A Comparison Of Sequence-To-Sequence Models For Speech Recognition
18TH ANNUAL CONFERENCE OF THE INTERNATIONAL SPEECH COMMUNICATION ASSOCIATION (INTERSPEECH 2017), VOLS 1-6: SITUATED INTERACTION(2017)
摘要
In this work, we conduct a detailed evaluation of various all neural, end-to-end trained, sequence-to-sequence models applied to the task of speech recognition. Notably. each of these systems directly predicts graphemes in the written domain, without using an external pronunciation lexicon, or a separate language model. We examine several sequence-to-sequence models including connectionist temporal classification (CTC), the recurrent neural network (RNN) transducer, an attention based model, and a model which augments the RNN transducer with an attention mechanism.We find that the sequence-to-sequence models are competitive with traditional state-of-the-art approaches on dictation test sets, although the baseline, which uses a separate pronunciation and language model, outperforms these models on voice-search test sets.
更多查看译文
关键词
sequence-to-sequence models, attention models, end-to-end models, RNN transducer
AI 理解论文
溯源树
样例
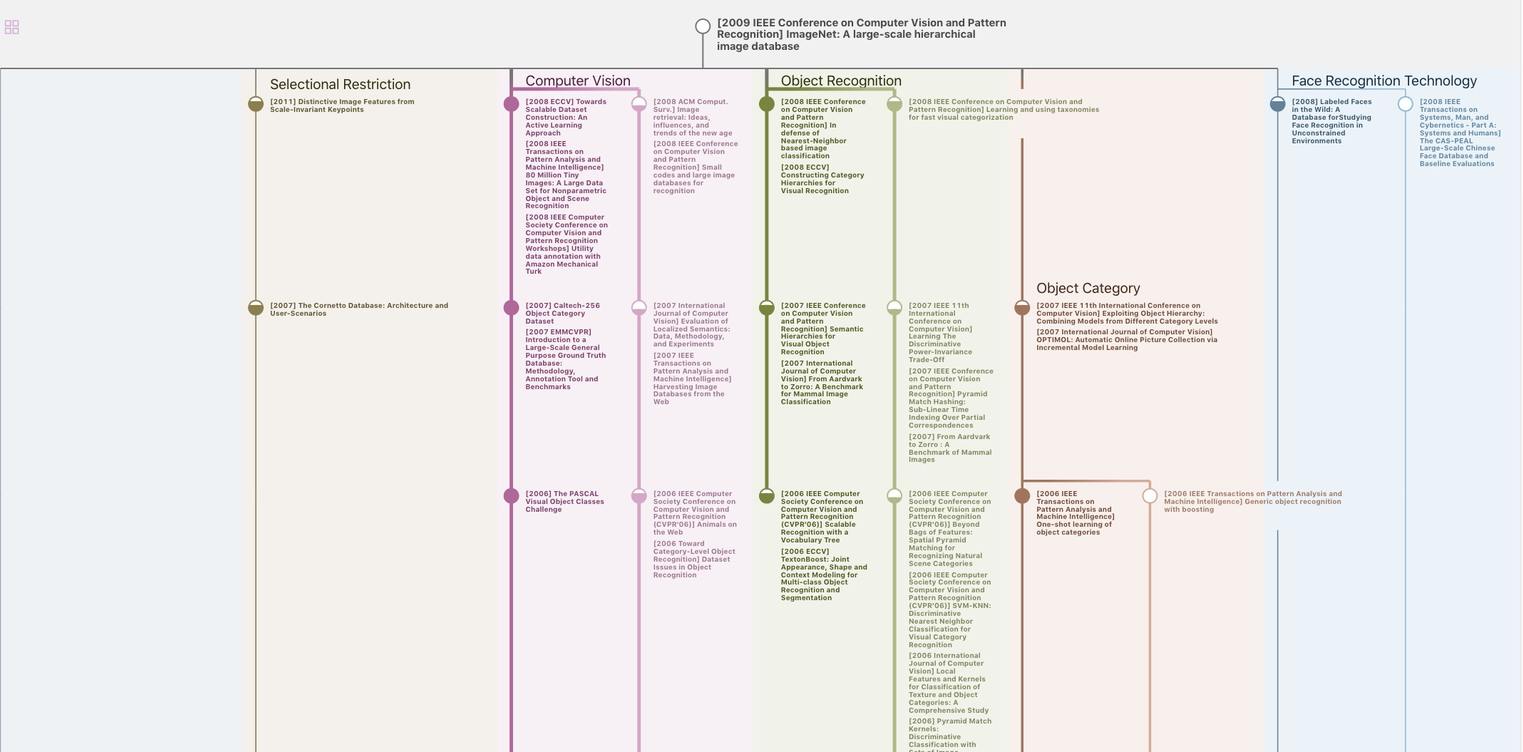
生成溯源树,研究论文发展脉络
Chat Paper
正在生成论文摘要