Bin Picking of Reflective Steel Parts Using a Dual-Resolution Convolutional Neural Network Trained in a Simulated Environment
2018 IEEE International Conference on Robotics and Biomimetics (ROBIO)(2018)
摘要
We consider the case of robotic bin picking of reflective steel parts, using a structured light 3D camera as a depth imaging device. In this paper, we present a new method for bin picking, based on a dual-resolution convolutional neural network trained entirely in a simulated environment. The dual-resolution network consists of a high resolution focus network to compute the grasp and a low resolution context network to avoid local collisions. The reflectivity of the steel parts result in depth images that have a lot of missing data. To take this into account, training of the neural net is done by domain randomization on a large set of synthetic depth images that simulate the missing data problems of the real depth images. We demonstrate both in simulation and in a real-world test that our method can perform bin picking of reflective steel parts.
更多查看译文
关键词
Three-dimensional displays,Robots,Grippers,Training,Steel,Convolutional neural networks
AI 理解论文
溯源树
样例
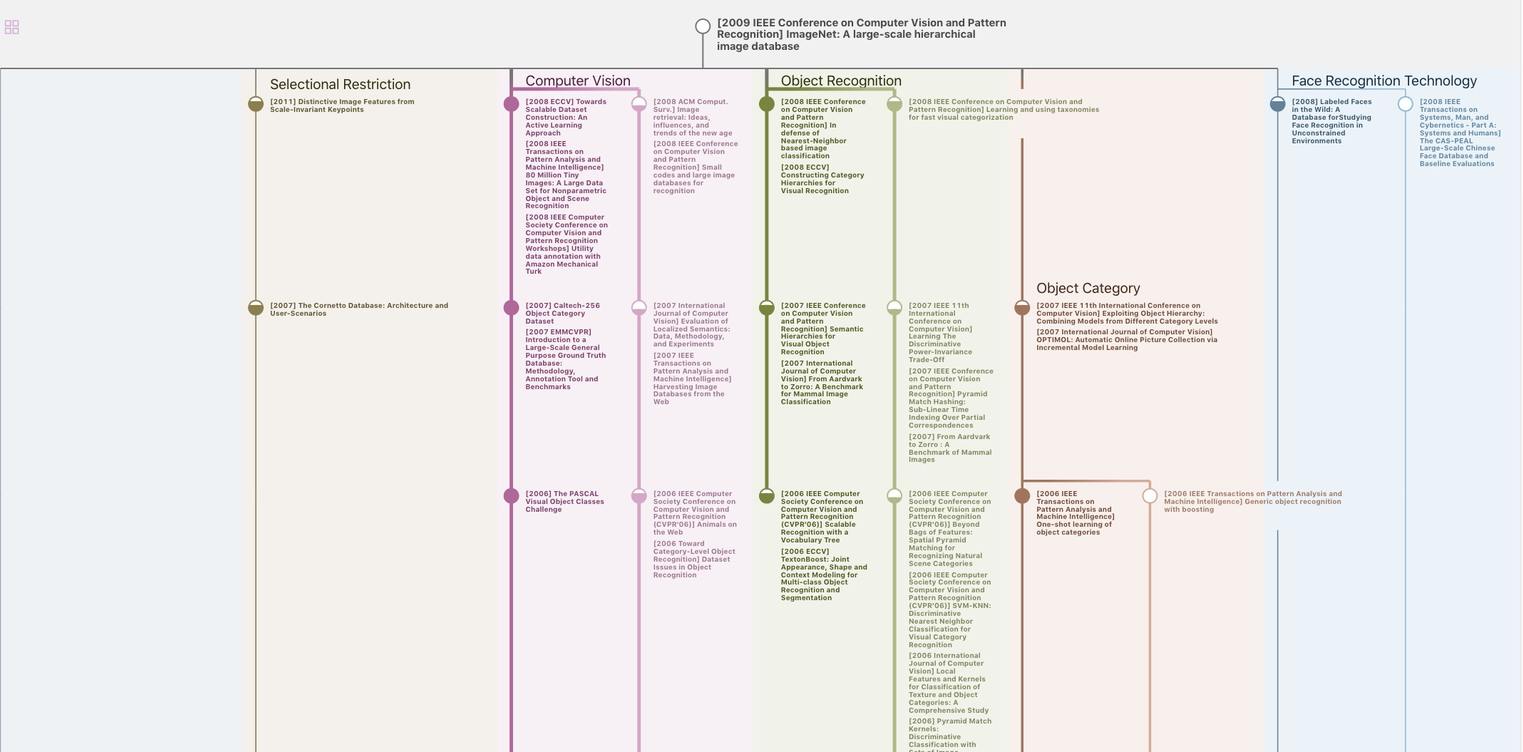
生成溯源树,研究论文发展脉络
Chat Paper
正在生成论文摘要