Grey Wolf Optimisation For Inversion Of Layered Earth Geophysical Datasets
NEAR SURFACE GEOPHYSICS(2017)
摘要
Geophysical observables using optimisation algorithms are interpreted in terms of physical properties defining the Earth. The field of optimisation is a dynamic field as no single algorithm can solve all optimisation problems. We implement the grey wolf algorithm, an apex predator-based method, in optimizing geophysical datasets over a layered earth. The grey wolf optimiser is a swarm-based metaheuristic algorithm and has two extremely interesting social practices, viz., social leadership hierarchy and hunting behaviour. The leadership hierarchy is simulated by employing different types of grey wolves where hunting strategies are implemented as optimisation methods. Global minimum from the grey wolf optimiser has been obtained with a pack of 7 wolves and 1500 iterations. To evaluate the efficacy of the grey wolf optimiser, we performed inversion of noise-contaminated vertical electrical sounding (apparent resistivity), induced polarisation sounding (apparent chargeability), and magnetotelluric apparent resistivity data. Subsequently, we implemented grey wolf optimisation on field apparent resistivity, apparent chargeability, and magnetotelluric apparent resistivity data adapted from published literature. Both noise-contaminated synthetic and field data have been compared with popular population-based algorithms, i.e., particle swarm optimisation and ant colony optimisation and with a local optimisation algorithm-ridge regression. The sensitivity analysis was performed by inverting the noise-contaminated datasets using the grey wolf optimiser, particle swarm optimisation and ant colony optimisation with six different search space. It is observed that the grey wolf optimiser is least sensitive to the varied search space. The results obtained from the grey wolf optimiser as compared with other techniques are relatively more stable and the obtained normalised RMS deviation is either less or equal with ridge regression, particle swarm optimisation, or ant colony optimisation. This is due to the fact that the grey wolf optimiser does not converge prematurely and avoids getting trapped in a local minimum, as a balance between exploration and exploitation is maintained. We also compared the grey wolf optimiser using L1-norm and L2-norm as misfit functions for field data examples. The grey wolf optimiser using L1-norm resulted in a more stable solution. The execution time of the grey wolf optimiser is least as compared with other population optimisation techniques. Grey wolf optimisation could be applied for routine interpretation of geophysical datasets.
更多查看译文
关键词
layered earth,inversion
AI 理解论文
溯源树
样例
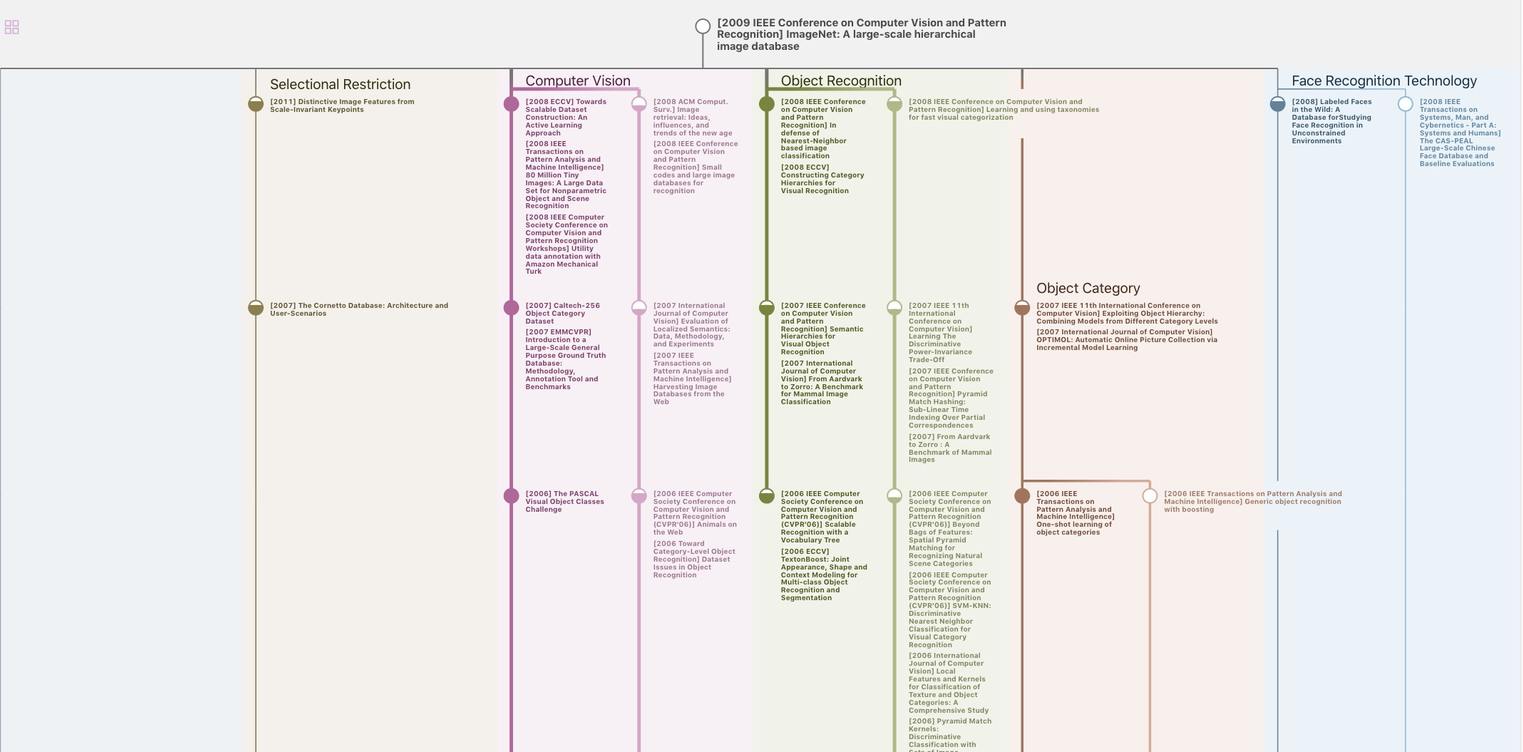
生成溯源树,研究论文发展脉络
Chat Paper
正在生成论文摘要