Applicability of the Spoken Knowledge in Low Literacy Patients with Diabetes in Brazilian elderly.
EINSTEIN-SAO PAULO(2016)
Abstract
Objective: To translate, adapt and evaluate the properties of a Brazilian Portuguese version of the Spoken Knowledge in Low Literacy Patients with Diabetes, which is a questionnaire that evaluate diabetes knowledge. Methods: A cross-sectional study with type 2 diabetes patients aged >= 60 years, seen at a public healthcare organization in the city of Sao Paulo (SP). After the development of the Portuguese version, we evaluated the psychometrics properties and the association with sociodemographic and clinical variables. The regression models were adjusted for sociodemographic data, functional health literacy, duration of disease, use of insulin, and glycemic control. Results: We evaluated 129 type 2 diabetic patients, with mean age of 75.9 (+/- 6.2) years, mean scholling of 5.2 (+/- 4.4) years, mean glycosylated hemoglobin of 7.2% (+/- 1.4), and mean score on Spoken Knowledge in Low Literacy Patients with Diabetes of 42.1% (+/- 25.8). In the regression model, the variables independently associated to Spoken Knowledge in Low Literacy Patients with Diabetes were schooling (B= 0.193; p= 0.003), use of insulin (B= 1.326; p= 0.004), duration of diabetes (B= 0.053; p= 0.022) and health literacy (B= 0.108; p= 0.021). The determination coefficient was 0.273. The Cronbach alpha was 0.75, demonstrating appropriate internal consistency. Conclusion: This translated version of the Spoken Knowledge in Low Literacy Patients with Diabetes showed to be adequate to evaluate diabetes knowledge in elderly patients with low schooling levels. It presented normal distribution, adequate internal consistency, with no ceiling or floor effect. The tool is easy to be used, can be quickly applied and does not depend on reading skills.
MoreTranslated text
Key words
Diabetes mellitus,Blood glucose,Survey and questionnaires,Health knowledge, attitudes, practice,Aged
AI Read Science
Must-Reading Tree
Example
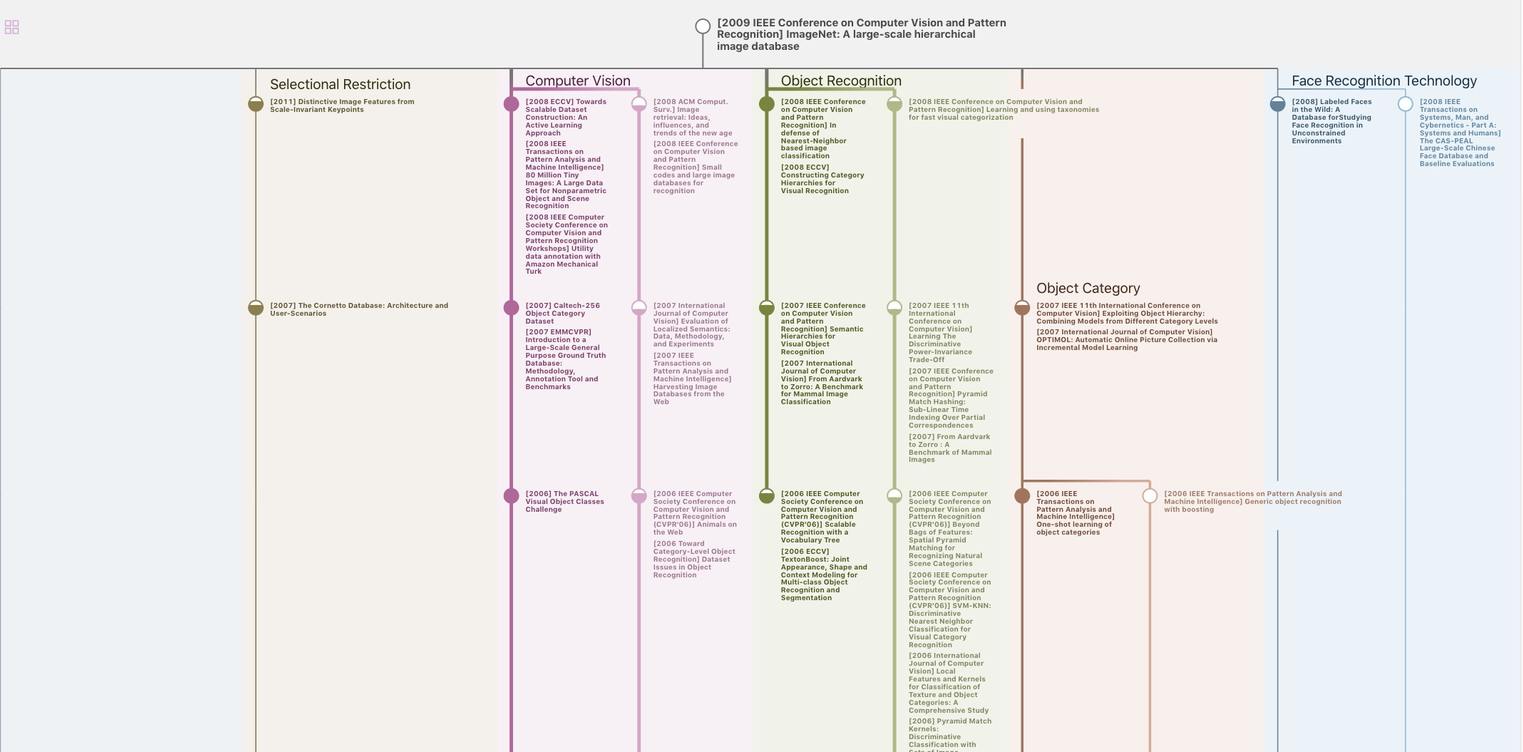
Generate MRT to find the research sequence of this paper
Chat Paper
Summary is being generated by the instructions you defined