Transient Classification in LIGO data using Difference Boosting Neural Network
PHYSICAL REVIEW D(2017)
摘要
Detection and classification of transients in data from gravitational wave detectors are crucial for efficient searches for true astrophysical events and identification of noise sources. We present a hybrid method for classification of short duration transients seen in gravitational wave data using both supervised and unsupervised machine learning techniques. To train the classifiers, we use the relative wavelet energy and the corresponding entropy obtained by applying one-dimensional wavelet decomposition on the data. The prediction accuracy of the trained classifier on nine simulated classes of gravitational wave transients and also LIGO's sixth science run hardware injections are reported. Targeted searches for a couple of known classes of nonastrophysical signals in the first observational run of Advanced LIGO data are also presented. The ability to accurately identify transient classes using minimal training samples makes the proposed method a useful tool for LIGO detector characterization as well as searches for short duration gravitational wave signals.
更多查看译文
关键词
transient classification,ligo data,neural network
AI 理解论文
溯源树
样例
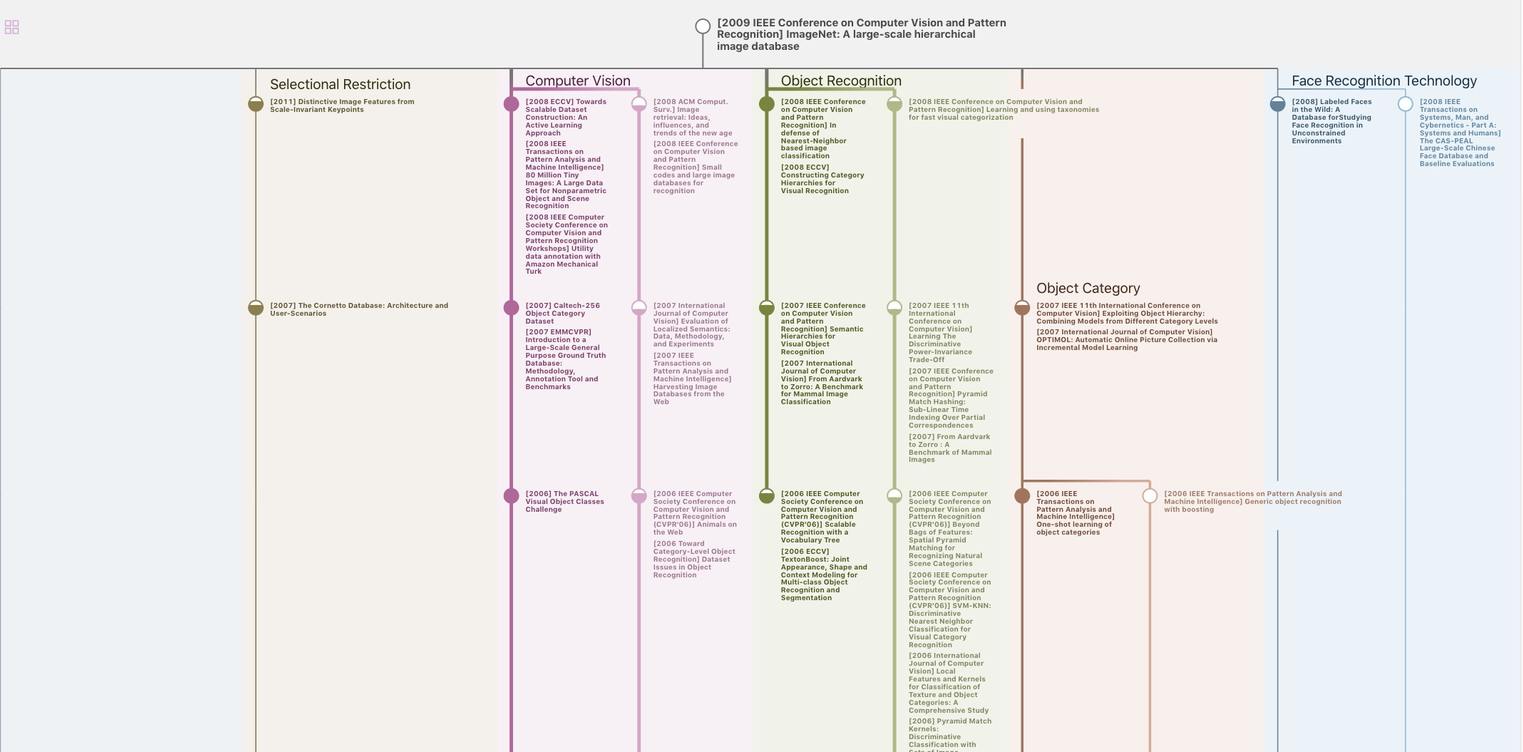
生成溯源树,研究论文发展脉络
Chat Paper
正在生成论文摘要