Symbiotic Tracker Ensemble with Feedback Learning
2017 30th SIBGRAPI Conference on Graphics, Patterns and Images (SIBGRAPI)(2017)
Abstract
Visual tracking is a challenging task due to a number of factors, such as occlusions, deformations, illumination variations and abrupt motion changes present in a video sequence. Generally, trackers are robust to some of these factors, but do not achieve satisfactory results when dealing with multiple factors at the same time. More robust results when multiple factors are present can be obtained by combining the results of different trackers. In this paper we propose a multiple tracker fusion method, named Symbiotic Tracker Ensemble with Feedback Learning (SymTE-FL), which combines the results of a set of trackers to produce a unified tracking estimate. The novelty of the method consists in providing feedback to the individual trackers, so that they can correct their own estimates, thus improving overall tracking accuracy. The proposal is validated by experiments conducted upon a publicly available database. The results show that the proposed method delivered in average more accurate tracking estimates than those obtained with individual trackers running independently and with the original approach.
MoreTranslated text
Key words
Object tracking,tracking fusion
AI Read Science
Must-Reading Tree
Example
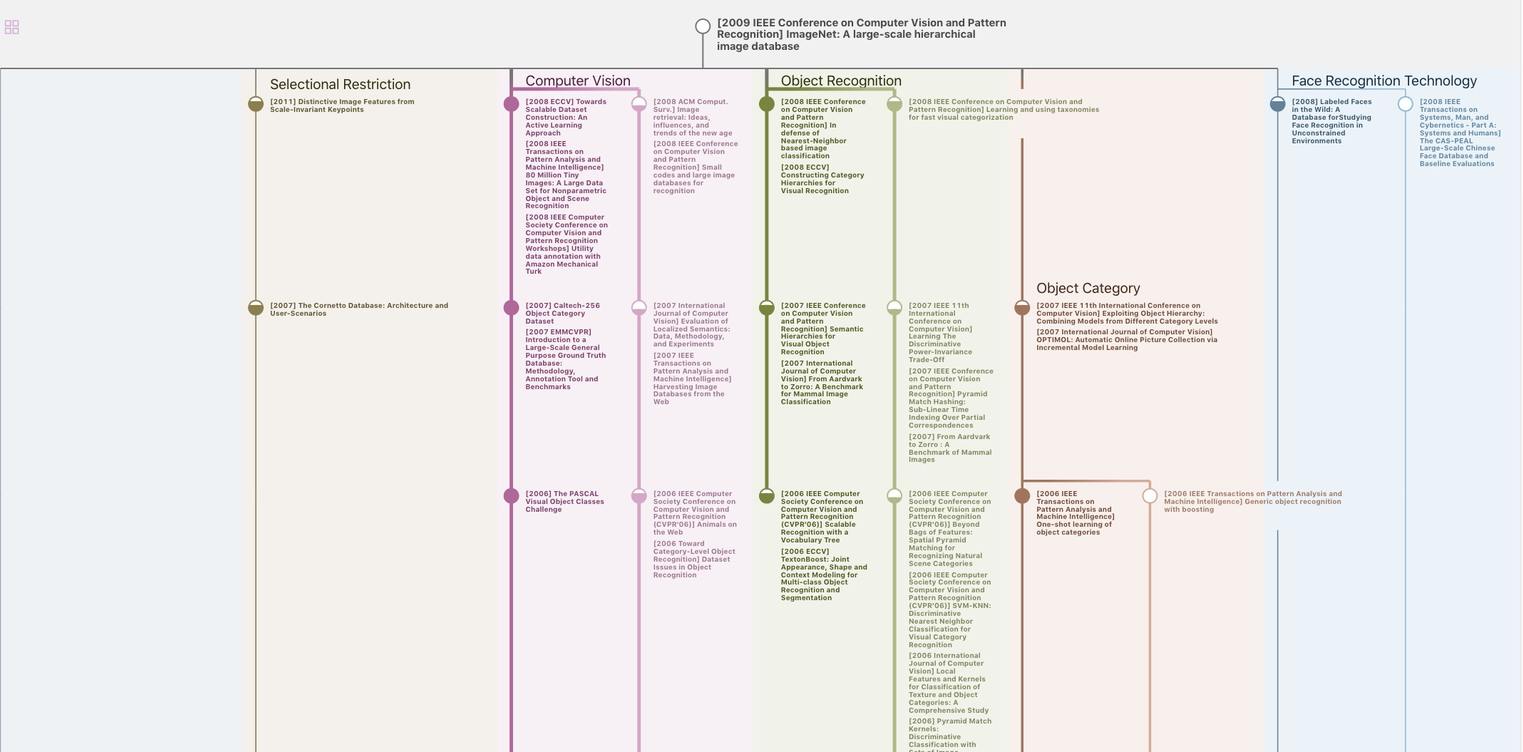
Generate MRT to find the research sequence of this paper
Chat Paper
Summary is being generated by the instructions you defined