A New Distance Measure for Non-Identical Data with Application to Image Classification.
Pattern Recognition(2017)
Abstract
Distance measures are part and parcel of many computer vision algorithms. The underlying assumption in all existing distance measures is that feature elements are independent and identically distributed. However, in real-world settings, data generally originate from heterogeneous sources even if they do possess a common data-generating mechanism. Since these sources are not identically distributed by necessity, the assumption of identical distribution is inappropriate. Here, we use statistical analysis to show that feature elements of local image descriptors are indeed non-identically distributed. To test the effect of omitting the unified distribution assumption, we created a new distance measure called the Poisson-Binomial radius (PBR). PBR is a bin-to-bin distance which accounts for the dispersion of bin-to-bin information. PBR's performance was evaluated on twelve benchmark data sets covering six different classification and recognition applications: texture, material, leaf, scene, ear biometrics and category-level image classification. Results from these experiments demonstrate that PBR outperforms state-of-the-art distance measures for most of the data sets and achieves comparable performance on the rest, suggesting that accounting for different distributions in distance measures can improve performance in classification and recognition tasks.
MoreTranslated text
Key words
Poisson-Binomial distribution,Semi-metric distance,Non-identical data,Distance measure,Image classification,Image recognition
AI Read Science
Must-Reading Tree
Example
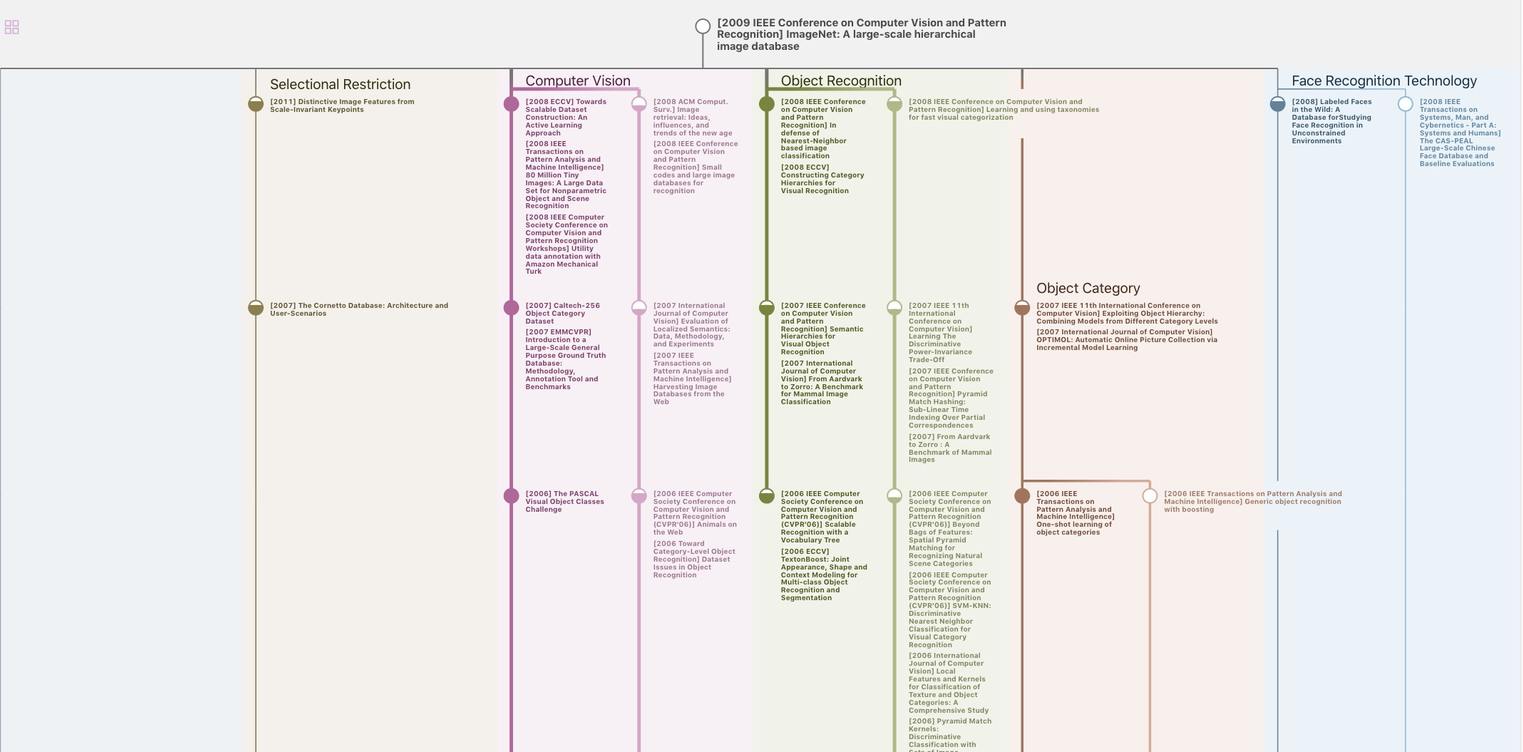
Generate MRT to find the research sequence of this paper
Chat Paper
Summary is being generated by the instructions you defined