Adaptive Sequential Stochastic Optimization
IEEE Trans. Automat. Contr.(2016)
摘要
A framework is introduced for sequentially solving convex stochastic minimization problems, where the objective functions change slowly, in the sense that the distance between successive minimizers is bounded. The minimization problems are solved by sequentially applying a selected optimization algorithm, such as stochastic gradient descent (SGD), based on drawing a number of samples in order to carry the iterations. Two tracking criteria are introduced to evaluate approximate minimizer quality: one based on being accurate with respect to the mean trajectory, and the other based on being accurate in high probability (IHP). An estimate of a bound on the minimizers' change, combined with properties of the chosen optimization algorithm, is used to select the number of samples needed to meet the desired tracking criterion. A technique to estimate the change in minimizers is provided along with analysis to show that eventually the estimate upper bounds the change in minimizers. This estimate of the change in minimizers provides sample size selection rules that guarantee that the tracking criterion is met for sufficiently large number of time steps. Simulations are used to confirm that the estimation approach provides the desired tracking accuracy in practice, while being efficient in terms of number of samples used in each time step.
更多查看译文
关键词
Optimization,Linear programming,Convex functions,Bayes methods,Minimization,Approximation algorithms,Upper bound
AI 理解论文
溯源树
样例
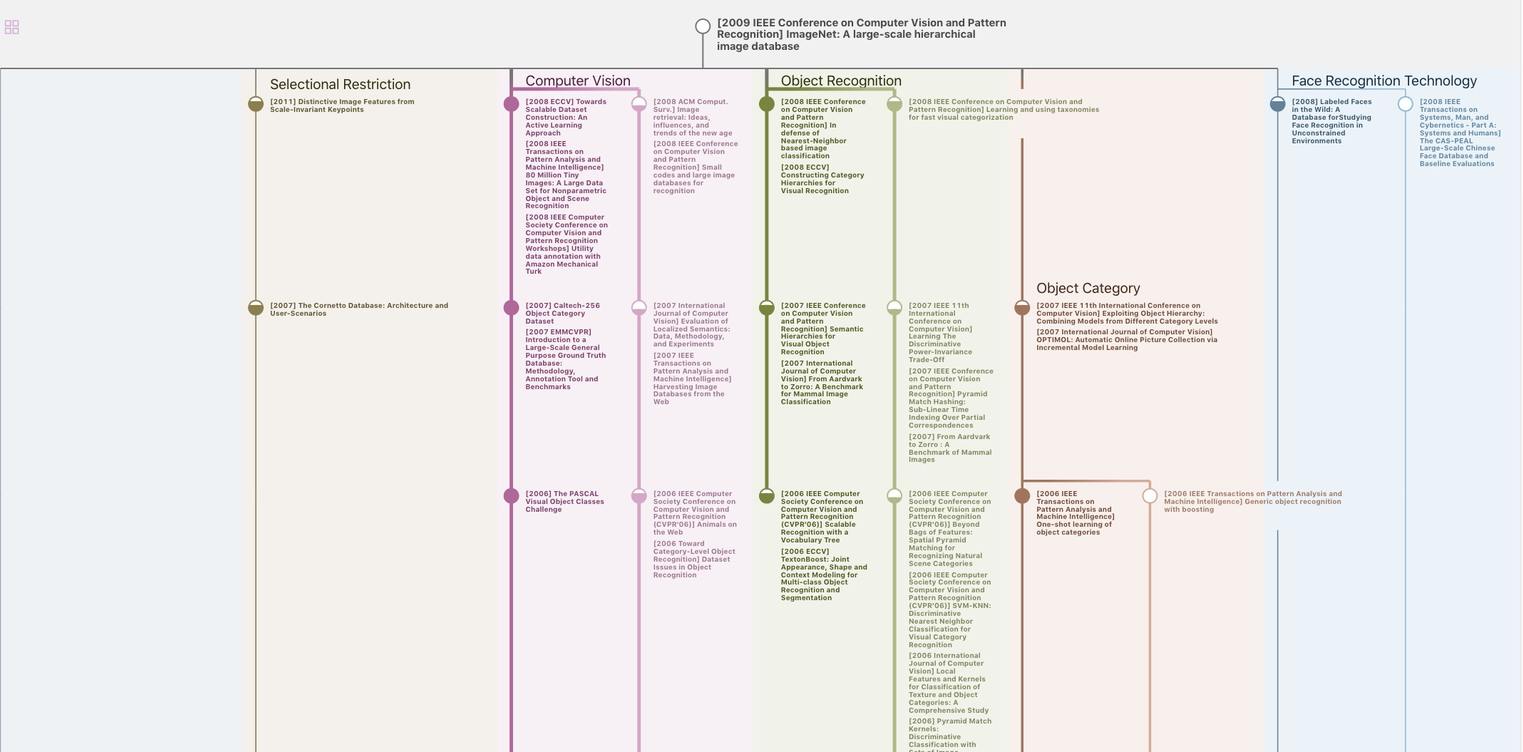
生成溯源树,研究论文发展脉络
Chat Paper
正在生成论文摘要