A data-driven metric learning-based scheme for unsupervised network anomaly detection.
Computers & Electrical Engineering(2019)
摘要
Most network anomaly detection systems (NADSs) rely on the distance between the connections’ feature vectors to identify attacks. Traditional distance metrics are inefficient for these systems as they deal with heterogeneous features of network connections. In this paper, we address a clustering-based NADS employing a data-driven distance metric. This metric is the outcome of a proposed metric learning method, which extracts its required side information from the training samples. The learned transformation matrix maps the connections’ features to a new feature space in which similar and dissimilar connections are more well-separated while the local neighborhood information of the connections’ features is preserved using the Laplacian Eigenmap technique. The proposed NADS is evaluated over the Kyoto 2006+ and NSL-KDD datasets. The experimental results show that it has superior performance in comparison with a recent SVM-clustering based NADS that employs the traditional Euclidean distance function.
更多查看译文
关键词
Network anomaly detection,Metric learning,Linear feature transformation,Clustering methods,Similarity/dissimilarity constraints
AI 理解论文
溯源树
样例
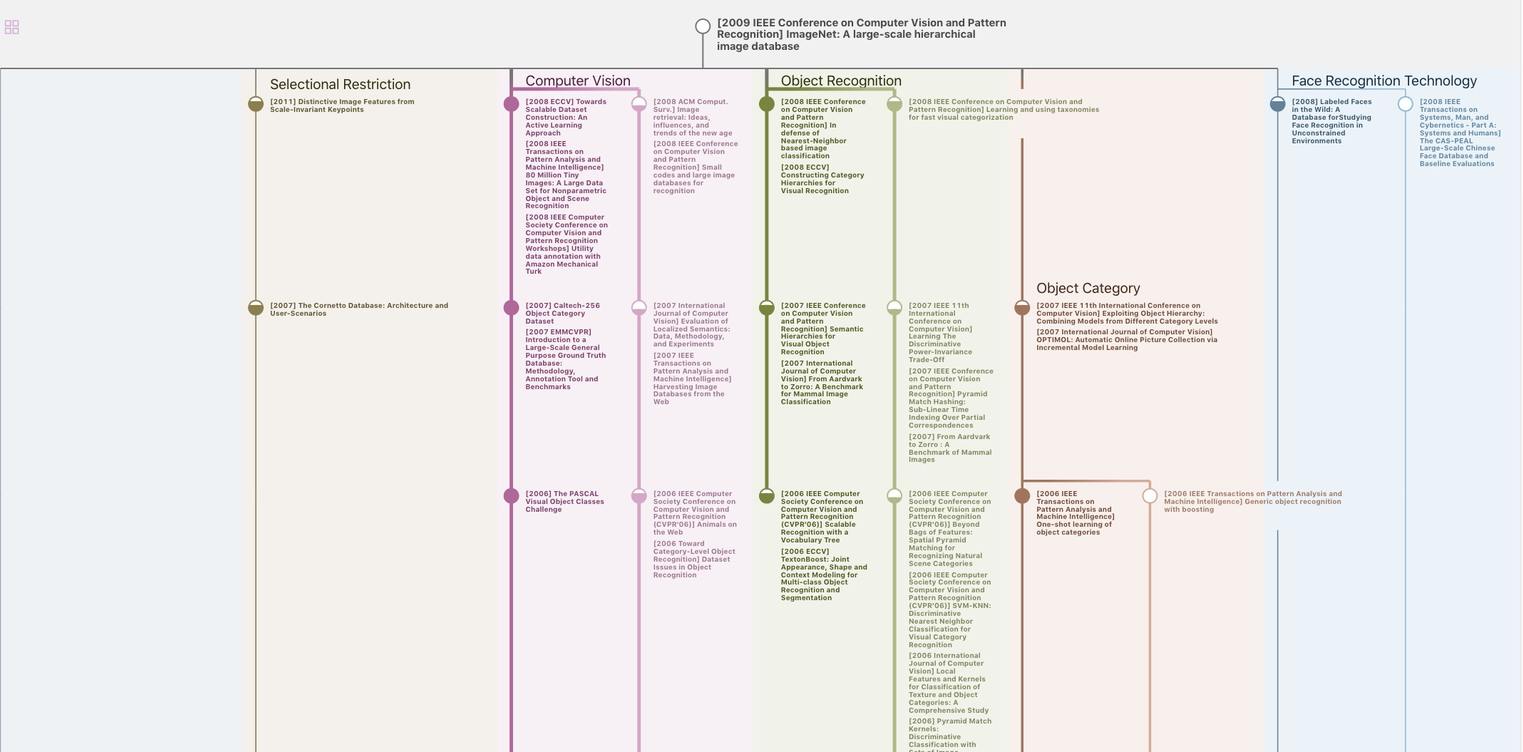
生成溯源树,研究论文发展脉络
Chat Paper
正在生成论文摘要