Trimmed categorical cross-entropy for deep learning with label noise
Electronics Letters(2019)
摘要
Deep learning methods are nowadays considered as state-of-the-art approach in many sophisticated problems, such as computer vision, speech understanding or natural language processing. However, their performance relies on the quality of large annotated datasets. If the data are not well-annotated and label noise occur, such data-driven models become less reliable. In this Letter, the authors present very simple way to make the training process robust to noisy labels. Without changing network architecture and learning algorithm, the authors apply modified error measure that improves network generalisation when trained with label noise. Preliminary results obtained for deep convolutional neural networks, trained with novel trimmed categorical cross-entropy loss function, revealed its improved robustness for several levels of label noise.
更多查看译文
关键词
entropy,learning (artificial intelligence),convolutional neural nets
AI 理解论文
溯源树
样例
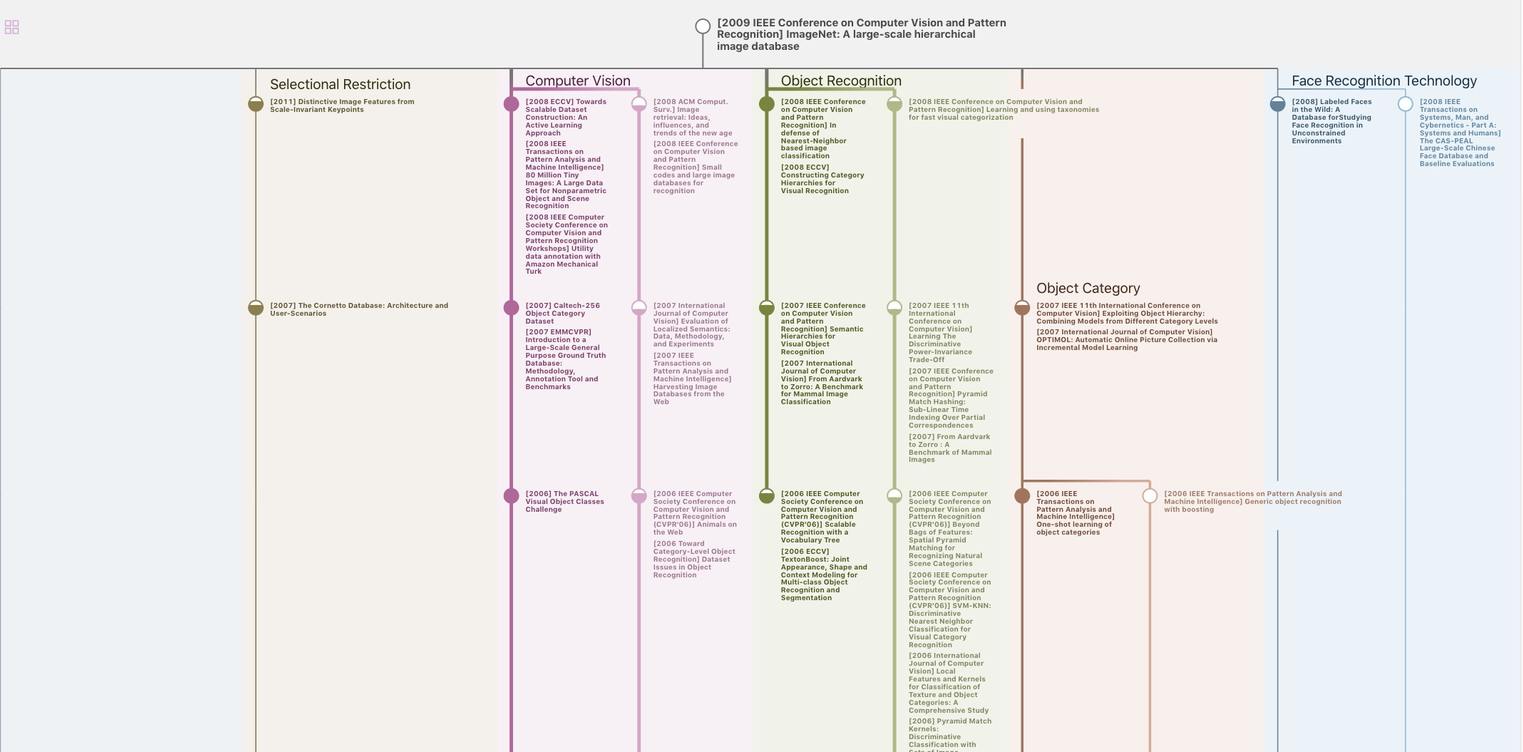
生成溯源树,研究论文发展脉络
Chat Paper
正在生成论文摘要