An Improved Multi-Scale Entropy Algorithm in Emotion EEG Features Extraction
JOURNAL OF MEDICAL IMAGING AND HEALTH INFORMATICS(2017)
摘要
The study on multi-scale entropy applied to extract emotional EEG features has been researched. Considering that the traditional feature extraction algorithm adopting multi-scale entropy may lose important information during the coarsening process, and that small-scale suffers from lack of significant feature while big-scale causes excessive computation, an improved extraction algorithm based on multi-scale entropy was put forward. The improved algorithm determines scales according to the number of intrinsic mode functions of the adaptive multi-scale entropy. At the same time, the EEG signal's small changes were highlighted through the binary-state processing of the EEG signal with the adaptive method, thus the characteristics of the EEG signal can be fully tapped, and the complexity of the algorithm can be reduced. Based on the optimized Support Vector Machine (SVM) classifier, the emotional EEG recognition was achieved by using the international DEAP database for emotion analysis, and the performance of the improved algorithm was compared with traditional algorithm's. The results indicate that the classification accuracy of the improved algorithm is 12.33% higher than that of the traditional multi-scale entropy algorithm and 7.27% higher than that of the adaptive multi-scale entropy algorithm, showing that the improved algorithm is effective in extracting EEG features.
更多查看译文
关键词
Emotional Electroencephalogram,Multi-Scale Entropy,Adaptive Multi-Scale Entropy,Improved Multi-Scale Entropy
AI 理解论文
溯源树
样例
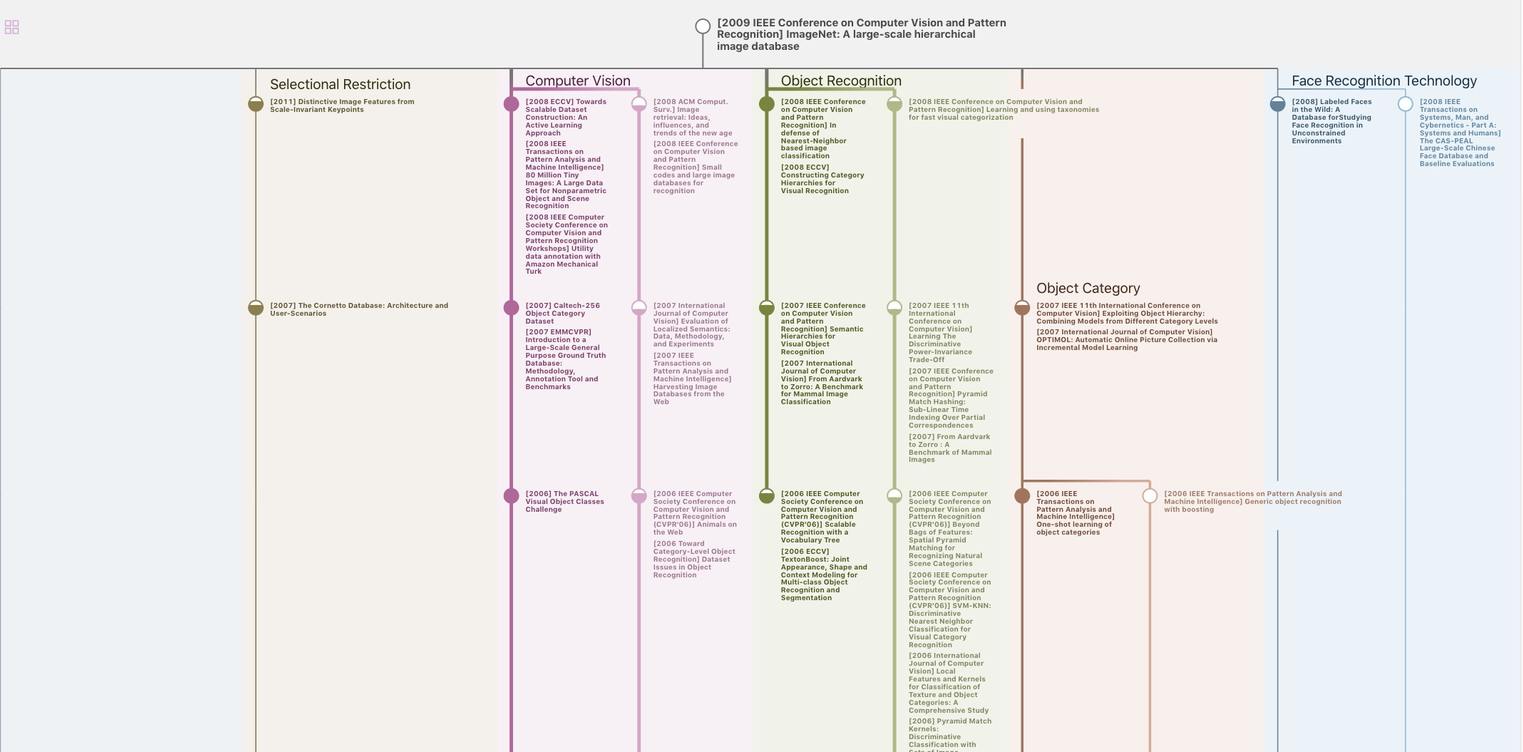
生成溯源树,研究论文发展脉络
Chat Paper
正在生成论文摘要