Land Cover Classification From Multispectral Data Using Computational Intelligence Tools: A Comparative Study
INFORMATION(2017)
摘要
This article discusses how computational intelligence techniques are applied to fuse spectral images into a higher level image of land cover distribution for remote sensing, specifically for satellite image classification. We compare a fuzzy-inference method with two other computational intelligence methods, decision trees and neural networks, using a case study of land cover classification from satellite images. Further, an unsupervised approach based on k-means clustering has been also taken into consideration for comparison. The fuzzy-inference method includes training the classifier with a fuzzy-fusion technique and then performing land cover classification using reinforcement aggregation operators. To assess the robustness of the four methods, a comparative study including three years of land cover maps for the district of Mandimba, Niassa province, Mozambique, was undertaken. Our results show that the fuzzy-fusion method performs similarly to decision trees, achieving reliable classifications; neural networks suffer from overfitting; while k-means clustering constitutes a promising technique to identify land cover types from unknown areas.
更多查看译文
关键词
image fusion, aggregation operators, remote sensing, land cover classification
AI 理解论文
溯源树
样例
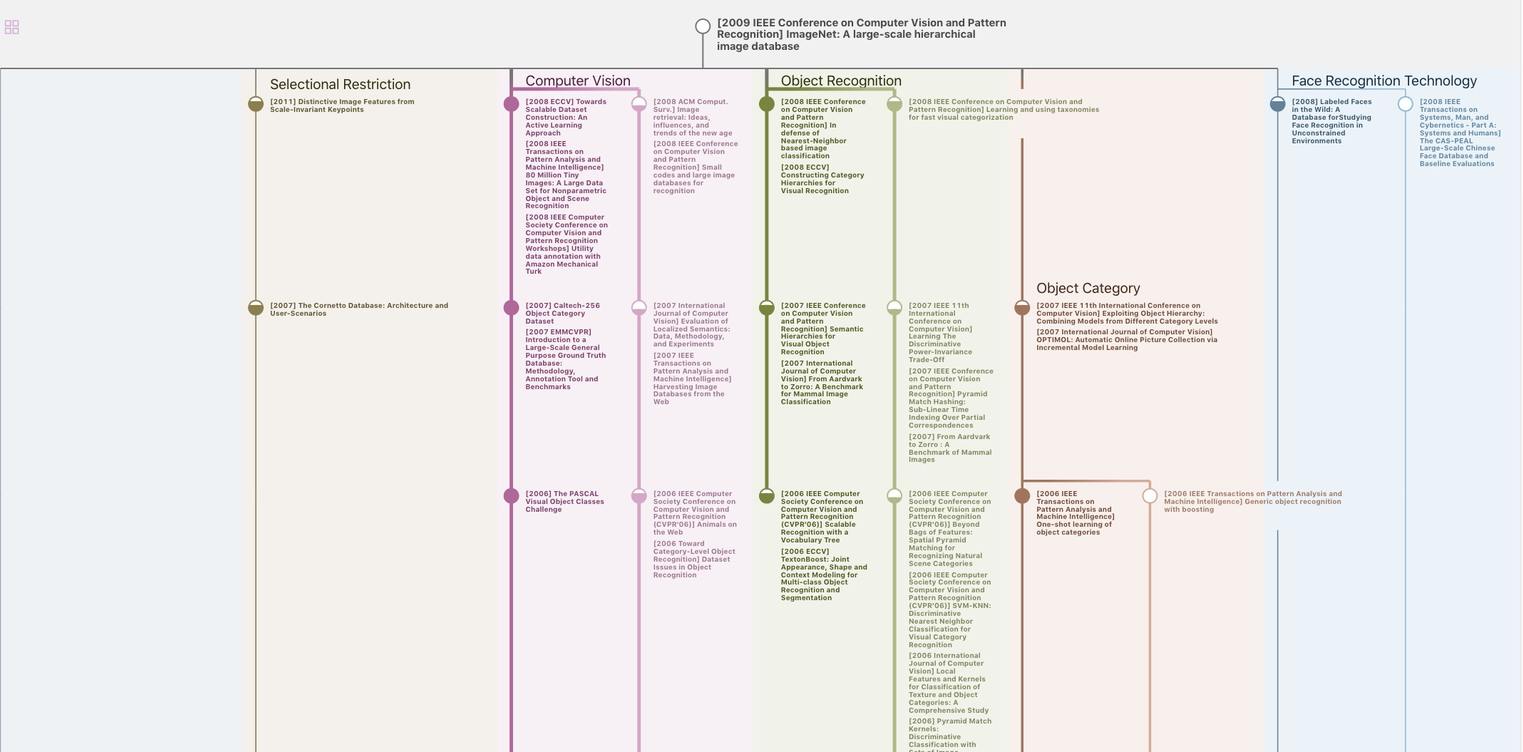
生成溯源树,研究论文发展脉络
Chat Paper
正在生成论文摘要