Activity classification for real-time wearable systems: Effect of window length, sampling frequency and number of features on classifier performance
2016 IEEE EMBS Conference on Biomedical Engineering and Sciences (IECBES)(2016)
摘要
The aim of this study was to evaluate how the physical activity classification window length, accelerometer sampling frequency and the number of correlating features affect the classifier performance. It is important to study the effect of these elements in order to reduce the computational power and memory buffers needed for wearable systems, where classification is done in real-time. Three different window lengths (5 s, 3 s, 1s), sampling frequencies (50 Hz, 25 Hz, 13 Hz) and two feature sets (110 and 43 features) were tested and evaluated in this study. As a result, it was found that the classifier performed similarly with the window lengths of 5 s and 3 s and the sampling rates of 50 Hz and 25 Hz, but the results with the window length of 1 s or the sampling rate of 13 Hz were lower. No noticeable difference in classifier sensitivity was found by decreasing the number of features based on correlation.
更多查看译文
关键词
physical activity classification,sampling frequency,window length,classification features,activity trackers,wearable systems
AI 理解论文
溯源树
样例
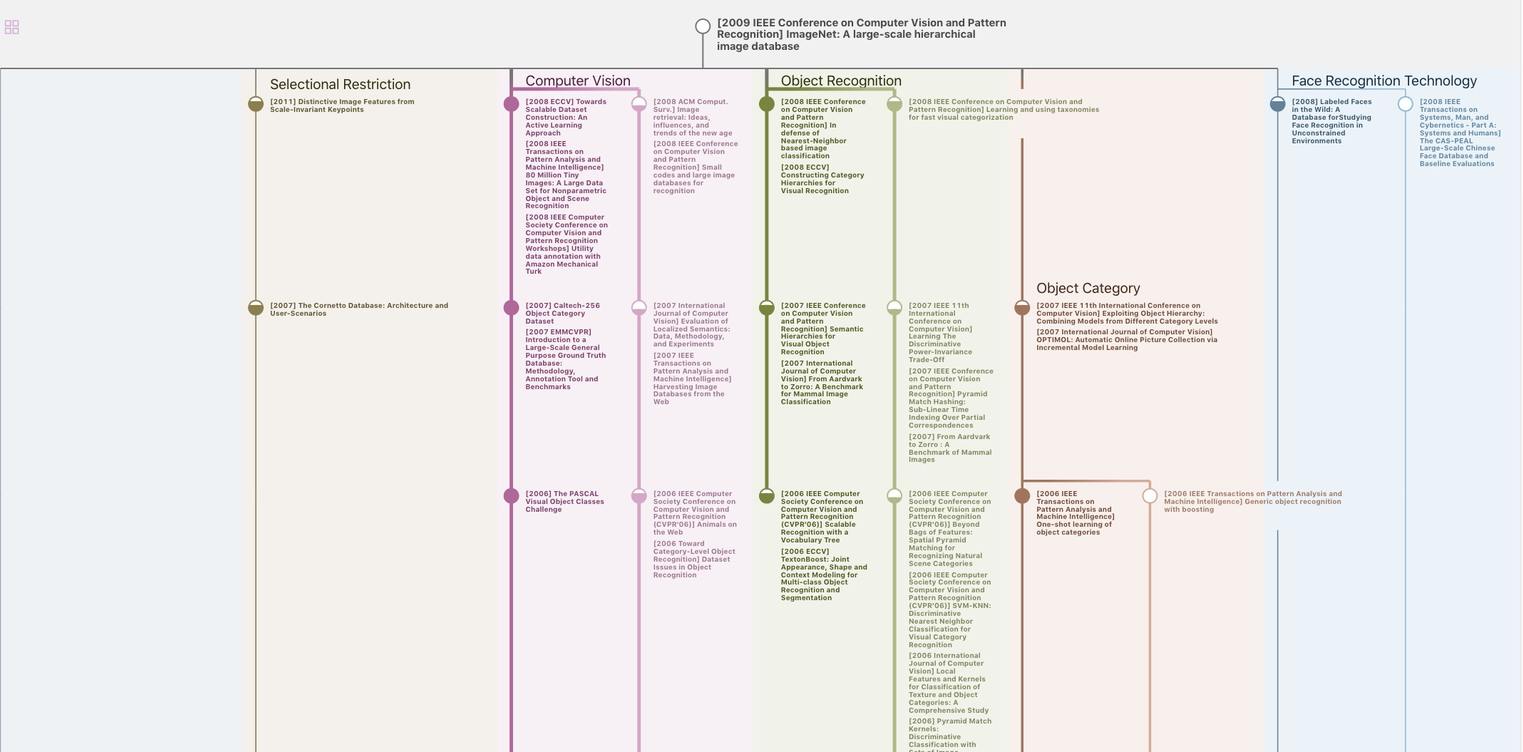
生成溯源树,研究论文发展脉络
Chat Paper
正在生成论文摘要