Improving Deep Belief Networks via Delta Rule for Sentiment Classification
2016 IEEE 28th International Conference on Tools with Artificial Intelligence (ICTAI)(2016)
摘要
Sentiment classification has received much attention in both engineering and academic fields. Deep belief networks (DBN) has proved powerful in many domains including natural language processing. In this paper, DBN is applied in sentiment classification, while we propose a new way to improve the DBN based on the unsupervised training phase of restricted Boltzmann machines (RBM). That is, the RBM generates the hidden layer in an unsupervised fashion, and then we use this hidden layer as the output of a single-layer neural network, which is trained using the delta rule. The new weights trained from delta rule are then transmitted into the whole back propagation. This way keeps much more correction signal information for each layer in back propagation compared to that in the same network structure. Consequently, our experimental results demonstrate that the new learning method performs relatively better on ten sentiment datasets, which further proves the delta rule improves DBN performance for natural language processing tasks.
更多查看译文
关键词
Deep belief networks,Restricted Boltzmann machines,Single-layer network,Delta rule,Sentiment classification
AI 理解论文
溯源树
样例
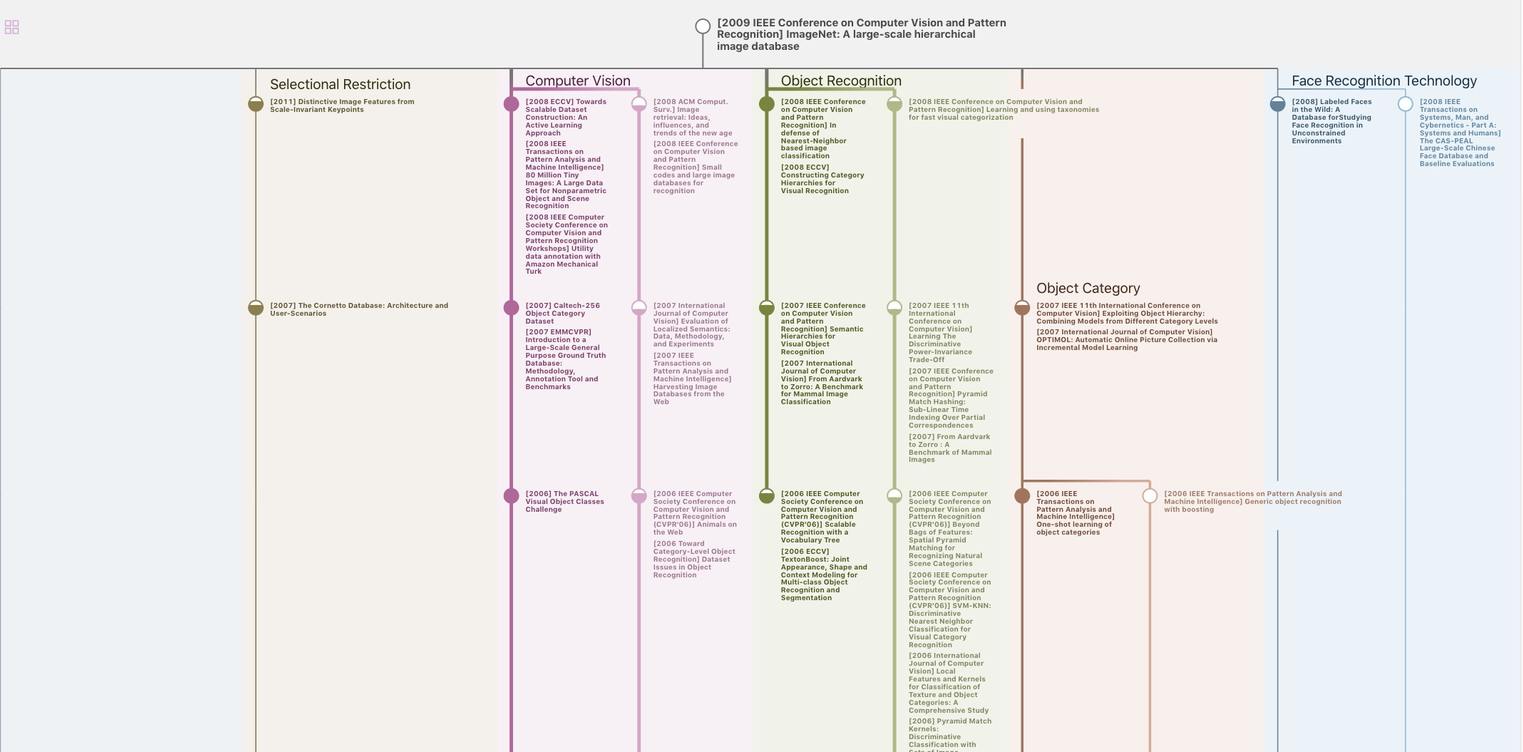
生成溯源树,研究论文发展脉络
Chat Paper
正在生成论文摘要