Aligned To The Object, Not To The Image: A Unified Pose-Aligned Representation For Fine-Grained Recognition
2019 IEEE WINTER CONFERENCE ON APPLICATIONS OF COMPUTER VISION (WACV)(2019)
摘要
Dramatic appearance variation due to pose constitutes a great challenge in fine-grained recognition, one which recent methods using attention mechanisms or second-order statistics fail to adequately address. Modern CNNs typically lack an explicit understanding of object pose and are instead confused by entangled pose and appearance. In this paper, we propose a unified object representation built from pose-aligned regions of varied spatial sizes. Rather than representing an object by regions aligned to image axes, the proposed representation characterizes appearance relative to the object's pose using pose-aligned patches whose features are robust to variations in pose, scale and viewing angle. We propose an algorithm that performs pose estimation and forms the unified object representation as the concatenation of pose-aligned region features, which is then fed into a classification network. The proposed algorithm attains state-of-the-art results on two fine-grained datasets, notably 89.2% on the widely-used CUB-200 [46] dataset and 87.9% on the much larger NABirds [45] dataset. Our success relative to competing methods shows the critical importance of disentangling pose and appearance for continued progress in fine-grained recognition.
更多查看译文
关键词
fine-grained datasets,fine-grained recognition,unified pose-aligned representation,object pose,unified object representation,pose-aligned regions,varied spatial sizes,image axes,viewing angle,pose-aligned region features,classification network,CUB-200 dataset,NABirds dataset,CNN
AI 理解论文
溯源树
样例
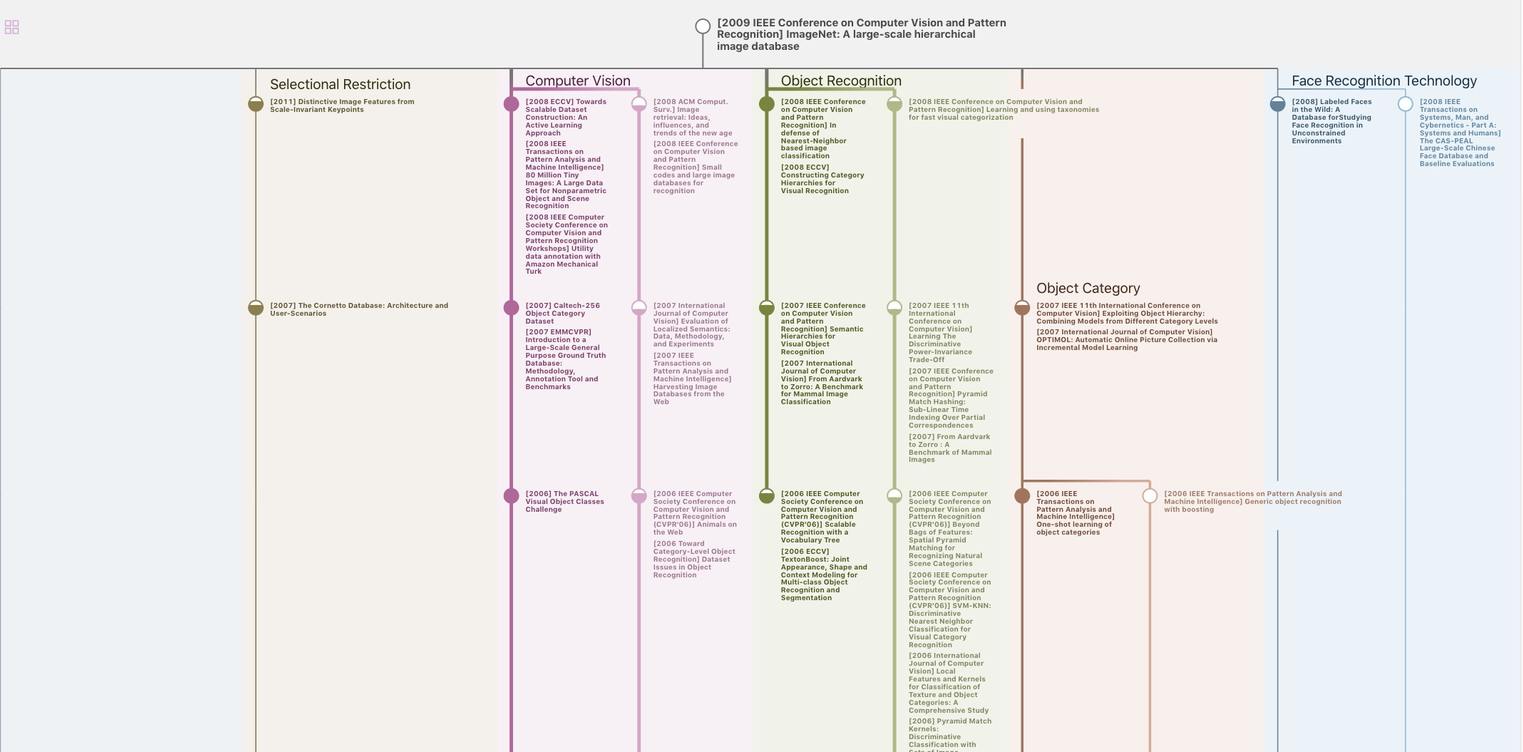
生成溯源树,研究论文发展脉络
Chat Paper
正在生成论文摘要