Overcoming Catastrophic Forgetting In Neural Networks
PROCEEDINGS OF THE NATIONAL ACADEMY OF SCIENCES OF THE UNITED STATES OF AMERICA(2017)
摘要
The ability to learn tasks in a sequential fashion is crucial to the development of artificial intelligence. Until now neural networks have not been capable of this and it has been widely thought that catastrophic forgetting is an inevitable feature of connectionist models. We show that it is possible to overcome this limitation and train networks that can maintain expertise on tasks that they have not experienced for a long time. Our approach remembers old tasks by selectively slowing down learning on the weights important for those tasks. We demonstrate our approach is scalable and effective by solving a set of classification tasks based on a hand-written digit dataset and by learning several Atari 2600 games sequentially.
更多查看译文
关键词
synaptic consolidation, artificial intelligence, stability plasticity, continual learning, deep learning
AI 理解论文
溯源树
样例
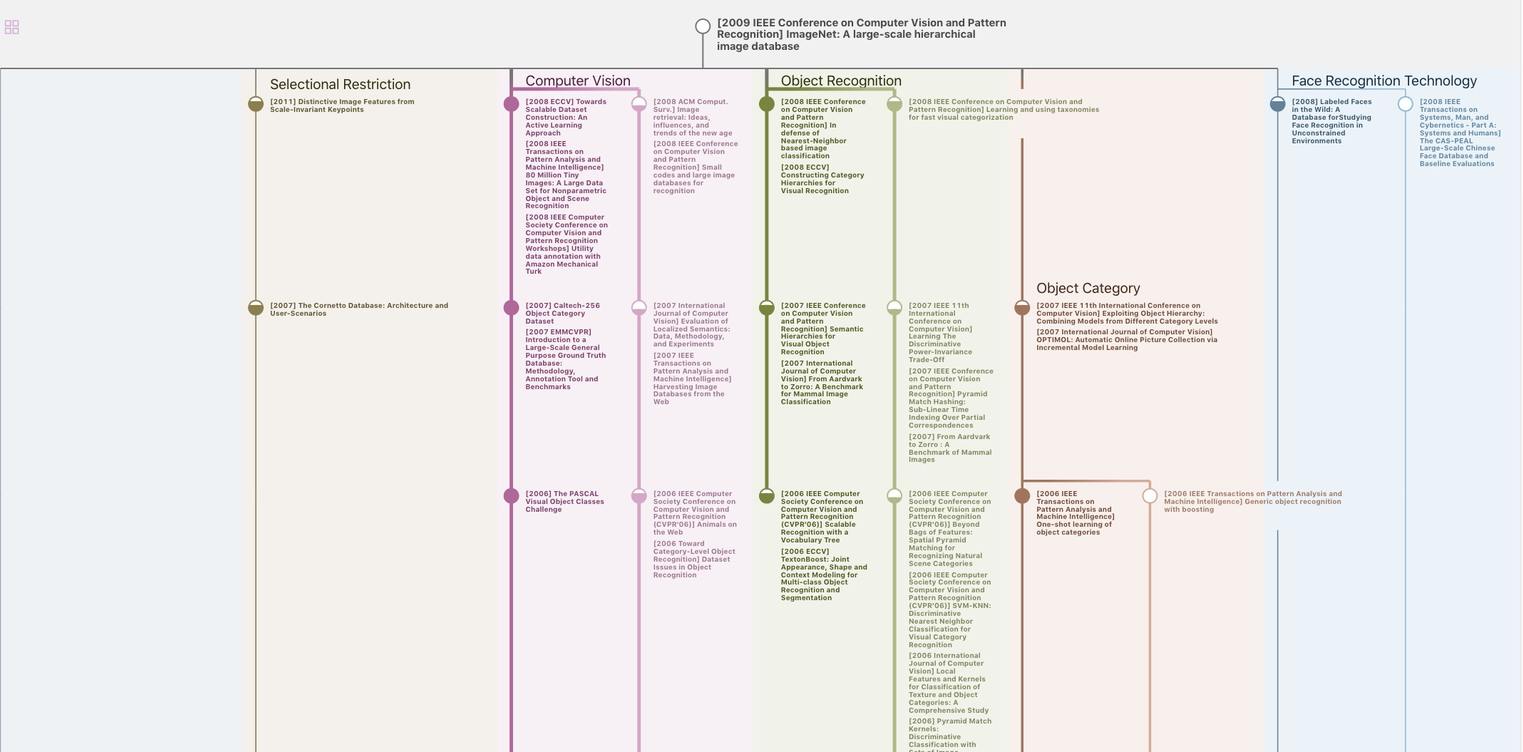
生成溯源树,研究论文发展脉络
Chat Paper
正在生成论文摘要