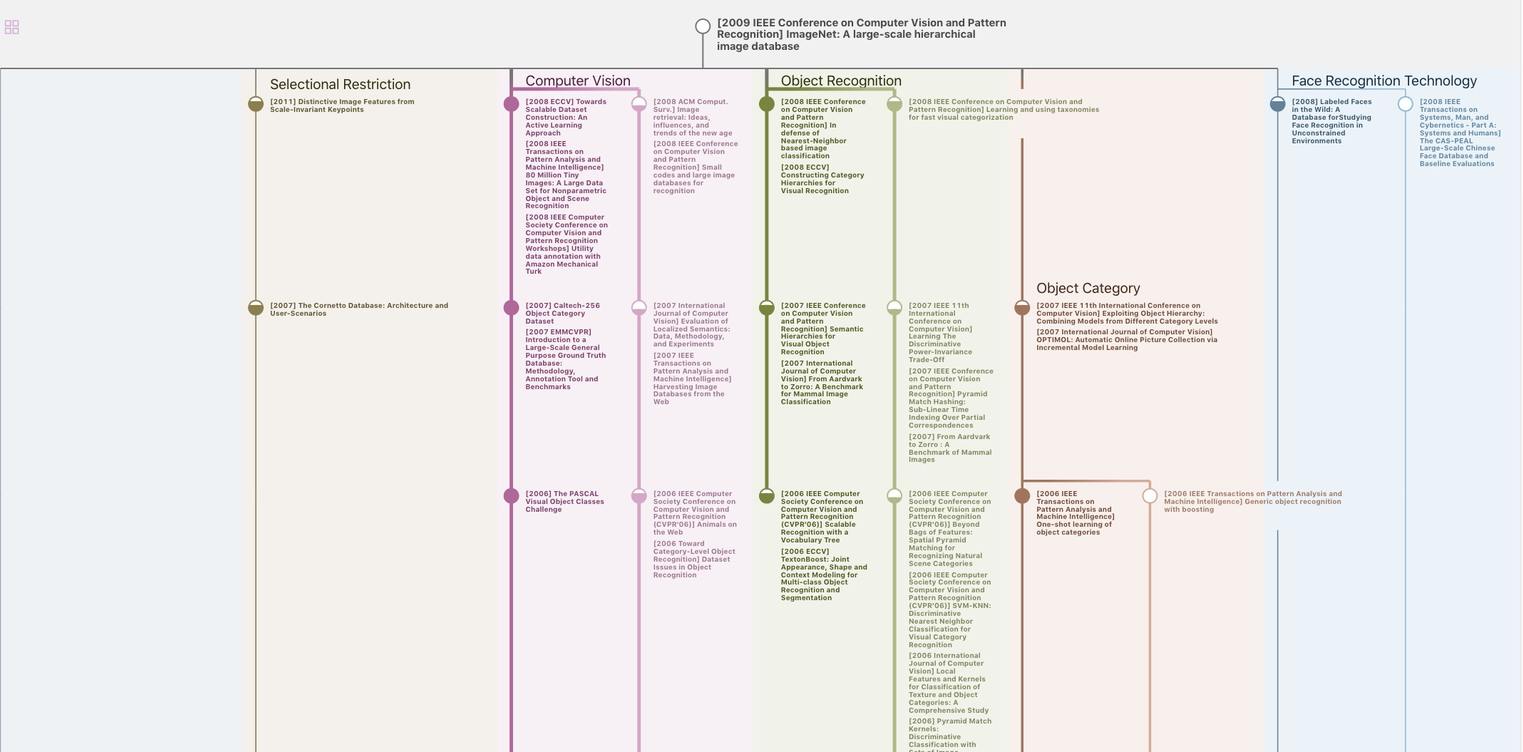
Generate MRT to find the research sequence of this paper
Chat Paper
Summary is being generated by the instructions you defined