On the Tradeoff between Mode Collapse and Sample Quality in Generative Adversarial Networks.
IEEE Global Conference on Signal and Information Processing(2018)
摘要
Generative Adversarial Networks (GAN) are used to generate synthetic samples while closely following the underlying distribution of a real data set. While GANs have recently gained significant popularity, they often suffer from the mode collapse problem, where the generated samples lack diversity. Moreover, some approaches that attempt to resolve the model collapse problem do not necessarily yield high quality synthetic samples. In this paper, we propose two novel performance metrics, namely mode-collapse divergence (MCD) which quantifies the extent of mode collapse for a GAN architecture. Second, we propose the metric Generative Quality Score (GQS), which measures the quality of generated samples. We present a comprehensive study of the performance of various GAN architectures proposed in the literature through the lens of MCD and GQS, for three different data sets, namely MNIST, Fashion MNIST and CIFAR-10.
更多查看译文
关键词
Generative Adversarial Networks,Mode Collapse,Performance Metrics
AI 理解论文
溯源树
样例
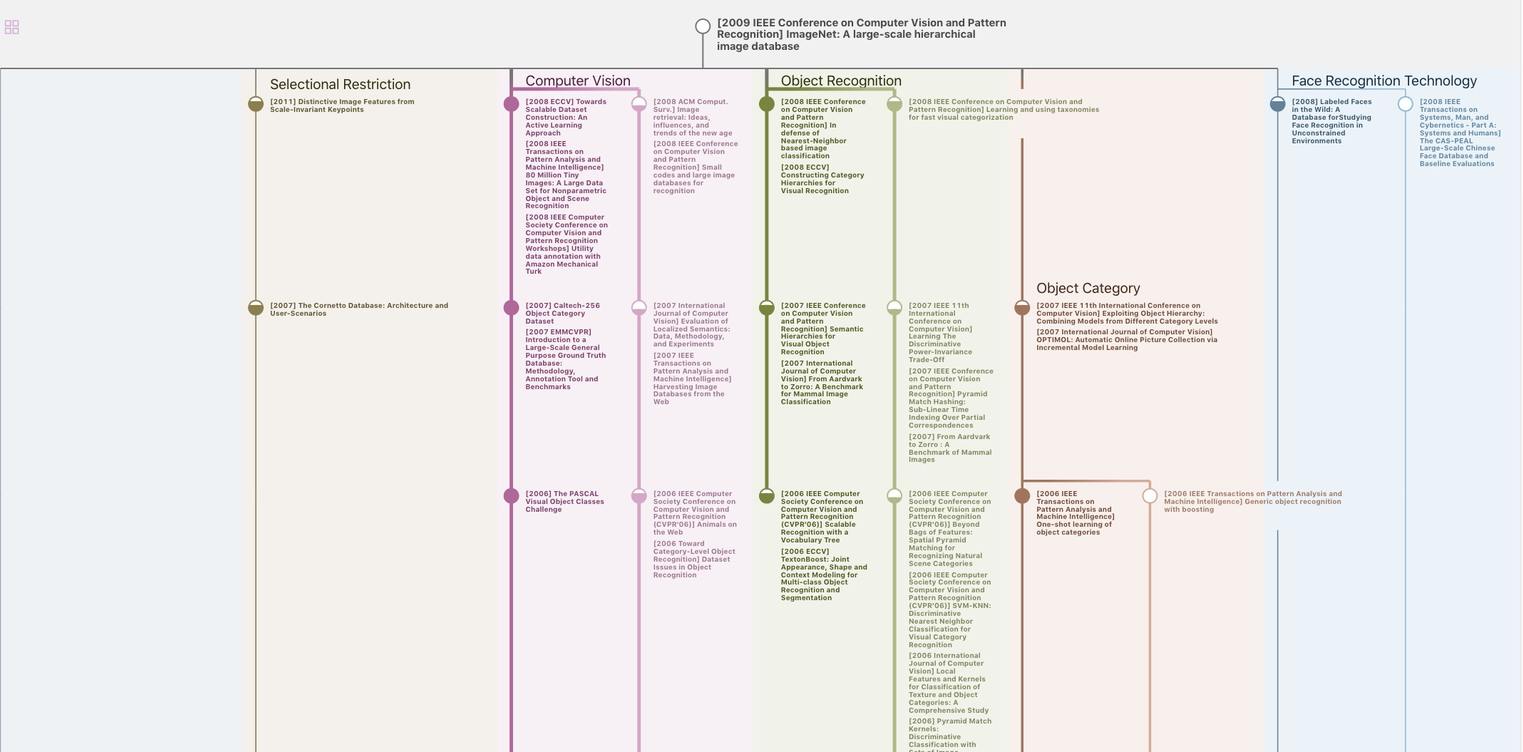
生成溯源树,研究论文发展脉络
Chat Paper
正在生成论文摘要