Convex-Constrained Sparse Additive Modeling And Its Extensions
CONFERENCE ON UNCERTAINTY IN ARTIFICIAL INTELLIGENCE (UAI2017)(2017)
摘要
Sparse additive modeling is a class of effective methods for performing high-dimensional nonparametric regression. In this work we show how shape constraints such as convexity/concavity and their extensions, can be integrated into additive models. The proposed sparse difference of convex additive models (SDCAM) can estimate most continuous functions without any a priori smoothness assumption. Motivated by a characterization of difference of convex functions, our method incorporates a natural regularization functional to avoid overfitting and to reduce model complexity. Computationally, we develop an efficient backfitting algorithm with linear periteration complexity. Experiments on both synthetic and real data confirm that our method is competitive against state-of-the-art sparse additive models, with improved performance in most scenarios.
更多查看译文
AI 理解论文
溯源树
样例
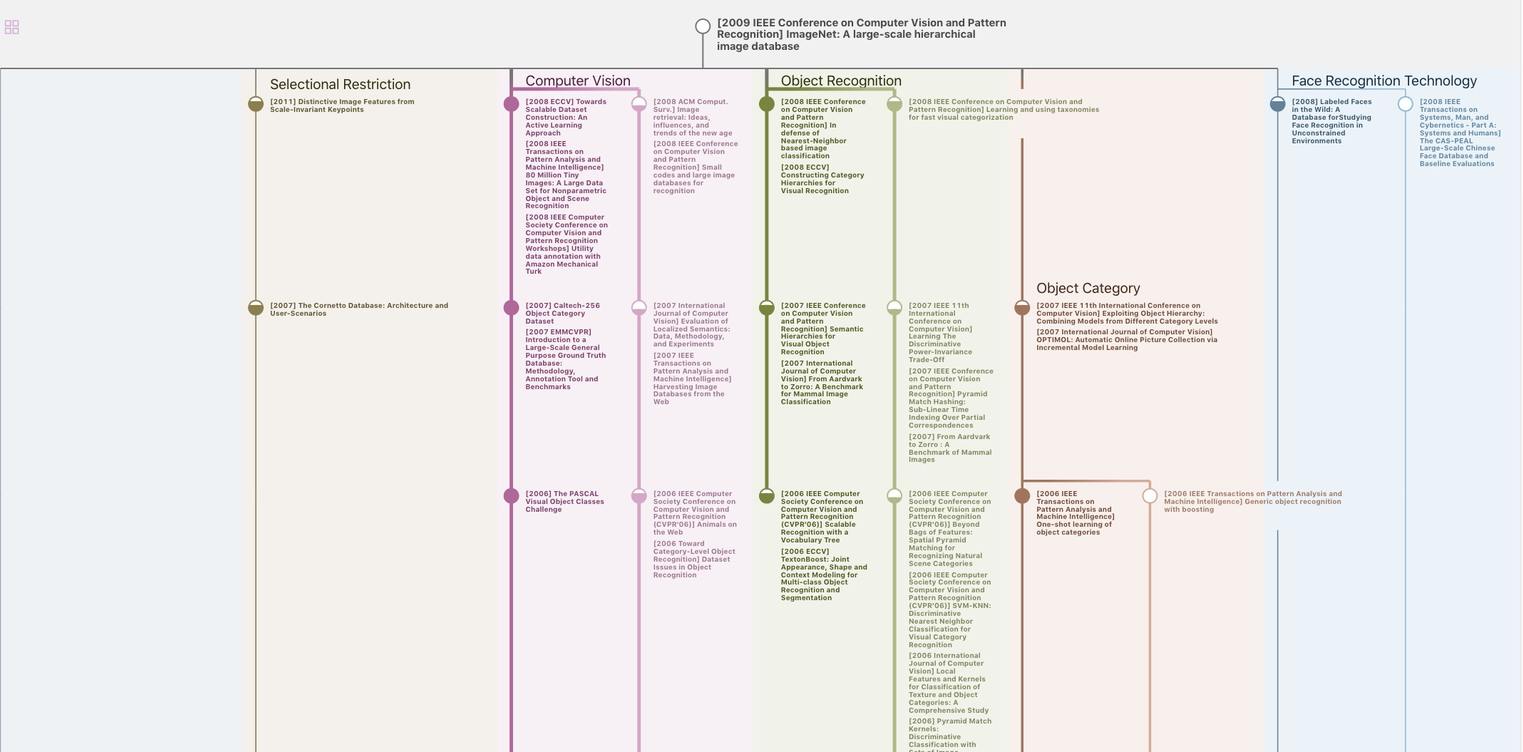
生成溯源树,研究论文发展脉络
Chat Paper
正在生成论文摘要