Maximum regularized likelihood estimators: A general prediction theory and applications: Maximum regularized likelihood estimators
STAT(2018)
Abstract
Maximum regularized likelihood estimators (MRLEs) are arguably the most established class of estimators in high-dimensional statistics. In this paper, we derive guarantees for MRLEs in the Kullback-Leibler divergence, a general measure of prediction accuracy. We assume only that the densities have a convex parametrization and that the regularization is definite and positive homogenous. The results thus apply to a very large variety of models and estimators, such as tensor regression and graphical models with convex and non-convex regularized methods. A main conclusion is that MRLEs are broadly consistent in prediction-regardless of whether restricted eigenvalues or similar conditions hold. Copyright (c) 2018 John Wiley & Sons, Ltd.
MoreTranslated text
Key words
maximum regularized likelihood estimators,oracle inequalities,prediction accuracy
AI Read Science
Must-Reading Tree
Example
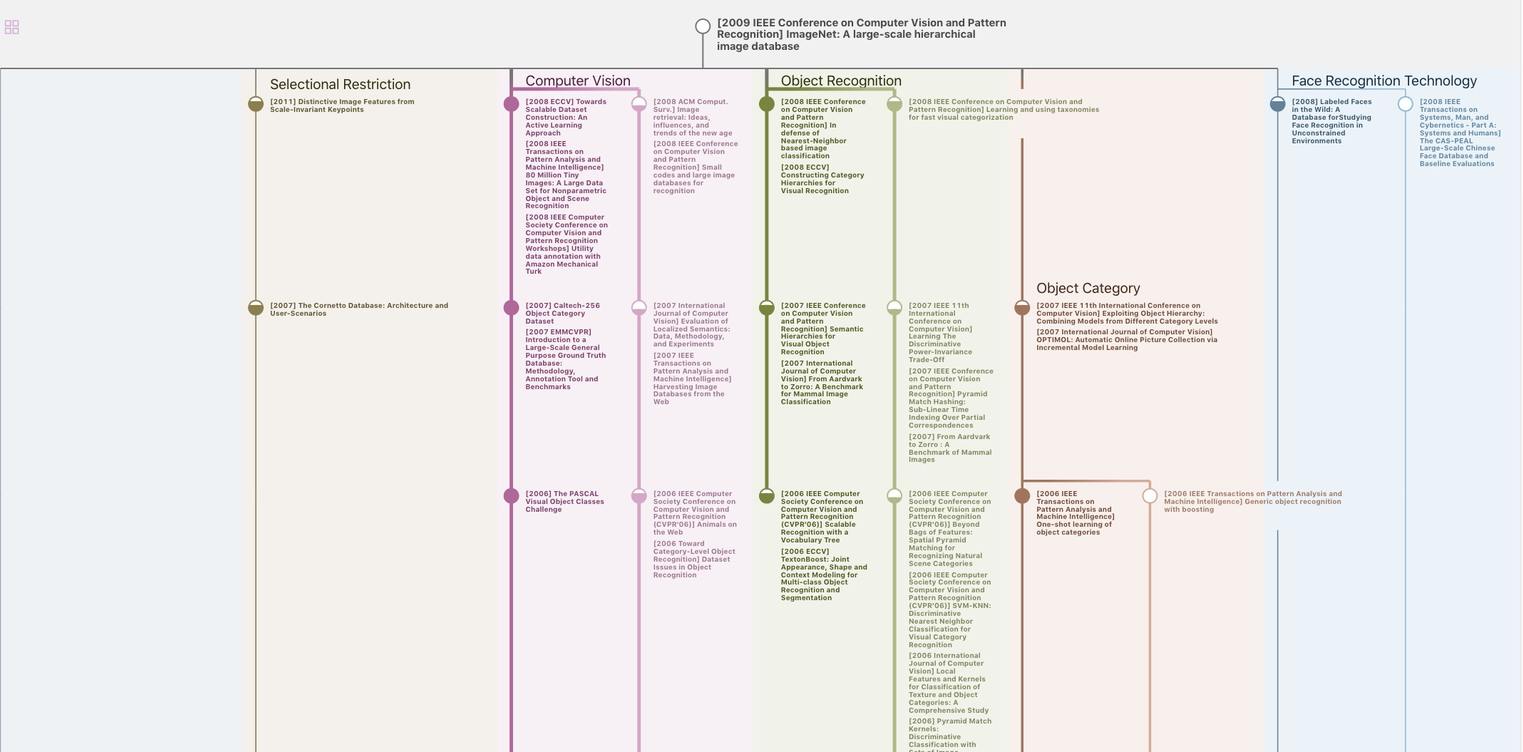
Generate MRT to find the research sequence of this paper
Chat Paper
Summary is being generated by the instructions you defined