Autonomous Curiosity For Real-Time Training Onboard Robotic Agents
2019 IEEE WINTER CONFERENCE ON APPLICATIONS OF COMPUTER VISION (WACV)(2019)
摘要
Learning requires both study and curiosity. A good learner is not only good at extracting information from the data given to it, but also skilled at finding the right new information to learn from. This is especially true when a human operator is required to provide the ground truth-such a source should only be queried sparingly. In this work, we address the problem of curiosity as it relates to online, real-time, human-in-the-loop training of an object detection algorithm onboard a robotic platform, one where motion produces new views of the subject. We propose a deep reinforcement learning approach that decides when to ask the human user for ground truth, and when to move. Through a series of experiments, we demonstrate that our agent learns a movement and request policy that is at least 3x more effective at using human user interactions to train an object detector than untrained approaches, and is generalizable to a variety of subjects and environments.
更多查看译文
关键词
object detection algorithm,robotic platform,deep reinforcement learning approach,human user interactions,autonomous curiosity,real-time training onboard robotic agents,human operator,human-in-the-loop training
AI 理解论文
溯源树
样例
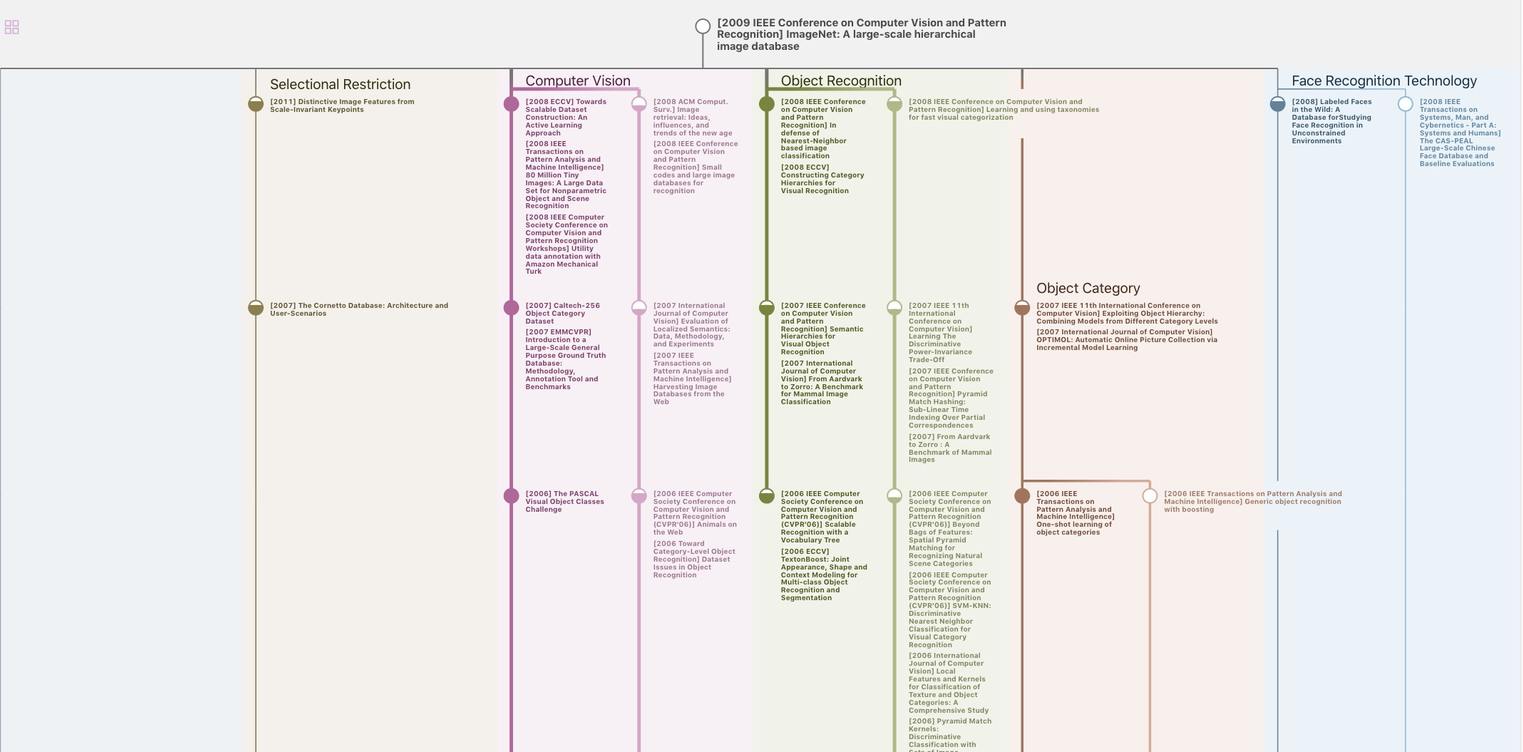
生成溯源树,研究论文发展脉络
Chat Paper
正在生成论文摘要