Convergence of the augmented decomposition algorithm
Comp. Opt. and Appl.(2018)
摘要
We study the convergence of the augmented decomposition algorithm (ADA) proposed in Rockafellar et al. (Problem decomposition in block-separable convex optimization: ideas old and new, https://www.washington.edu/ , 2017 ) for solving multi-block separable convex minimization problems subject to linear constraints. We show that the global convergence rate of the exact ADA is o(1/ν ) under the assumption that there exists a saddle point. We consider the inexact augmented decomposition algorithm and establish global and local convergence results under some mild assumptions, by providing a stability result for the maximal monotone operator 𝒯 associated with the perturbation from both primal and dual perspectives. This result implies the local linear convergence of the inexact ADA for many applications such as the lasso , total variation reconstruction, exchange problem and many other problems from statistics, machine learning and engineering with ℓ _1 regularization.
更多查看译文
关键词
Separable convex minimization, Convergence rate, Augmented decomposition algorithm, Distributed computing
AI 理解论文
溯源树
样例
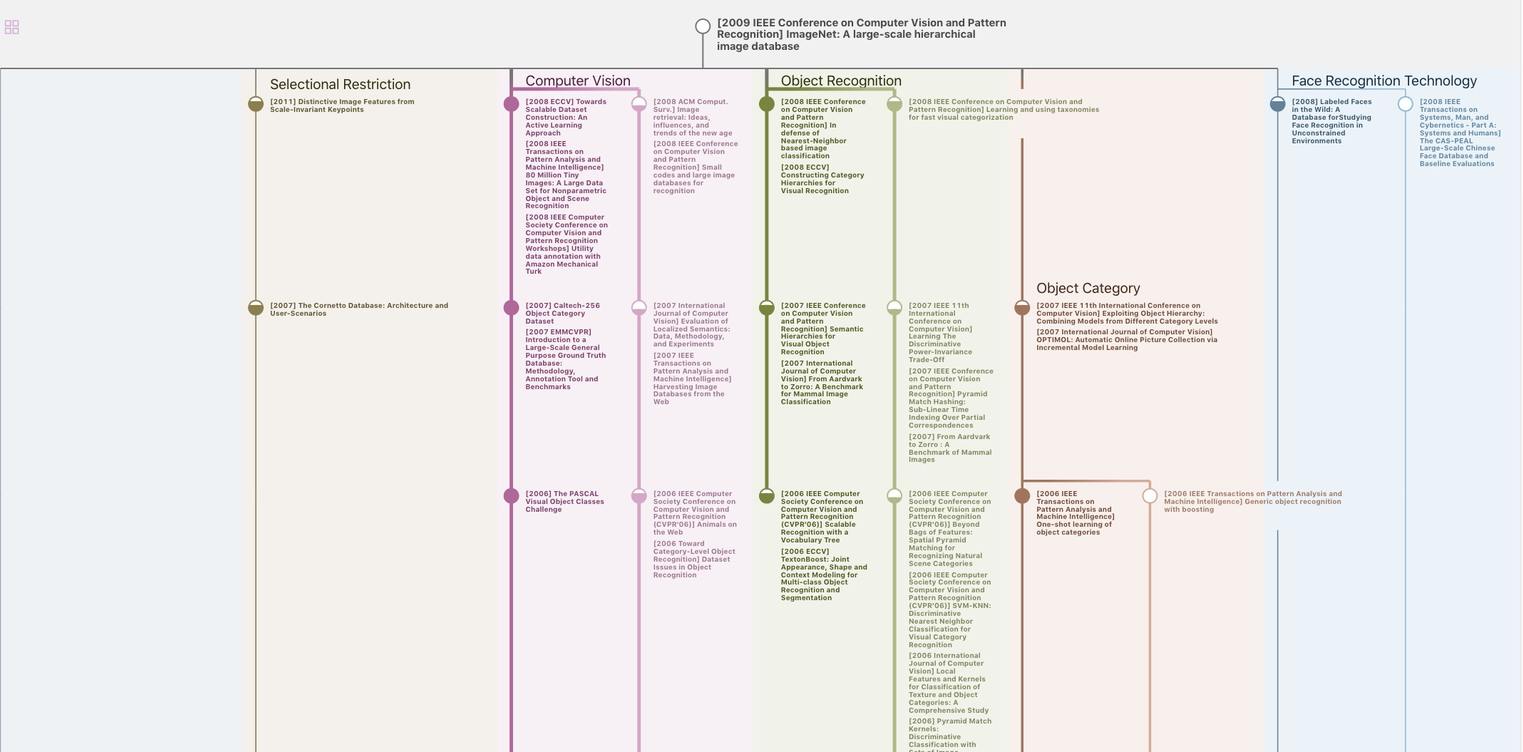
生成溯源树,研究论文发展脉络
Chat Paper
正在生成论文摘要