Fast Top-k Area Topics Extraction with Knowledge Base
2018 IEEE Third International Conference on Data Science in Cyberspace (DSC)(2017)
摘要
What are the most popular research topics in Artificial Intelligence (AI)? We formulate the problem as extracting top-k topics that can best represent a given area with the help of knowledge base. We theoretically prove that the problem is NP-hard and propose an optimization model, FastKATE, to address this problem by combining both explicit and latent representations for each topic. We leverage a large-scale knowledge base (Wikipedia) to generate topic embeddings using neural networks and use this kind of representations to help capture the representativeness of topics for given areas. We develop a fast heuristic algorithm to efficiently solve the problem with a provable error bound. We evaluate the proposed model on three real-world datasets. Experimental results demonstrate our model's effectiveness, robustness, real-timeness (return results in <1s), and its superiority over several alternative methods.
更多查看译文
关键词
knowledge discovery,data mining,topic extraction,knowledge base,heuristic search
AI 理解论文
溯源树
样例
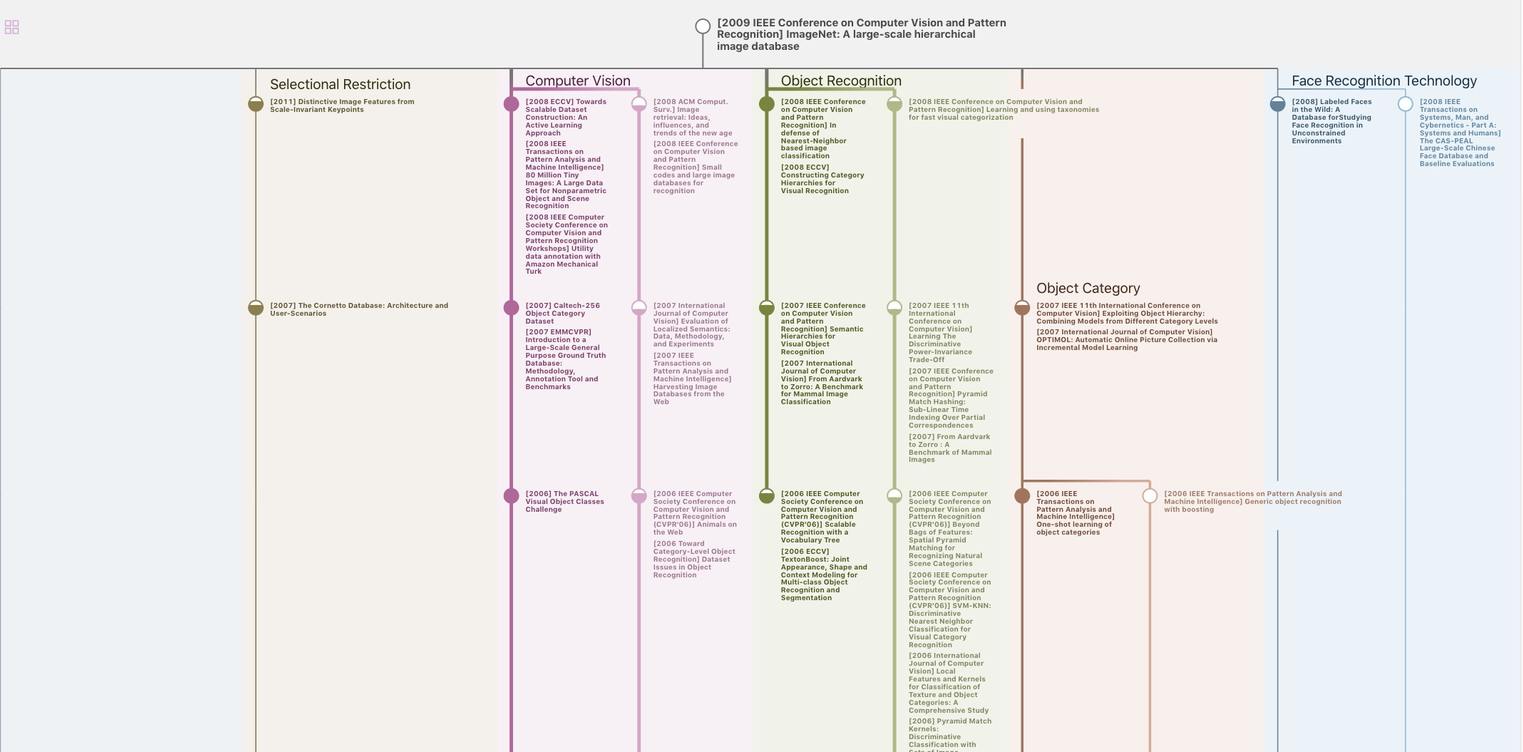
生成溯源树,研究论文发展脉络
Chat Paper
正在生成论文摘要