3D Anisotropic Convolutional Neural Network with Step Transfer Learning for Liver Segmentation
Proceedings of the 4th International Conference on Communication and Information Processing(2018)
Abstract
Automatic liver segmentation on computed tomography (CT) slices plays an important role in current clinical practice for liver cancer supporting diagnosis. While manual segmentation is accurate and precise, it is time-consuming and tedious. Otherwise, automatic segmenting liver from raw CT images suffers from insufficient GPU memory and poor generalization. In this paper, we propose an algorithm to automatically segment liver from CT abdomen images. Frist and foremost, to enlarge receptive field of Convolutional Neural Network (CNN), we apply Atrous layer and Atrous Spatial Pyramid Pooling (ASPP) to our image segmentation network. Furthermore, because it is difficult to train a deep CNN image segmentation network with the is relatively small amount of 3D volumes, we propose step transfer learning technique to boost model performance. Finally, due to the within-slice resolution is much higher than between-slice in CT images, we propose a 3D anisotropic deep CNN network to segment raw CT image from axial, coronal and sagittal axis. Our experiments show that the proposed method achieves Dice scores over 95% on LiST dataset, which is comparable to the state-of-the-art performance. Experimental results demonstrate that the presented model on liver segmentation task is powerful.
MoreTranslated text
Key words
atrous spatial pyramid pooling, convolutional neural network, liver segmentation, step transfer learning
AI Read Science
Must-Reading Tree
Example
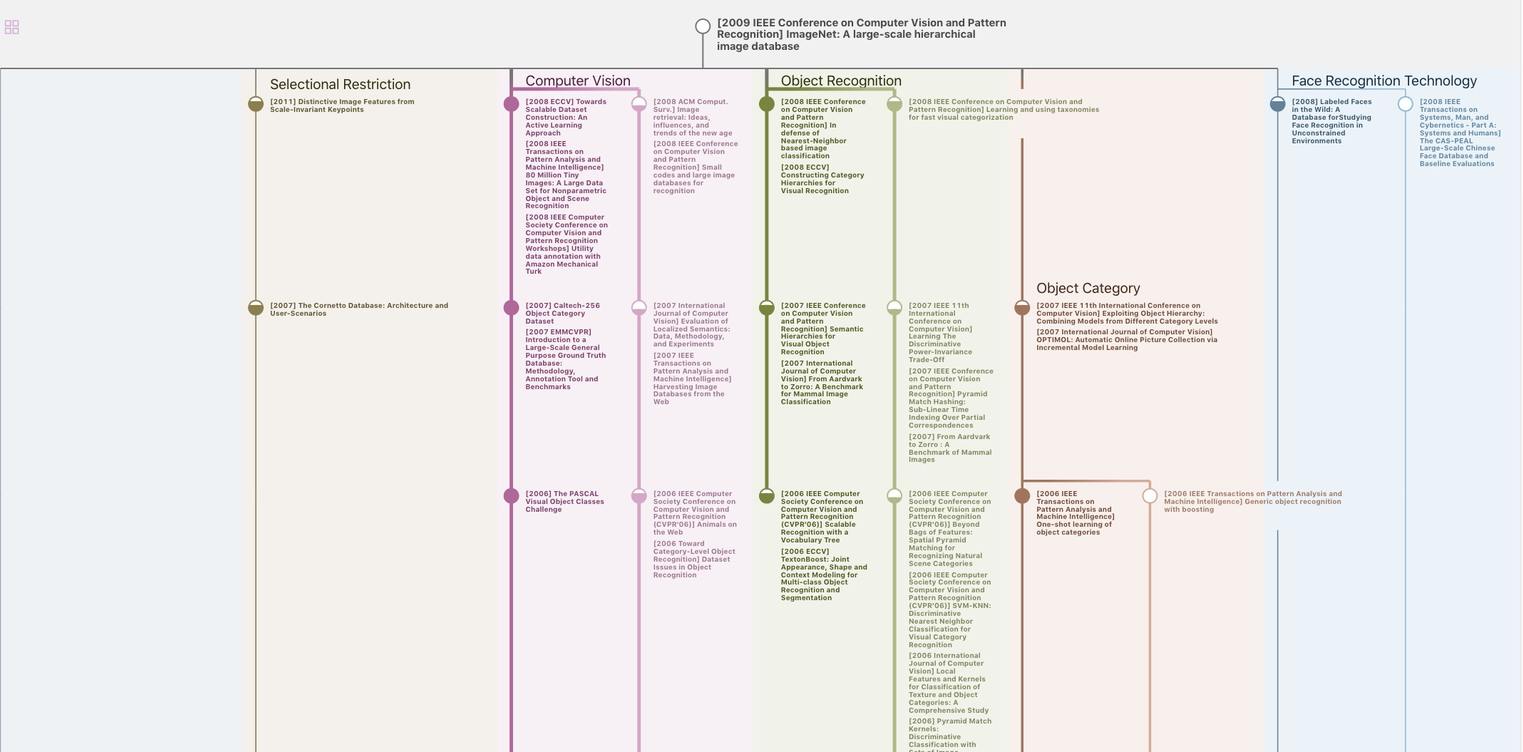
Generate MRT to find the research sequence of this paper
Chat Paper
Summary is being generated by the instructions you defined