A Segmentation Method For Lung Nodule Image Sequences Based On Superpixels And Density-Based Spatial Clustering Of Applications With Noise
PLOS ONE(2017)
摘要
The fast and accurate segmentation of lung nodule image sequences is the basis of subsequent processing and diagnostic analyses. However, previous research investigating nodule segmentation algorithms cannot entirely segment cavitary nodules, and the segmentation of juxta-vascular nodules is inaccurate and inefficient. To solve these problems, we propose a new method for the segmentation of lung nodule image sequences based on superpixels and density-based spatial clustering of applications with noise (DBSCAN). First, our method uses three-dimensional computed tomography image features of the average intensity projection combined with multi-scale dot enhancement for preprocessing. Hexagonal clustering and morphological optimized sequential linear iterative clustering (HMSLIC) for sequence image oversegmentation is then proposed to obtain superpixel blocks. The adaptive weight coefficient is then constructed to calculate the distance required between superpixels to achieve precise lung nodules positioning and to obtain the subsequent clustering starting block. Moreover, by fitting the distance and detecting the change in slope, an accurate clustering threshold is obtained. Thereafter, a fast DBSCAN superpixel sequence clustering algorithm, which is optimized by the strategy of only clustering the lung nodules and adaptive threshold, is then used to obtain lung nodule mask sequences. Finally, the lung nodule image sequences are obtained. The experimental results show that our method rapidly, completely and accurately segments various types of lung nodule image sequences.
更多查看译文
关键词
lung nodule image sequences,superpixels,spatial clustering,segmentation method,density-based
AI 理解论文
溯源树
样例
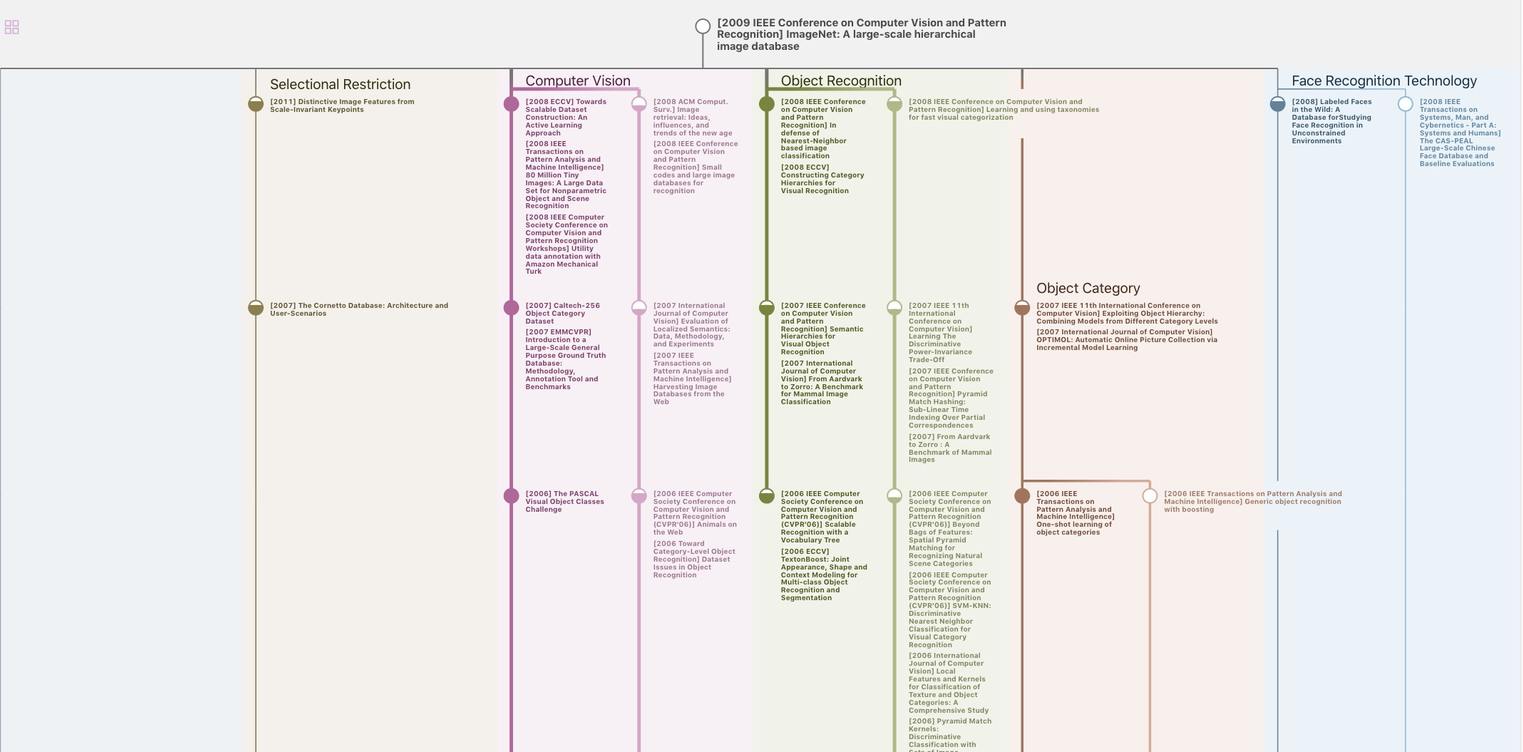
生成溯源树,研究论文发展脉络
Chat Paper
正在生成论文摘要