Augmenting a Clinical Study With Virtual Patient Models: Food and Drug Administration and Industry Collaboration
JOURNAL OF MEDICAL DEVICES-TRANSACTIONS OF THE ASME(2016)
摘要
Medical device manufacturers are increasingly using predictive computer models during the product development process. These models are created for a specific context of use in order to simulate the device and relevant anatomy and physiology associated with predicting safety and efficacy outcomes. Since these models are created to predict the same endpoints that would be observed in clinical practice with the same population variability, we use the term virtual patient models to refer to these simulations. These virtual patient models can be incorporated into a study in way that is analogous to how some Bayesian clinical trials incorporate historical data as prior information.To properly inform clinical evaluation, the virtual patient model must:The construction of a virtual patient model will be different for each particular outcome and may involve a variety of disciplines. Applications that lend themselves to virtual patient models will involve local mechanisms that are well understood. The potential examples include closed-loop glucose control, heart valve frame or stent fracture, orthopedic implant wear or fracture, or cardiac lead fracture.The inputs for the model can come from a variety of sources including animal and human studies, finite-element analysis, and bench testing. Regardless of the origin, each data set needs to be considered statistically in order to represent variability seen across devices and patients. Additionally, the model output needs to reflect a clinically measurable factor (e.g., pacing threshold and lead fracture) as opposed to a traditional engineering metric, such as temperature, stress, or strain.The Medical Device Innovation Consortium (MDIC) working group on Clinical Trials Informed by Bench and Simulation includes members from the industry and the U.S. Food and Drug Administration (FDA). The working group has used a mock investigational device exemption (IDE) submission as a means to demonstrate the statistical considerations and choices associated with using this framework for a clinical trial designed with virtual patients. This approach has provided a mutually beneficial way for the medical device and regulatory community to identify the issues that should be addressed when designing this type of trial.The working group has identified fatigue fracture in a hypothetical new implantable cardioverter defibrillator (ICD) lead (Model 2014VP) as a vehicle for a specific example. The ICD lead fracture is a good candidate for virtual patient modeling because it is a failure mode with direct clinical implications (e.g., loss of therapy), and there are examples of analysis methods in the literature [1].The working group developed a hypothetical new ICD lead, called the Model 2014Vp. The Model 2014VP has design changes that are expected to improve the handling characteristics of the lead, but could also affect the fatigue performance.Fatigue performance in the Model 2014VP lead was modeled by incorporating bench fatigue strength with predicate lead in vivo use conditions, similar to Ref. [1]. For demonstration purposes, we only considered the case of fatigue fracture inside the heart, where cycle accumulation is solely due to heart motion. An actual implementation of this approach would consider all the failure modes that could contribute to clinical performance. The basic algorithm is a Monte Carlo simulation, as follows:Inputs for the lead fracture model were based on a draft ISO standard for cardiac lead fracture evaluation, currently under review by the AAMI Transvenous Leads Working Group. This methodology uses in vivo use conditions from human clinical study of predicate leads along with the in vitro bench test measurements of the candidate lead to produce a statistical reliability projection, illustrated in Fig. 1.The virtual patients were implemented as prior knowledge in a Bayesian clinical trial. We refer to this as an augmented trial. The statistical framework leverages FDA guidance for the use of Bayesian statistics in medical device clinical trials [2]. This approach can have benefits of decreased sample size and trial length while minimizing impact to study endpoints, type I error, and statistical power (type II error).There are two elements that may be novel to the statistician implementing or reviewing this method, discussed briefly below.The loss function developed by the working group is the Weibull cumulative distribution function, using a Bayesian p-value as an input. When the clinical and virtual data are highly similar, the p-value approaches 1 and the full amount of virtual patients can be incorporated. When the clinical data diverge from the virtual data, the p-value approaches zero and the number of virtual patients also approaches zero. The Weibull parameters control the relationship between p-value and fraction of virtual patients allowed. The loss function parameters and the maximum number of virtual patients allowed in the study are items to be agreed upon prior to starting the study. Candidate loss functions are shown in Fig. 2.Due to the novelty of this methodology, and the mutual interest of both FDA and industry in advancing the use of modeling in the medical device development process, a mock IDE submission was proposed as a means to evaluate and document the process. The sponsor team consisted of members representing the medical device manufacturers, engineering software, MDIC, and FDA. The team followed the standard process for presubmission meetings [3]. A review team was formed at FDA, consisting of members representing engineering, statistical, and medical functions, as if the submission was an actual product.The virtual patient model was simulated in a hypothetical clinical study with a failure rate endpoint of 5% at 2 yr. The number of real patients and the maximum number of virtual patients were both set to 100. We implemented the procedure described above and compared various loss function scenarios, including no loss function, for an augmented virtual patient trial to the traditional trial scenario.Including virtual patients in the clinical trial resulted in an increase in both statistical power (chance of correctly passing the trial when the true failure rate is less than 5%) and type I error (chance of incorrectly passing the trial when the failure rate is larger than 5%). The increased type I error was mitigated by the loss function, with only moderate decrease in power.The MDIC mock submission working group has had two presubmission meetings with the FDA review team at the time of this writing, with one more presubmission meeting and a public workshop planned in 2016. Although the mock submission process is not yet complete and will be documented in future publications, there were several themes emerging from the interactive discussions that can be immediately useful to both the medical device and regulatory communities:In cases where engineering models of clinical outcomes exist, the methodology summarized here provides a means for improving the clinical trial efficiency while protecting safety and efficacy endpoints. Additionally, the augmented clinical trial provides a natural means for additional model validation as well as a framework for ongoing postmarket study.
