A Practical Framework of Conversion Rate Prediction for Online Display Advertising
ADKDD'17 23RD ACM SIGKDD CONFERENCE ON KNOWLEDGE DISCOVERY AND DATA MINING (KDD 2017)(2017)
摘要
Cost-per-action (CPA), or cost-per-acquisition, has become the primary campaign performance objective in online advertising industry. As a result, accurate conversion rate (CVR) prediction is crucial for any real-time bidding (RTB) platform. However, CVR prediction is quite challenging due to several factors, including extremely sparse conversions, delayed feedback, attribution gaps between the platform and the third party, etc. In order to tackle these challenges, we proposed a practical framework that has been successfully deployed on Yahoo! BrightRoll, one of the largest RTB ad buying platforms. In this paper, we first show that over-prediction and the resulted over-bidding are fundamental challenges for CPA campaigns in a real RTB environment. We then propose a safe prediction framework with conversion attribution adjustment to handle over-predictions and to further alleviate over-bidding at different levels. At last, we illustrate both offline and online experimental results to demonstrate the effectiveness of the framework.
更多查看译文
关键词
display advertising,demand-side platform,real-time bidding,large-scale learning
AI 理解论文
溯源树
样例
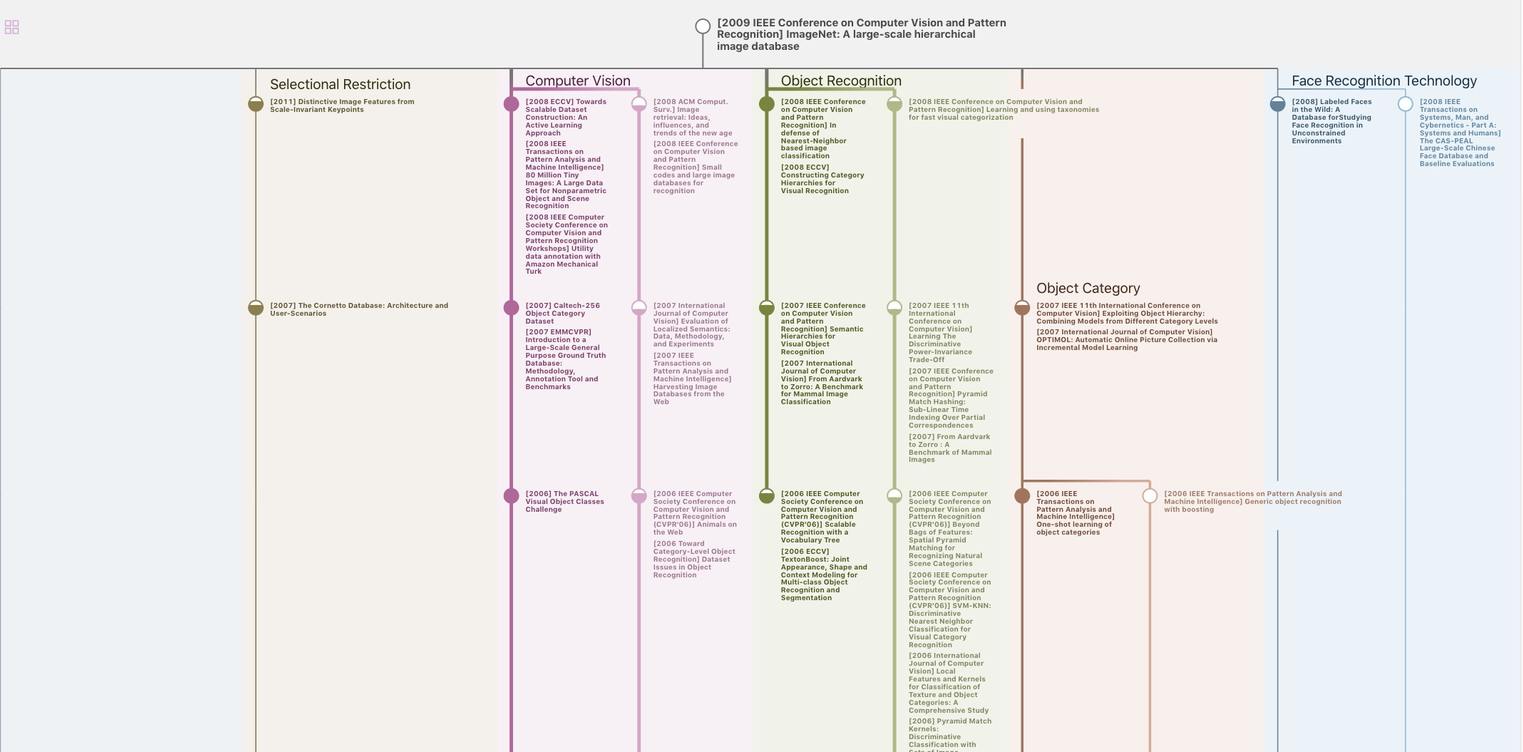
生成溯源树,研究论文发展脉络
Chat Paper
正在生成论文摘要