Deep Learning Based Forecasting of Critical Infrastructure Data.
CIKM(2017)
摘要
Intelligent monitoring and control of critical infrastructure such as electric power grids, public water utilities and transportation systems produces massive volumes of time series data from heterogeneous sensor networks. Time Series Forecasting (TSF) is essential for system safety and security, and also for improving the efficiency and quality of service delivery. Being highly dependent on various external factors, the observed system behavior is usually stochastic, which makes the next value prediction a tricky and challenging task that usually needs customized methods. In this paper we propose a novel deep learning based framework for time series analysis and prediction by ensembling parametric and nonparametric methods. Our approach takes advantage of extracting features at different time scales, which improves accuracy without compromising reliability in comparison with the state-of-the-art methods. Our experimental evaluation using real-world SCADA data from a municipal water management system shows that our proposed method outperforms the baseline methods evaluated here.
更多查看译文
关键词
Deep learning, Time series forecasting, Multimodal learning, Critical infrastructure protection
AI 理解论文
溯源树
样例
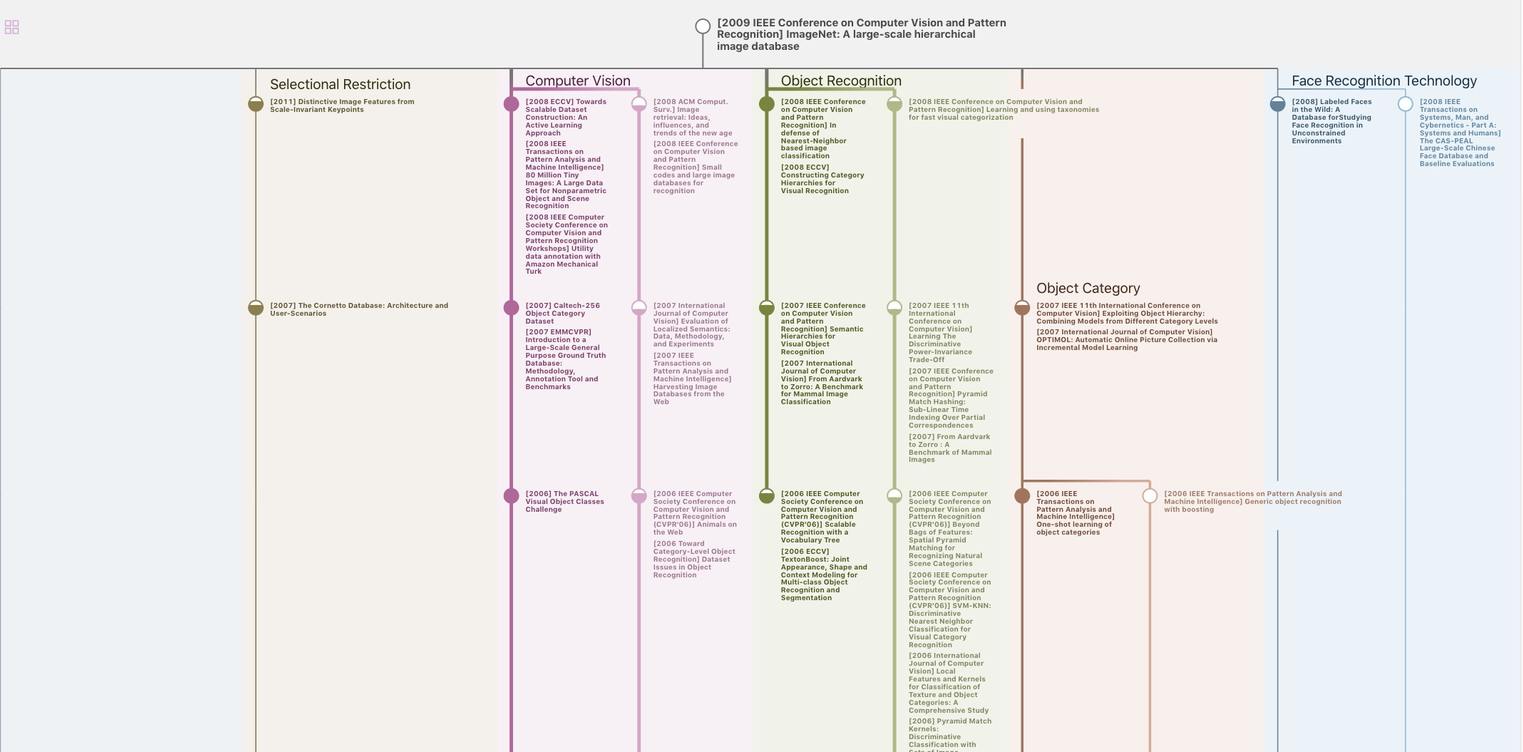
生成溯源树,研究论文发展脉络
Chat Paper
正在生成论文摘要