EEG representation using multi-instance framework on the manifold of symmetric positive definite matrices.
JOURNAL OF NEURAL ENGINEERING(2019)
摘要
Objective. The generalization and robustness of an electroencephalogram (EEG)-based system are crucial requirements in actual practices. Approach. To reach these goals, we propose a new EEG representation that provides a more realistic view of brain functionality by applying multi-instance (MI) framework to consider the non-stationarity of the EEG signal. In this representation, the non-stationarity of EEG is considered by describing the signal as a bag of relevant and irrelevant concepts. The concepts are provided by a robust representation of homogeneous segments of EEG signal using spatial covariance matrices. Due to the nonlinear geometry of the space of covariance matrices, we determine the boundaries of the homogeneous segments based on adaptive segmentation of the signal in a Riemannian framework. Each subject is described as a bag of covariance matrices of homogeneous segments and the bag-level discriminative information is used for classification. Main results. To evaluate the performance of the proposed approach, we examine it in a cultural neuroscience application for classification Iranian versus Swiss normal subjects to discover if strongly differing cultures can result in distinguishing patterns in brain electrical activity of the subjects. To confirm the effectiveness of the proposed representation, we also evaluate the proposed representation in EEG-based mental disorder diagnosis application for attention deficit hyperactivity disorder (ADHD)/bipolar mood disorder (BMD), Schizophrenia/ normal, and Major Depression Disorder/normal diagnosis applications. Significance. Experimental results confirm the superiority of the proposed approach, which is gained due to the robustness of covariance descriptor, the effectiveness of Riemannian geometry, the benefits of considering the inherent non-stationary nature of the brain by applying bag-level discriminative information, and automatic handling the artifacts.
更多查看译文
关键词
EEG,non-stationary,multi-instance framework
AI 理解论文
溯源树
样例
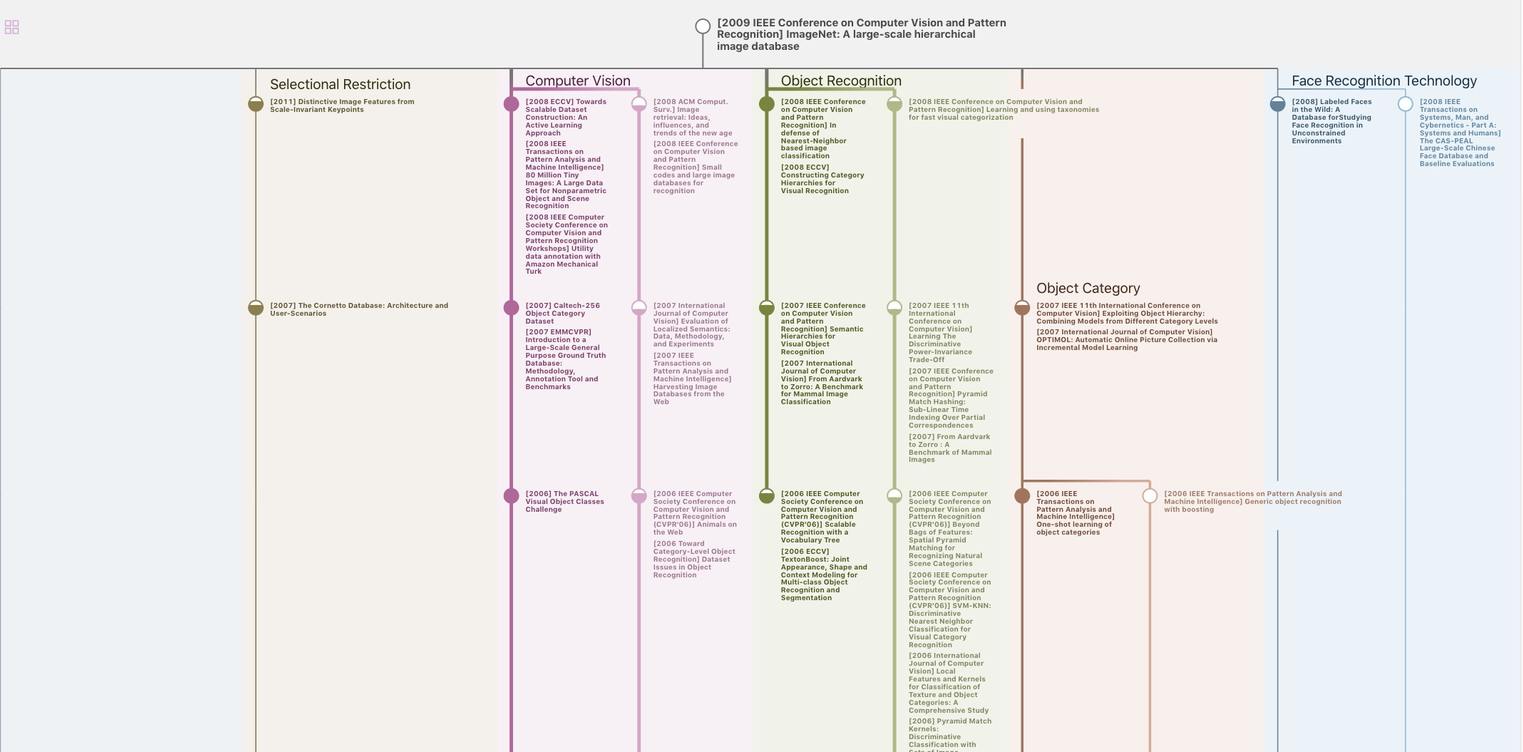
生成溯源树,研究论文发展脉络
Chat Paper
正在生成论文摘要