更多查看译文
关键词
virtual patient models,clinical study,drug administration
AI 理解论文
溯源树
样例
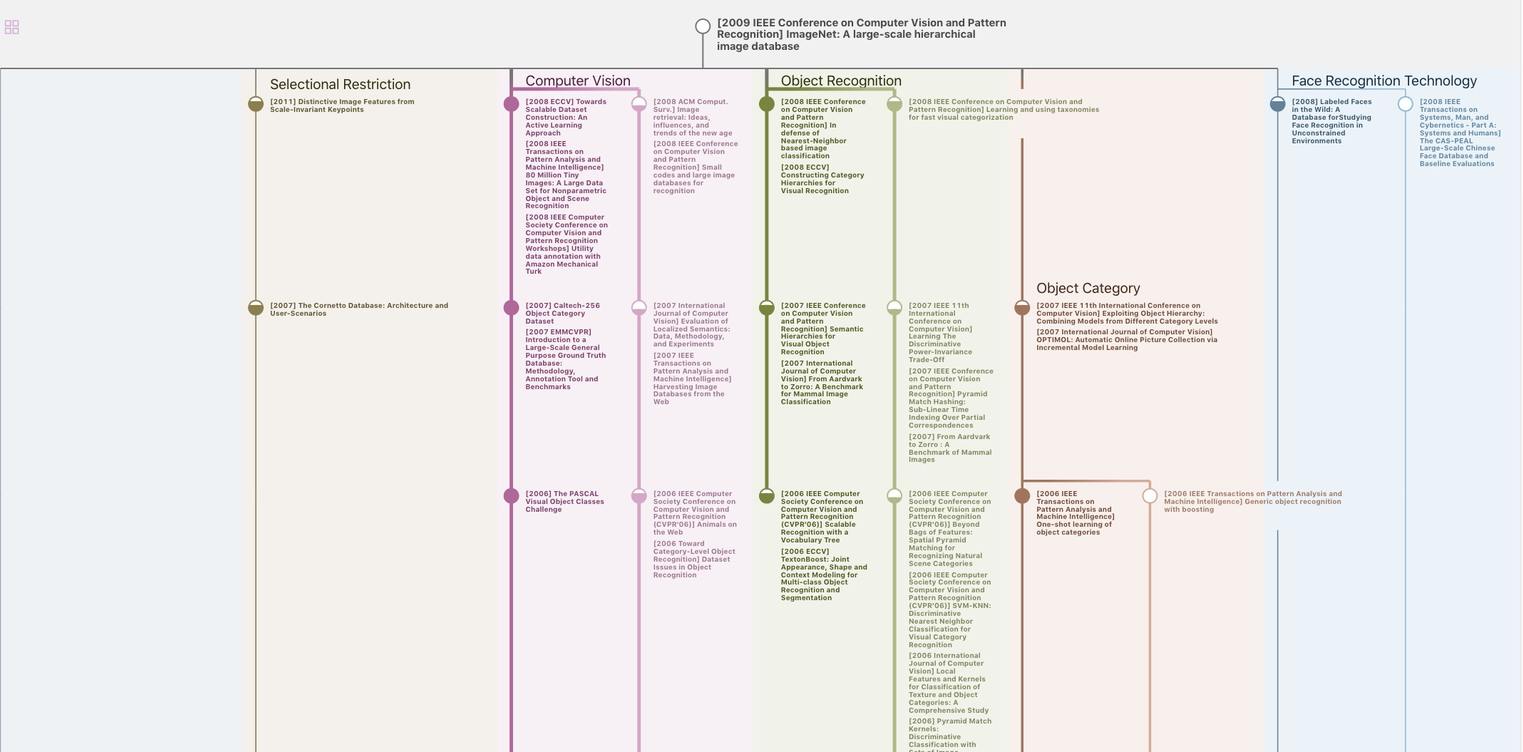
生成溯源树,研究论文发展脉络
Chat Paper
正在生成论文摘要